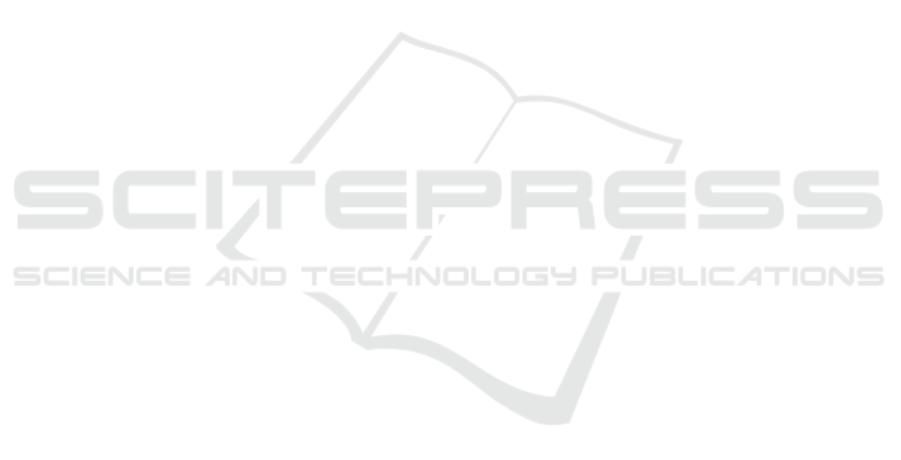
Using this alternative view on computation allows
a more direct comparison to the processing of infor-
mation in neurobiological systems. From an evo-
lutionary perspective neurobiological systems devel-
oped to solve specific computational problems, e.g.,
to control muscles or to process the information com-
ing from a sensory system. As such, the respec-
tive networks of neurons or groups of neurons could
be seen as specialised transformation networks sim-
ilar to the transformation networks described above.
However, instead of being emulated and virtual, these
natural transformation networks are actually imple-
mented within the neurobiological substrate removing
the necessity for memory as a “transformation glue”
while at the same time adding a number of constraints
regarding possible connection patterns and types of
transformations. In particular, an on-the-fly reconfig-
uration and expansion of the neurobiological transfor-
mation network is not possible in general. Instead, the
basic structure of the network emerges during the de-
velopment of the organism and is controlled by, e.g.,
periods of cell proliferation, cell migration along cel-
lular support structures, or guidance by short and long
range chemical gradients (Squire et al., 2008). The
variability inherent in this self-organized formation
limits the extent with which the solution to a specific
computational problem, e.g., a behavioral pattern, can
be hardwired into the network structure and adapted
over time. To mitigate this constraint neurobiologi-
cal networks show forms of plasticity that facilitate
fast changes to the network by adapting the response
of individual neurons or local groups of neurons to a
given input signal. As a consequence, the correspond-
ing input output transformation implemented by those
neurons changes accordingly. However, subsequent
transformations are not informed about this change
but have to adapt themselves as well if necessary and
propagate the change further. This means that from a
global perspective the way by which signals are en-
coded and processed within the transformation net-
work is only known locally and remains in a constant
flux.
This dynamic change of how information is en-
coded and processed by different parts of the trans-
formation network conflicts with an idea of memory
that sees memory as a container that stores patterns
of information for later use since the encoding of that
information might have changed while it was stored.
Such an outdated encoding would then become unin-
telligible for the network. Similarly, the idea of mem-
ory as a means to coordinate and control the flow of
information relies as well on a consistent encoding,
which is not guaranteed when local neuroplasticity is
taken into account. Despite these doubts regarding
the biological plausibility of a container-like memory
one might argue that neurobiological systems clearly
do have the ability to remember, e.g., past experiences
and therefore must have some form of memory. To
address this point we will outline our view on a neu-
robiologically plausible memory in the next section.
4 MEMORY AS A PROCESS
In the previous section we described how local neuro-
plasticity continually changes the way how neurons
or local groups of neurons respond to their inputs
and thus encode these inputs differently for neurons
downstream in the network. A memory system that is
based on storing and recreating patterns of informa-
tion is not well suited to cope with this drift of encod-
ing. We therefore suggest that memory in a neurobio-
logical network does not store and recreate the signals
that pass through the network but is rather a mecha-
nism that allows to store and reestablish the activation
state of the network or parts thereof, i.e., to not recre-
ate the result of some computation but to reestablish
the conditions that led to the result.
We argue that such a type of memory has to be a
distributed, intrinsic part of the network instead of be-
ing a dedicated, localized memory system. Moreover,
we see local neuroplasticity, the characteristic respon-
sible for the drift of encoding, as a key mechanism for
this memory. It enables individual neurons to learn
typical input patterns that capture some information
about the statistical nature of their inputs (Kerdels and
Peters, 2018). If such a neuron n
a
receives its inputs
from sensory cells, then the neuron learns something
about the statistical nature of that part of the world
that is transduced by these cells. If the neuron n
a
receives its inputs from multiple other neurons n
i
, it
learns something about the statistical nature of the co-
activation of these input neurons. It learns or forms
an association between these cells, i.e., it becomes a
small associative memory. However, at this stage it
is more of an association detector or something akin
to a hash function. The cell could answer the ques-
tion “Have I seen this activation pattern before?”, but
it can not be “read out” to reestablish that activation
pattern. A solution to this problem arises when one
assumes that the neurons n
i
are capable of forming as-
sociative memories themselves. In that case, the orig-
inal associative memory neuron n
a
would just have
to form reciprocal feedback connections to all its in-
puts. Reading out this cell, i.e., activating it, would
then result in reestablishing the activation state of the
input neurons n
i
. Although this reciprocal connec-
tion appears rather simple it can exhibit a range of
Challenging the Intuition about Memory and Computation in Theories of Cognition
525