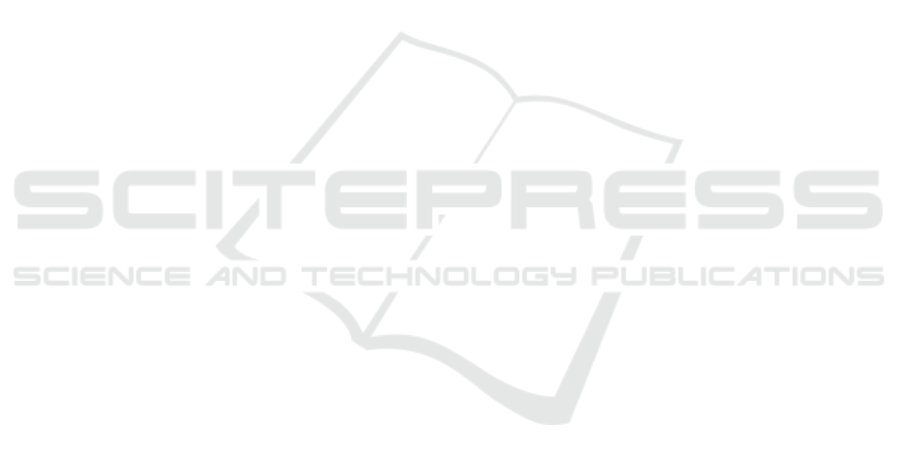
of them based on the probability that they contain a
tumor. Using the detection metrics, the performance
of the network was measured with the remaining 15%
of the dataset in order to evaluate its robustness. After
training the network, the results show that this pro-
posed computer-aided diagnosis method achieved a
mean accuracy of 97.375% proving that the system
could aid specialized doctors to recognize cancerous
signs when analyzing mammograms, improving pa-
tients’ quality of life.
In future works, the authors will study different
CNNs models and also other network architectures
like Mask R-CNNs instead of Faster R-CNNs in order
to not only locate the tumor inside the mammogram,
but also to create a mask with its shape. This way, the
size of the tumor can be estimated more precisely and
taken into account in the decision making task.
ACKNOWLEDGEMENTS
This work was supported by the excellence project
from the Spanish government grant (with support
from the European Regional Development Fund)
COFNET (TEC2016-77785-P).
REFERENCES
Akselrod-Ballin, A., Karlinsky, L., Alpert, S., Hasoul, S.,
Ben-Ari, R., and Barkan, E. (2016). A region based
convolutional network for tumor detection and classi-
fication in breast mammography. In Deep Learning
and Data Labeling for Medical Applications, pages
197–205. Springer.
Bray, F., Ferlay, J., Soerjomataram, I., Siegel, R. L., Torre,
L. A., and Jemal, A. (2018). Global cancer statistics
2018: Globocan estimates of incidence and mortality
worldwide for 36 cancers in 185 countries. CA: a can-
cer journal for clinicians, 68(6):394–424.
He, K., Zhang, X., Ren, S., and Sun, J. (2016). Deep resid-
ual learning for image recognition. In Proceedings of
the IEEE conference on computer vision and pattern
recognition, pages 770–778.
Heath, M., Bowyer, K., Kopans, D., Kegelmeyer, P., Moore,
R., Chang, K., and Munishkumaran, S. (1998). Cur-
rent status of the digital database for screening mam-
mography. In Digital mammography, pages 457–460.
Springer.
Heath, M., Bowyer, K., Kopans, D., Moore, R., and
Kegelmeyer, W. P. (2000). The digital database
for screening mammography. In Proceedings of the
5th international workshop on digital mammography,
pages 212–218. Medical Physics Publishing.
Hoang Ngan Le, T., Zheng, Y., Zhu, C., Luu, K., and Sav-
vides, M. (2016). Multiple scale faster-rcnn approach
to driver’s cell-phone usage and hands on steering
wheel detection. In Proceedings of the IEEE Con-
ference on Computer Vision and Pattern Recognition
Workshops, pages 46–53.
Jiang, H. and Learned-Miller, E. (2017). Face detection
with the faster r-cnn. In Automatic Face & Gesture
Recognition (FG 2017), 2017 12th IEEE International
Conference on, pages 650–657. IEEE.
Maitra, I. K., Nag, S., and Bandyopadhyay, S. K. (2012).
Technique for preprocessing of digital mammogram.
Computer methods and programs in biomedicine,
107(2):175–188.
Ponraj, D. N., Jenifer, M. E., Poongodi, P., and Manoha-
ran, J. S. (2011). A survey on the preprocessing tech-
niques of mammogram for the detection of breast can-
cer. Journal of Emerging Trends in Computing and
Information Sciences, 2(12):656–664.
Ren, S., He, K., Girshick, R., and Sun, J. (2015). Faster
r-cnn: Towards real-time object detection with region
proposal networks. In Advances in neural information
processing systems, pages 91–99.
Zhang, Q.-x., Lin, G.-h., Zhang, Y.-m., Xu, G., and Wang,
J.-j. (2018). Wildland forest fire smoke detection
based on faster r-cnn using synthetic smoke images.
Procedia engineering, 211:441–446.
NCTA 2019 - 11th International Conference on Neural Computation Theory and Applications
448