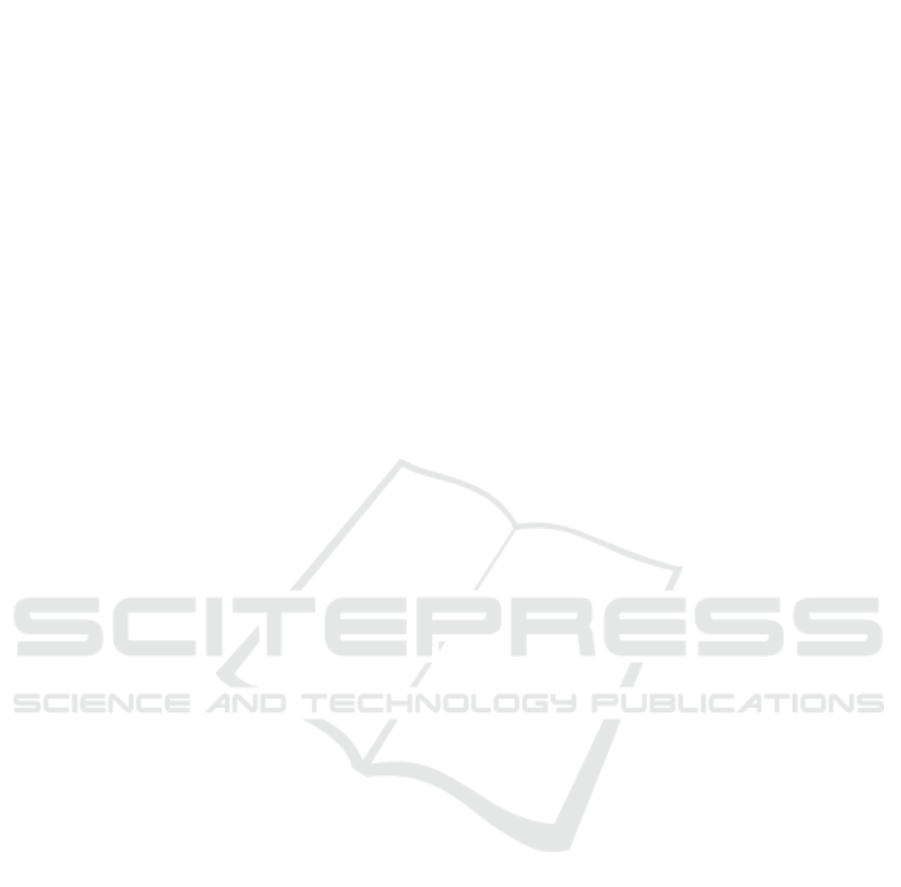
Dunlosky, Marsh, Nathan, & Willingham, 2013)
clickstream analysis (Nasraoui, Cardona, Rojas &
Gonzalez, 2003), effectiveness of online learning or
Massive open online course (MOOC, 2019;Kaplan &
Haenlein, 2016; Balakrishnan, 2013; Hew, 2016),
content management (CMS, 2018), and high level
cognition and learning models (Heer, 2010;
Kirkpatrick, 2019).
2 DESIGN MEASURES OF
EFFECTIVENESS (MoEs) AND
DATA COLLECTION
We see the potential for the MarineNet platform to
deliver personalized learning including micro-
learning--targeted learning either for certain groups, a
specific student, or perhaps identified learning styles
of the student. The technology can support
personalized learning through Experience API (xAPI,
2019)— an e-learning software specification that
allows learning content and learning systems to speak
to each other in a manner that records and tracks all
types of learning experiences. However, the
technology isn’t the full solution because content
must support learning outcomes/objectives and
learning must be assessed to measure and improve the
learning process. This is accomplished first by
designing valid measures of effectiveness (MoEs). To
answer the research questions, we studied current
learning theories and, using existing and available
MarineNet data, designed and selected MoEs to
support those constructs based on accepted
pedagogical theory and practice as well as on our
exploration and evaluation of various deep analytics
models.
Initial research identified 36 MoEs, among them,
content profiles, student profiles, and student learning
behavior are the most important categories as follows:
1 Content Alignment Data.
At the enterprise level, measures of program
effectiveness (to a lesser extent learning) are typically
tracked by completion rate, GPA, and stated course
outcomes or objectives. A CMS must capture this
information first. One consideration is that course
content must align with the course objectives which
could greatly impact the course outcomes (e.g.
completion rate and GPA) and provide students
suitable learning experiences, appropriate
assessment, and measurable progress.
For example, a MoE in this category can compute
the correlation of a course content with its predefined
objectives. However, this may require text analysis
which is out of the initial scope of this project. As an
alternative, an instructor could tag the various content
such as pre-tests, progress tests, and post-tests with
the corresponding learning objectives so that the test
scores, GPA, and complete rates can accurately be
measured if the learning objectives are achieved. If
the instructor is trained to develop
objectives/outcomes for higher levels of learning for
example, critical thinking can be measured using
content tags as well.
2 Student Profile Data.
Measures for learning are often unique to disciplinary
fields or individual cohorts or communities.
Measuring learning must consider the level of
knowledge the student has before the course as
compared to after the course, the delta. This delta
would then measure the transference of new
knowledge not what the student already knows. These
MoEs can be supported by a pre-course survey to
collect demographic, biographical information.
Motivation reflected in the information can
significantly enhance retention and transfer and can
be the unique differences among individual learners.
3 Student Learning Result Data.
A MoE in this category can be the degree to which
targeted outcomes occur as a result of the training, for
example, grades, rubric, rewards, GPA, and the
number of attempts for competency-based quizzes—
i.e. required score of 80%? For example, MarineNet
Distance Education Programs and training courses
typically use 80% to demonstrate the required
knowledge master level.
4 Student Learning Behavioral Data.
Through the MarineNet website, a student can
interact with content, instructors, and peers. These
interactions can be recorded as student learning
behavior data.
The average time and frequency that students
access different content are the important measures of
student learning behavior. A MoE in this category can
be the frequency (or clickstream patterns) for each
type of content accessed.
Since an instructor could tag that content (or not)
that he or she deems that repetitive learning would be
beneficial. MoEs in this category could be aggregated
counts or percentages if tagged by objectives or by
type of content.
Interaction with instructors with guided practice
and timely, formative feedback improves learning
and performance. A MoE in this category can be the
frequency (or clickstream patterns) for such
interactions: the number of times (counts)
instructors/students communicate through email
Measures of Effectiveness (MoEs) for MarineNet: A Case Study for a Smart e-Learning Organization
147