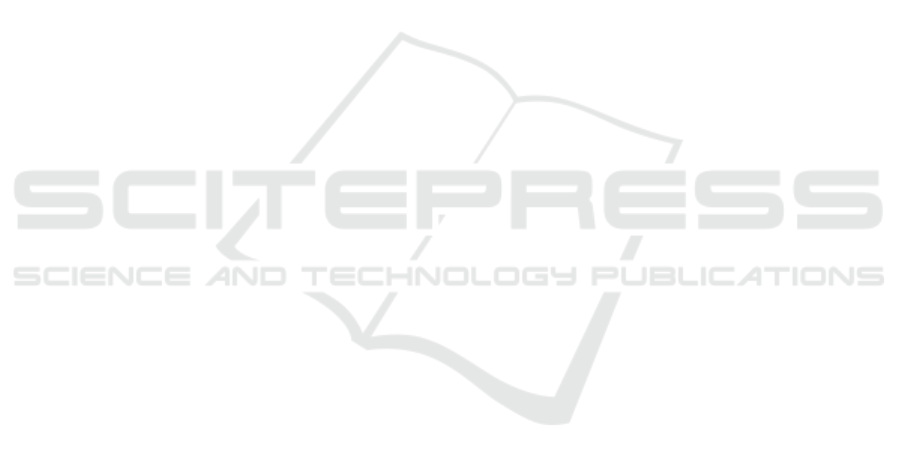
the spread functions L and R are bijective. Moreover
there is no use to compute the inverse of the spread
functions since the real transform is a universal for-
mula independent from L and R.
Further work will explore how to obtain the whole
set of real zeros of (E), not only positive zeros.
The problem when computing with real variables and
fuzzy numbers is intrinsic to fuzzy numbers since the
product by a real scalar is expressed differently de-
pending on the sign of this scalar.
One idea to work around the problem of unknown
sign of x
x
x
d
d
d
is to only focus on positive solutions by
putting back the issue on the fuzzy coefficients. We
solve the system by introducing an artificial k-uplet
of signs I ∈ {−1, 1}
k
, and we replace
e
n
d
d
d
x
x
x
d
d
d
where x
x
x
represents any real by I
d
d
d
e
n
d
d
d
|x
x
x|
d
d
d
which equals
e
n
d
d
d
|x
x
x|
d
d
d
or −
e
n
d
d
d
|x
x
x|
d
d
d
depending on the sign of x
x
x
d
d
d
, where |x
x
x|
is positive. The 2
k
possible k-uplets for I induce the
same number of induced equations E(I). Hence, we
recover the solutions of (E) from the positive solu-
tions of its 2
k
induced equations E(I).
To obtain the real solutions of (E), it will be nec-
essary and sufficient to collect the positive real solu-
tions of the 2
k
real transforms T (E(I)). In practice,
since the equations E(I) are not pairwise distinct, a
strategy will be needed to reduce the number of in-
duced systems T (E(I)) to solve, in order to imple-
ment an optimized algorithm that automatizes the re-
search of solutions by avoiding the studies of signs
needed in previous methods.
ACKNOWLEDGMENT
This work was supported by ANR ARRAND 15-
CE39-0002-01
REFERENCES
Abbasbandy, S. and Amirfakhrian, M. (2006). Numeri-
cal approximation of fuzzy functions by fuzzy poly-
nomials. Applied Mathematics and Computation,
174(2):1001 – 1006.
Abbasbandy, S. and Otadi, M. (2006). Numerical solu-
tion of fuzzy polynomials by fuzzy neural network.
Applied Mathematics and Computation, 181(2):1084–
1089.
Abbasbandy, S., Otadi, M., and Mosleh, M. (2008). Nu-
merical solution of a system of fuzzy polynomi-
als by fuzzy neural network. Information Sciences,
178(8):1948 – 1960.
Ahmad, N., Jacob, K., Mamat, M., and Amir Hamzah, N. S.
(2011). Solving dual fuzzy polynomial equation by
ranking method. Far East Journal of Mathematical
Sciences, 51:151 –163.
Aluja, J., Tacu, A., and Teodorescu, H., editors (1994).
Fuzzy Systems in Economy and Engineering. Publish-
ing House of The Romanian Academy.
Amirfakhrian, M. (2008). Numerical solution of al-
gebraic fuzzy equations with crisp variable by
gauss–newton method. Applied Mathematical Mod-
elling, 32(9):1859 – 1868.
Aubry, P. and Maza, M. (1999). Triangular sets for solving
polynomial systems: a comparative implementation
of four methods. Journal of Symbolic Computation,
28:125–154.
Becker and Weispfenning (1993). Grobner bases..., volume
141 of Graduate texts in math. Springer-Verlag.
Boroujeni, M., Basiri, A., Rahmany, S., and Valibouze, A.
(2016). Finding solutions of fuzzy polynomial equa-
tions systems by an algebraic method. Journal of In-
telligent & Fuzzy Systems, 30(2):791–800.
Buckley, J. and Eslami, E. (1997). Neural net solutions to
fuzzy problems: The quadratic equation. Fuzzy Sets
and Systems, 86(3):289 – 298.
Buckley, J. and Qu, Y. (1990). Solving linear and quadratic
fuzzy equations. Fuzzy Sets and Systems, 38(1):43 –
59.
Buckley, J. J., Feuring, T., and Hayashi, Y. (2002). Solv-
ing fuzzy equations using evolutionary algorithms and
neural nets. Soft Computing, 6(2):116–123.
Dubois, D., Kerre, E., Mesiar, R., and Prade, H. (2000).
Fuzzy Interval Analysis, pages 483–581. Springer US,
Boston, MA.
Dubois, D. and Prade, H. (1978). Operations on fuzzy
numbers. International Journal of systems science,
9(6):613–626.
Farahani, H., Paripour, M., and Abbasbandy, S. (2019).
Resolution of single-variable fuzzy polynomial equa-
tions and an upper bound on the number of solutions.
Soft Computing, pages 1–9.
Farahani, H., Rahmany, S., Basiri, A., and Molai, A. A.
(2015). Resolution of a system of fuzzy polynomial
equations using eigenvalue method. Soft Comput.,
19:283–291.
Goetschel, R. and Voxman, W. (1986). Elementary fuzzy
calculus. Fuzzy Sets Syst., 18(1):31–43.
Kajani, M. T., Asady, B., and Vencheh, A. H. (2005).
An iterative method for solving dual fuzzy nonlinear
equations. Applied Mathematics and Computation,
167(1):316 – 323.
Liu, P. (2002). Analysis of approximation of continuous
fuzzy functions by multivariate fuzzy polynomials.
Fuzzy Sets and Systems, 127(3):299–313.
Lodwick, W. (2007). Fuzzy Surfaces in GIS and Geographi-
cal Analysis: Theory, Analytical Methods, Algorithms
and Applications. CRC Press.
Lodwick, W. A. and Santos, J. (2003). Constructing con-
sistent fuzzy surfaces from fuzzy data. Fuzzy Sets and
Systems, 135(2):259 – 277.
Molai, A. A., Basiri, A., and Rahmany, S. (2013). Reso-
lution of a system of fuzzy polynomial equations us-
FCTA 2019 - 11th International Conference on Fuzzy Computation Theory and Applications
358