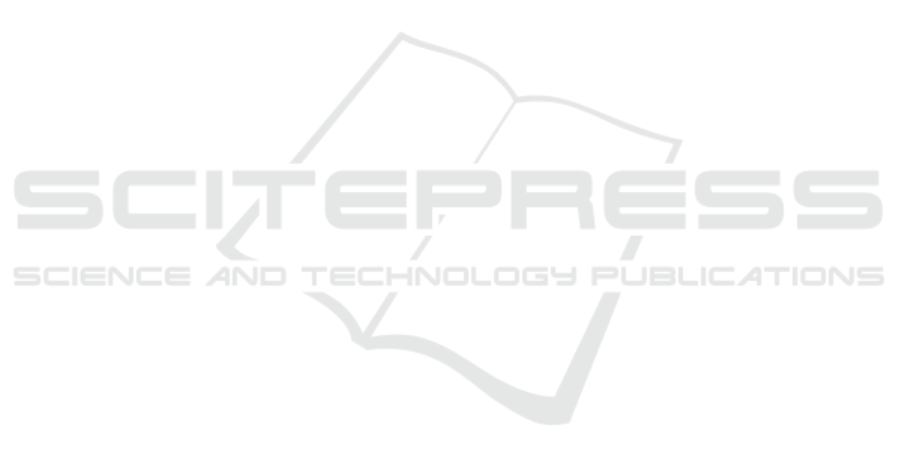
ings of the 2007 SIAM international conference on
data mining, pages 443–448. SIAM.
Borchani, H., Mart
´
ınez, A. M., Masegosa, A. R., Langseth,
H., Nielsen, T. D., Salmer
´
on, A., Fern
´
andez, A., Mad-
sen, A. L., and S
´
aez, R. (2015). Modeling concept
drift: A probabilistic graphical model based approach.
In International Symposium on Intelligent Data Anal-
ysis, pages 72–83. Springer.
Caba
˜
nas, R., Cano, A., G
´
omez-Olmedo, M., Masegosa,
A. R., and Moral, S. (2018). Virtual subconcept drift
detection in discrete data using probabilistic graphi-
cal models. In International Conference on Informa-
tion Processing and Management of Uncertainty in
Knowledge-Based Systems, pages 616–628. Springer.
Gama, J., Fernandes, R., and Rocha, R. (2006). Decision
trees for mining data streams. Intelligent Data Analy-
sis, 10(1):23–45.
Gama, J., Medas, P., Castillo, G., and Rodrigues, P. (2004).
Learning with drift detection. In Brazilian symposium
on artificial intelligence, pages 286–295. Springer.
Gama, J.,
ˇ
Zliobait
˙
e, I., Bifet, A., Pechenizkiy, M., and
Bouchachia, A. (2014). A survey on concept
drift adaptation. ACM computing surveys (CSUR),
46(4):44.
Harries, M. B., Sammut, C., and Horn, K. (1998). Extract-
ing hidden context. Machine learning, 32(2):101–
126.
Huang, D. T. J., Koh, Y. S., Dobbie, G., and Pears, R.
(2014). Detecting volatility shift in data streams. In
2014 IEEE International Conference on Data Mining,
pages 863–868. IEEE.
Iwashita, A. S. and Papa, J. P. (2019). An overview on con-
cept drift learning. IEEE Access, 7:1532–1547.
Kelly, M. G., Hand, D. J., and Adams, N. M. (1999). The
impact of changing populations on classifier perfor-
mance. In Proceedings of the fifth ACM SIGKDD in-
ternational conference on Knowledge discovery and
data mining, pages 367–371. Citeseer.
Kifer, D., Ben-David, S., and Gehrke, J. (2004). Detecting
change in data streams. In Proceedings of the Thirti-
eth international conference on Very large data bases-
Volume 30, pages 180–191. VLDB Endowment.
Lauritzen, S. L. and Spiegelhalter, D. J. (1988). Local
computations with probabilities on graphical struc-
tures and their application to expert systems. Journal
of the Royal Statistical Society. Series B (Methodolog-
ical), pages 157–224.
Lynch, S. M. (2007). Introduction to applied Bayesian
statistics and estimation for social scientists. Springer
Science & Business Media.
Madsen, A. L., Jensen, F., Kjaerulff, U. B., and Lang, M.
(2005). The hugin tool for probabilistic graphical
models. International Journal on Artificial Intelli-
gence Tools, 14(03):507–543.
Moreno-Torres, J. G., Raeder, T., Alaiz-Rodr
´
ıGuez, R.,
Chawla, N. V., and Herrera, F. (2012). A unifying
view on dataset shift in classification. Pattern Recog-
nition, 45(1):521–530.
Neapolitan, R. E. et al. (2004). Learning bayesian networks,
volume 38. Pearson Prentice Hall Upper Saddle River,
NJ.
Olesen, K. G., Lauritzen, S. L., and Jensen, F. V. (1992).
ahugin: A system creating adaptive causal probabilis-
tic networks. In Uncertainty in Artificial Intelligence,
1992, pages 223–229. Elsevier.
Page, E. S. (1954). Continuous inspection schemes.
Biometrika, 41(1/2):100–115.
Pearl, J. (2014). Probabilistic reasoning in intelligent sys-
tems: networks of plausible inference. Elsevier.
Raiffa, H. and Schlaifer, R. (1961). Applied statistical de-
cision theory. Div. of Research, Graduate School of
Business Administration, Harvard Univ.
Ross, G. J., Adams, N. M., Tasoulis, D. K., and Hand,
D. J. (2012). Exponentially weighted moving average
charts for detecting concept drift. Pattern recognition
letters, 33(2):191–198.
Sebasti
˜
ao, R., Gama, J., and Mendonc¸a, T. (2017). Fad-
ing histograms in detecting distribution and concept
changes. International Journal of Data Science and
Analytics, 3(3):183–212.
Shannon, C. E. (2001). A mathematical theory of commu-
nication. ACM SIGMOBILE mobile computing and
communications review, 5(1):3–55.
Tsymbal, A. (2004). The problem of concept drift: defi-
nitions and related work. Computer Science Depart-
ment, Trinity College Dublin, 106(2):58.
Wang, S., Schlobach, S., and Klein, M. (2010). What is
concept drift and how to measure it? In International
Conference on Knowledge Engineering and Knowl-
edge Management, pages 241–256. Springer.
Webb, G. I., Hyde, R., Cao, H., Nguyen, H. L., and Petit-
jean, F. (2016). Characterizing concept drift. Data
Mining and Knowledge Discovery, 30(4):964–994.
Widmer, G. and Kubat, M. (1996). Learning in the pres-
ence of concept drift and hidden contexts. Machine
learning, 23(1):69–101.
ˇ
Zliobait
˙
e, I., Pechenizkiy, M., and Gama, J. (2016). An
overview of concept drift applications. In Big data
analysis: new algorithms for a new society, pages 91–
114. Springer.
KDIR 2019 - 11th International Conference on Knowledge Discovery and Information Retrieval
224