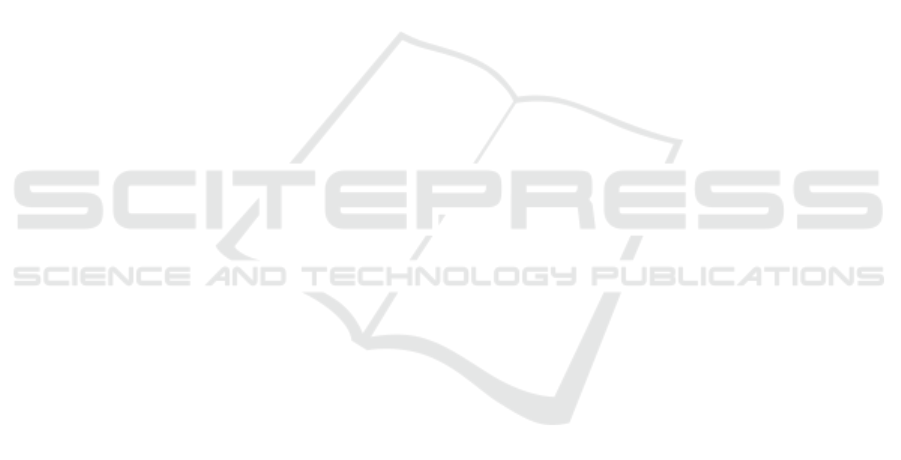
Chong, S. C. & Choi, Y. S. 2005. Critical Factors in the
Successful Implementation of Knowledge Management,
Journal of Knowledge Management Practice, 6.
Chen, W., Elnaghi, M. & Hatzakis, T. 2011. Investigating
knowledge management factors affecting Chinese ICT
firms performance: An integrated KM framework.
Information Systems Management, 28(1), 19-29.
Chin, W. W. 1998. The partial least squares approach to
structural equation modeling, Lawrence Erlbaum
Associates, Mahwah, New Jersey
Cohen J. 1988. Statistical Power Analysis for the Behavioral
Sciences. Mahwah, NJ: Lawrence Erlbaum
Cong, X., Li-Hua, R. and Stonehouse G. 2007. Knowledge
management in the Chinese public sector: empirical
investigation, Journal of Technology Management in
China, 2(3), 250-263
Donate, M. J. & Pablo, J. D. S. 2015. The role of knowledge-
oriented leadership in knowledge management practices
and innovation, Journal of Business Research, 68(2),
360-370
Evoy, P. J. M., Mohamed, A.F.R. & Arisha, A. 2019. The
effectiveness of knowledge management in the public
sector, Knowledge Management Research & Practice,
17(1), 39-51.
Fornell, C. & Larker, D. F. 1981. Evaluating Structural
Equation Models with Unobservable Variables and
Measurement Error. Journal of Marketing Research,
18(1), 39-50.
Goel, A. K., Sharma, G. R. & Rastogi, R. (2010), Knowledge
Management implementation in NTPC: an Indian PSU,
Management Decision, 48(3), 383-395.
Gold, A.H., Malhotra, A., Segars, A.H. 2001. Knowledge
Management: an organizational capabilities perspective,
Journal of Management Information Systems,18(1), 185-
214.
Hair, J. F., Sarstedt, M., Hopkins, L., & Kuppelwieser, V. G.
2014. Partial least squares structural equation modeling
(PLS-SEM). European Business Review, 26, 106–121.
Hiebler, R. 1996. Benchmarking Knowledge Management,
Strategy and Leadership, 24(2), 22-29.
Lee, H. and Choi., B. 2003. Knowledge Management
enablers, processes and organizational performance: an
integrative view and empirical examination, Journal of
Management Information System, 20(1), 179-228.
Leonard-Barton, D. 1995. Wellsprings of Knowledge:
Building and Sustaining the Source of Innovation,
Harvard Business School Press, Boston, MA.
Liao, C., Palvia, P., & Lin, H-N. 2006. The Roles of Habit
and Web Site Quality in E-Commerce, International
Journal of Information Management, 26(6), 469-483.
Mills, A. & Smith, T. 2011. Knowledge Management and
Organizational Performance: A Decomposed View,
Journal of Knowledge Management, 15(1), 156-171.
Mom, T.J.M., Van Den Bosch, F.A.J. & Volberda, H. W.
2007. Investing managers’ exploration and exploitation
activities: the influence of top-down, bottom-up and
horizontal knowledge flows, Journal of Management
Studies, 44, 910-931.
Nevis, E., DiBella, A., Gould, J. 1998. Understanding
organizations as Learning Systems, https://
sloanreview.mit.edu/article/understanding-organizations
-as-learning-systems/ accessed on June 05, 2019
O’Dell, C., Hasanali, F., Hubert, C., Lopez, K. Odem, P. &
Raybourn, C. 2004. Successful KM Implementations: A
Study of Best Practice Organizations, Handbook of
Knowledge Management 2 – Knowledge Directions, pp.
411-441.
Park, S. C. 2007. The comparison of knowledge management
practices between public and private organizations: An
exploratory study, Dissertation, The Pennsylvania State
University.
Rao, M. 2005. Overview of KM Tools, in Knowledge
Management Tools and Techniques: Practitioners and
Experts Evaluate KM Solutions, Elsevier Butterworth-
Heinemann; Oxford UK.
Ringle, C.M., Sarstedt, M., Straub, D.W. 2012. A critical
look at the use of PLS-SEM in MIS quarterly, MIS
Quarterly 36 (1), 3-14.
Sarvary, M. 1999. Knowledge management and competition
in the service industry, California Management Review,
41(2), 95-107.
Seleim, A. & Khalil, O. 2007. Knowledge management and
organizational performance in the Egyptian software
firms, International Journal of Knowledge Management,
3(4), 37-66.
Singh, A. & Soltani, E. 2010. Knowledge management
practices in Indian information technology companies,
Total Quality Management, 21(2), 145-157.
Singh, A. K. & Sharma, V. 2011. Knowledge management
antecedents and its Impact on Employee Satisfaction – A
Study on Indian Telecommunication Industries, The
Learning Organization, 18(2), 115-130.
Smith, H. A. & McKeen, J. D. 2004. The Knowledge Chain
Model: Activities for Competiveness, Handbook of
Knowledge Management 2 – Knowledge Directions, pp.
395-410.
Sveiby, K. 2001. What is Knowledge Management?
available at https://www.sveiby.com/files/pdf/
whatisknowledgemanagement.pdf accessed on June 01,
2019.
Taylor, W. A. & Wright, G. H. 2004. Organizational
Readiness for Successful Knowledge Sharing:
Challenges for Public Sector Managers, Information
Resources Management Journal, 17(2), 22-37.
Wang, E., Klein, G. & Jiang, J. J. 2007. IT support in
manufacturing firms for a knowledge management
dynamic capability link to performance, International
Journal of Production Research, 45(11), 2419-2434.
Wong, K. Y. and Aspinwall, E. 2004. Knowledge
Management Implementation Framework: A Review,
Knowledge and Process Management, 11(2), 93-104.
Zack, M., McKeen, J. and Singh, S. 2009. Knowledge
Management and Organizational Performance: An
Exploratory Analysis, Journal of Knowledge
Management, 13(6), 392-409.
Zhou, A. Z. 2004. Managing Knowledge Strategically: A
Comparison of Managers' Perceptions between the
Private and Public Sector in Australia, Journal of
Information & Knowledge Management, 3(3), 213-222.
KMIS 2019 - 11th International Conference on Knowledge Management and Information Systems
434