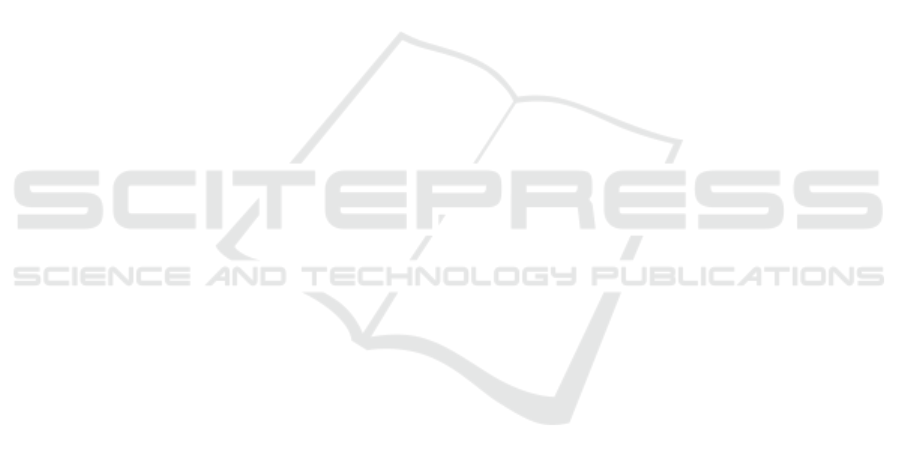
tion scheme adapts to changes in the model. The best
result 137.1s (10 times with forecasting) ) is 55.5%
faster than the one obtained with the basic scenario.
6 CONCLUSION
In this work, a symbiotic four-level scheme of the
organization of the computational process for multi-
agent simulation was proposed. The key feature of
interaction between the model and the system is con-
centrated in the virtual modeling environment, which
includes the application agents’ activities and opti-
mization results, formed by the planning and control
module. This module implements an algorithm with
the genetic optimization core and intelligent agents
for workload prediction. The obtained results show
the high efficiency of the proposed approach, as they
not only complete the set of test scenarios faster but
also increase the potential ability for scaling, by se-
lecting the right division areas of the space and reduc-
ing overhead costs. The results of experiments show
the efficiency of proposed execution scheme and GA
by the improvement of the modelling time in compar-
ison to the default schedule by 55%.
ACKNOWLEDGEMENTS
This research is financially supported by The Russian
Foundation for Basic Research, Agreement #18-37-
00416.
REFERENCES
(2019). Lobachevskiy supercomputer. http://www.itmm.
unn.ru/ob-institute/oborudovanie/. Accessed: 2019-
06-10.
(2019). Lomonosov supercomputer. https://parallel.ru/
cluster/about. Accessed: 2019-06-10.
Alowayyed, S., Piontek, T., Suter, J. L., Hoenen, O., Groen,
D., Luk, O., Bosak, B., Kopta, P., Kurowski, K.,
Perks, O., et al. (2019). Patterns for high performance
multiscale computing. Future Generation Computer
Systems, 91:335–346.
Andreadis, G., Versluis, L., Mastenbroek, F., and Iosup,
A. (2018). A reference architecture for datacenter
scheduling: design, validation, and experiments. In
Proceedings of the International Conference for High
Performance Computing, Networking, Storage, and
Analysis, page 37. IEEE Press.
Kremer-Herman, N., Tovar, B., and Thain, D. (2018). A
lightweight model for right-sizing master-worker ap-
plications. In SC18: International Conference for
High Performance Computing, Networking, Storage
and Analysis, pages 504–516. IEEE.
Liu, F., Keahey, K., Riteau, P., and Weissman, J.
(2018). Dynamically negotiating capacity between
on-demand and batch clusters. In Proceedings of the
International Conference for High Performance Com-
puting, Networking, Storage, and Analysis, page 38.
IEEE Press.
Malakar, P., Munson, T., Knight, C., Vishwanath, V., and
Papka, M. E. (2018). Topology-aware space-shared
co-analysis of large-scale molecular dynamics simu-
lations. In SC18: International Conference for High
Performance Computing, Networking, Storage and
Analysis, pages 305–319. IEEE.
Martinasso, M., Gila, M., Bianco, M., Alam, S. R., McMur-
trie, C., and Schulthess, T. C. (2018). Rm-replay: a
high-fidelity tuning, optimization and exploration tool
for resource management. In Proceedings of the In-
ternational Conference for High Performance Com-
puting, Networking, Storage, and Analysis, page 25.
IEEE Press.
Nasonov, D., Butakov, N., Melnik, M., Visheratin, A.,
Linev, A., Shvets, P., Sobolev, S., and Mukhina, K.
(2018). The multi-level adaptive approach for efficient
execution of multi-scale distributed applications with
dynamic workload. In Russian Supercomputing Days,
pages 675–686. Springer.
Pollard, S. D., Jain, N., Herbein, S., and Bhatele, A. (2018).
Evaluation of an interference-free node allocation pol-
icy on fat-tree clusters. In Proceedings of the Interna-
tional Conference for High Performance Computing,
Networking, Storage, and Analysis, page 26. IEEE
Press.
Smith, S. A., Cromey, C. E., Lowenthal, D. K., Domke, J.,
Jain, N., Thiagarajan, J. J., and Bhatele, A. (2018).
Mitigating inter-job interference using adaptive flow-
aware routing. In Proceedings of the International
Conference for High Performance Computing, Net-
working, Storage, and Analysis, page 27. IEEE Press.
Subedi, P., Davis, P., Duan, S., Klasky, S., Kolla, H.,
and Parashar, M. (2018). Stacker: an autonomic
data movement engine for extreme-scale data staging-
based in-situ workflows. In Proceedings of the In-
ternational Conference for High Performance Com-
puting, Networking, Storage, and Analysis, page 73.
IEEE Press.
Zimmer, C., Maxwell, D., McNally, S., Atchley, S., and
Vazhkudai, S. S. (2018). Gpu age-aware scheduling
to improve the reliability of leadership jobs on titan.
In Proceedings of the International Conference for
High Performance Computing, Networking, Storage,
and Analysis, page 7. IEEE Press.
Zitzlsberger, G., Jans
´
ık, B., and Martinovi
ˇ
c, J. (2018). Fea-
sibility analysis of using the maui scheduler for job
simulation of large-scale pbs based clusters. IADIS
International Journal on Computer Science & Infor-
mation Systems, 13(2).
Intellectual Execution Scheme of Iterative Computational Models based on Symbiotic Interaction with Application for Urban Mobility
Modelling
251