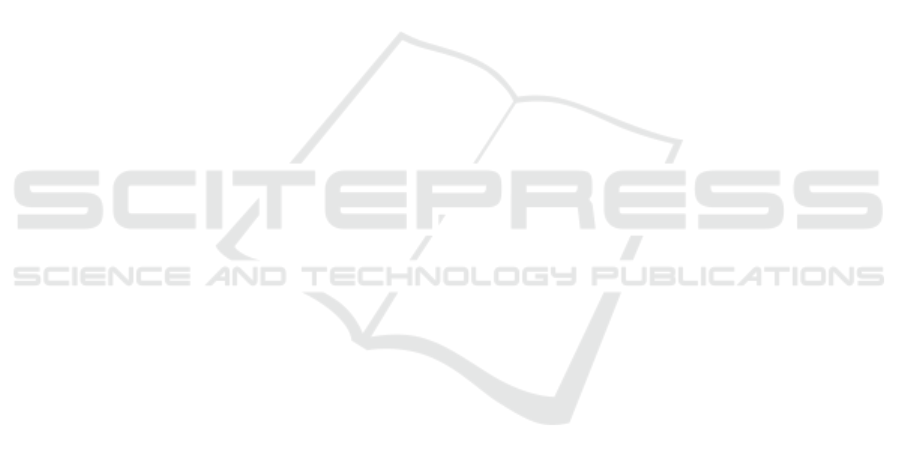
Brown, I. and Mues, C. (2012). An experimental compari-
son of classification algorithms for imbalanced credit
scoring data sets. Expert Systems with Applications,
39(3):3446–3453.
Castiello, C., Castellano, G., and Fanelli, A. M. (2005).
Meta-data: Characterization of input features for
meta-learning. In International Conference on Mod-
eling Decisions for Artificial Intelligence, pages 457–
468. Springer.
Chai, T. and Draxler, R. R. (2014). Root mean square er-
ror (rmse) or mean absolute error (mae)?–arguments
against avoiding rmse in the literature. Geoscientific
model development, 7(3):1247–1250.
Chawla, N. V., Japkowicz, N., and Kotcz, A. (2004). Special
issue on learning from imbalanced data sets. ACM
Sigkdd Explorations Newsletter, 6(1):1–6.
Chen, N., Ribeiro, B., and Chen, A. (2016). Financial credit
risk assessment: a recent review. Artificial Intelli-
gence Review, 45(1):1–23.
Chopra, A. and Bhilare, P. (2018). Application of ensemble
models in credit scoring models. Business Perspec-
tives and Research, 6(2):129–141.
Crook, J. N., Edelman, D. B., and Thomas, L. C. (2007).
Recent developments in consumer credit risk assess-
ment. European Journal of Operational Research,
183(3):1447–1465.
Dal Pozzolo, A., Caelen, O., Le Borgne, Y.-A., Wa-
terschoot, S., and Bontempi, G. (2014). Learned
lessons in credit card fraud detection from a practi-
tioner perspective. Expert systems with applications,
41(10):4915–4928.
Damrongsakmethee, T. and Neagoe, V.-E. (2019). Principal
component analysis and relieff cascaded with decision
tree for credit scoring. In Computer Science On-line
Conference, pages 85–95. Springer.
Doumpos, M., Lemonakis, C., Niklis, D., and Zopounidis,
C. (2019). Credit scoring and rating. In Analytical
Techniques in the Assessment of Credit Risk, pages
23–41. Springer.
Garc
´
ıa, S., Ram
´
ırez-Gallego, S., Luengo, J., Ben
´
ıtez, J. M.,
and Herrera, F. (2016). Big data preprocessing: meth-
ods and prospects. Big Data Analytics, 1(1):9.
Ghodselahi, A. (2011). A hybrid support vector machine
ensemble model for credit scoring. International
Journal of Computer Applications, 17(5):1–5.
Giraud-Carrier, C., Vilalta, R., and Brazdil, P. (2004). Intro-
duction to the special issue on meta-learning. Machine
learning, 54(3):187–193.
Haixiang, G., Yijing, L., Shang, J., Mingyun, G., Yuanyue,
H., and Bing, G. (2017). Learning from class-
imbalanced data: Review of methods and applica-
tions. Expert Systems with Applications, 73:220–239.
Harris, T. (2015). Credit scoring using the clustered sup-
port vector machine. Expert Systems with Applica-
tions, 42(2):741–750.
Hassan, M. K., Brodmann, J., Rayfield, B., and Huda, M.
(2018). Modeling credit risk in credit unions using
survival analysis. International Journal of Bank Mar-
keting, 36(3):482–495.
Hawkins, D. M. (2004). The problem of overfitting. Jour-
nal of chemical information and computer sciences,
44(1):1–12.
Henrique, B. M., Sobreiro, V. A., and Kimura, H. (2019).
Literature review: Machine learning techniques ap-
plied to financial market prediction. Expert Systems
with Applications.
Huang, J. and Ling, C. X. (2005). Using auc and accuracy
in evaluating learning algorithms. IEEE Transactions
on knowledge and Data Engineering, 17(3):299–310.
Jappelli, T., Pagano, M., et al. (2000). Information sharing
in credit markets: a survey. Technical report, CSEF
working paper.
Jeni, L. A., Cohn, J. F., and De La Torre, F. (2013). Fac-
ing imbalanced data–recommendations for the use of
performance metrics. In 2013 Humaine Association
Conference on Affective Computing and Intelligent In-
teraction, pages 245–251. IEEE.
Khemais, Z., Nesrine, D., Mohamed, M., et al. (2016).
Credit scoring and default risk prediction: A compar-
ative study between discriminant analysis & logistic
regression. International Journal of Economics and
Finance, 8(4):39.
Khemakhem, S., Ben Said, F., and Boujelbene, Y. (2018).
Credit risk assessment for unbalanced datasets based
on data mining, artificial neural network and support
vector machines. Journal of Modelling in Manage-
ment, 13(4):932–951.
Lessmann, S., Baesens, B., Seow, H., and Thomas, L. C.
(2015a). Benchmarking state-of-the-art classifica-
tion algorithms for credit scoring: An update of re-
search. European Journal of Operational Research,
247(1):124–136.
Lessmann, S., Baesens, B., Seow, H.-V., and Thomas,
L. C. (2015b). Benchmarking state-of-the-art classi-
fication algorithms for credit scoring: An update of
research. European Journal of Operational Research,
247(1):124–136.
Li, Q., Wu, Q., Zhu, C., Zhang, J., and Zhao, W. (2019).
Unsupervised user behavior representation for fraud
review detection with cold-start problem. In Pacific-
Asia Conference on Knowledge Discovery and Data
Mining, pages 222–236. Springer.
Liu, C., Huang, H., and Lu, S. (2019). Research on personal
credit scoring model based on artificial intelligence. In
International Conference on Application of Intelligent
Systems in Multi-modal Information Analytics, pages
466–473. Springer.
Liu, H., Hussain, F., Tan, C. L., and Dash, M. (2002). Dis-
cretization: An enabling technique. Data mining and
knowledge discovery, 6(4):393–423.
Livshits, I. (2015). Recent developments in consumer credit
and default literature. Journal of Economic Surveys,
29(4):594–613.
Luo, C., Wu, D., and Wu, D. (2017). A deep learn-
ing approach for credit scoring using credit default
swaps. Engineering Applications of Artificial Intel-
ligence, 65:465–470.
Luque, A., Carrasco, A., Mart
´
ın, A., and de las Heras, A.
(2019). The impact of class imbalance in classifica-
KDIR 2019 - 11th International Conference on Knowledge Discovery and Information Retrieval
212