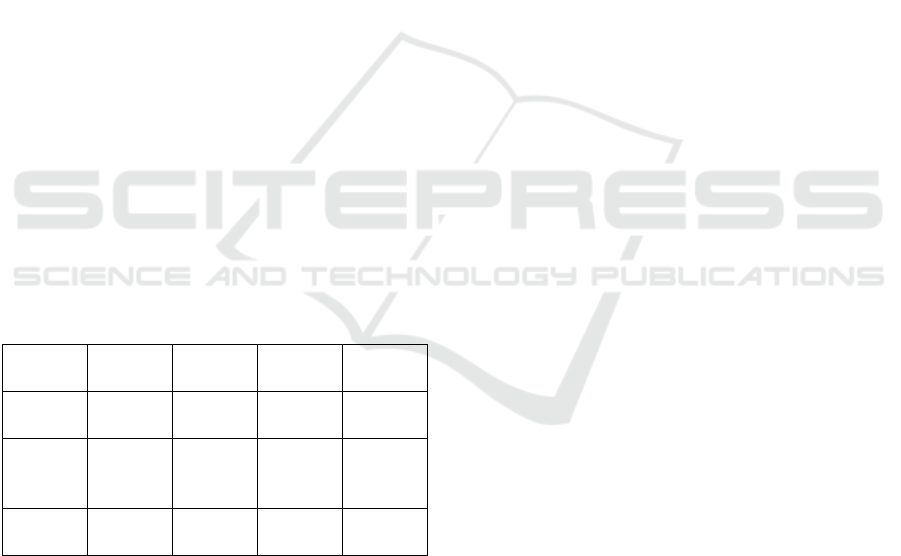
In all these cases the researchers have trained and
tested independently with the different datasets.
Even though our network is very light when we
train with a single dataset, we get similar results to
those obtained by other researchers. For DRISHTI
dataset training we obtained a Dice coefficient of 0.98
for both OD and OC segmentation. This compares
favourably with 0.97 and 0.87 (Zilly et al., 2017).
When training with RIM ONE we obtain 0.97 for OD
and 0.96 for OC. This also compares well with 0.98
and 0.94 (Shankaranarayana et al., 2017).
The most important result from table I comes
from the data that is not available in other studies, i.e.,
when we train with a dataset and use the network with
data captured with another source, we get poor
prediction result.
Table I also shows that when we train with a
combined dataset the network performs well doing
predictions from both datasets.
In Table II we show the percentage of the
predictions that estimate the radius with an error
below 10%. This data is clinically very relevant as the
ratio between the cup and disc radii, i.e., the CDR, is
directly related to glaucoma.
When we train with a specific dataset, almost all
the radii for the testing data from the same dataset are
predicted with less than 10% error. However, the radii
prediction for the other dataset are much worse and,
in some case, we never get errors below 10%. As can
be seem in the table this situation improves very
significatively when we train with a mixed dataset.
Table 2: Images with less than 10% radius error.
4 CONCLUSIONS AND FUTURE
WORK
We have been able to show that by using data from
different data sets, doing adequate image pre-
processing and performing very significant data
augmentation, both statically and dynamically, we
have been able to perform cup and disc segmentation
getting results with a performance that is equivalent
to that obtained by other authors using a single dataset
for evaluation and testing. This is, at least, a first
approach at the possibility of running this type of
segmentations as a service on the cloud.
We have also introduced a new clinically
significant parameter (Radii Ratio parameter- RRP)
that is very useful to estimate the accuracy of the
CDR.
We have shown that a very deep lightweight U-
Net derivative can perform as well as other heavier
less deeper alternatives for OD/OC segmentation.
This work has shown the advantages of using a
dataset that combines data from different sources
using aggressive data augmentation. Much work is
necessary to improve the commercial viability of this
type of service. In this work we have trained with a
mixed dataset but, in real life, we would have to start
training with the available data and do retraining as
more and more image data from different sources
becomes available. It would be necessary to
adequately study the behaviour of this type of trained
network with existing and new datasets.
ACKNOWLEDGEMENTS
This work was partially supported by the NPP project
funded by SAIT (2015-2018) and by the Spanish
government grant (with support from the European
Regional Development Fund) COFNET (TEC2016-77785-
P). Development in Cloud environment was supported by
Google Cloud platform research credit program
REFERENCES
Al-Bander, B., Williams, B., Al-Nuaimy, W., Al-Taee, M.,
Pratt, H., & Zheng, Y. (2018). Dense fully
convolutional segmentation of the optic disc and cup in
colour fundus for glaucoma diagnosis. Symmetry,
10(4), 87.
Fumero, F., Alayón, S., Sanchez, J. L., Sigut, J., &
Gonzalez-Hernandez, M. (2011). RIM-ONE: An open
retinal image database for optic nerve evaluation.
Paper presented at the 2011 24th international
symposium on computer-based medical systems
(CBMS).
Kim, S. J., Cho, K. J., & Oh, S. (2017). Development of
machine learning models for diagnosis of glaucoma.
PLoS One, 12(5), e0177726.
Litjens, G., Kooi, T., Bejnordi, B. E., Setio, A. A. A.,
Ciompi, F., Ghafoorian, M., . . . Sánchez, C. I. (2017).
A survey on deep learning in medical image analysis.
Medical image analysis, 42, 60-88.
Mary, M. C. V. S., Rajsingh, E. B., Jacob, J. K. K.,
Anandhi, D., Amato, U., & Selvan, S. E. (2015). An
NCTA 2019 - 11th International Conference on Neural Computation Theory and Applications
546