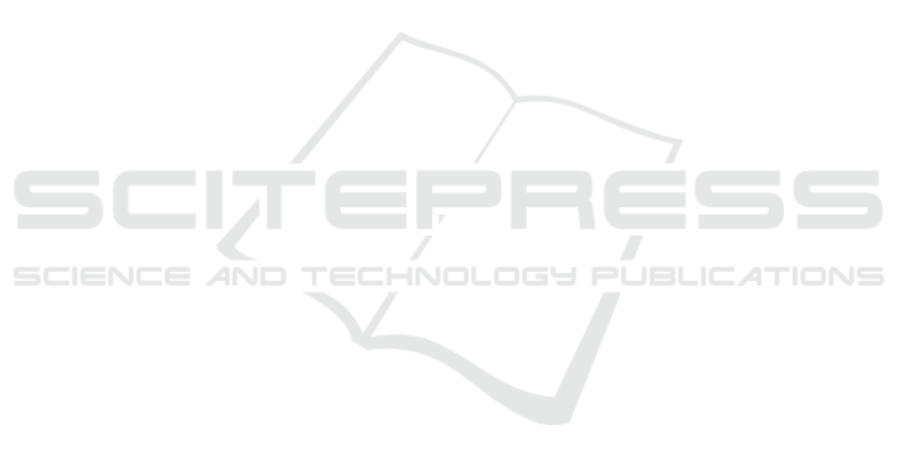
fortunately these types of deeper analysis are often
restrained from publication and research studies like
ours can rarely provide detailed experimental setup or
disclose chosen solution.
REFERENCES
Abdi, L. and Hashemi, S. (2016). To combat multi-class im-
balanced problems by means of over-sampling tech-
niques. IEEE Transactions on Knowledge & Data En-
gineering.
Ahmad, S., Lavin, A., Purdy, S., and Agha, Z. (2017). Un-
supervised real-time anomaly detection for streaming
data. Neurocomputing, 262:134–147.
Anandakrishnan, A., Kumar, S., Statnikov, A., Faruquie, T.,
and Xu, D. (2018). Anomaly Detection in Finance:
Editors’ Introduction. In KDD 2017 Workshop on
Anomaly Detection in Finance, pages 1–7.
Breunig, M. M., Kriegel, H.-P., Ng, R. T., and Sander, J.
(2000). LOF: identifying density-based local outliers.
In ACM sigmod record, volume 29, pages 93–104.
Chen, J., Shen, Y., and Ali, R. (2019). Credit Card Fraud
Detection Using Sparse Autoencoder and Generative
Adversarial Network. In 2018 IEEE 9th Annual In-
formation Technology, Electronics and Mobile Com-
munication Conference, IEMCON 2018, pages 1054–
1059. IEEE.
Deng, J., Zhang, Z., Marchi, E., and Schuller, B. (2013).
Sparse autoencoder-based feature transfer learning for
speech emotion recognition. In 2013 Humaine Asso-
ciation Conference on Affective Computing and Intel-
ligent Interaction, pages 511–516. IEEE.
Fu, K., Cheng, D., Tu, Y., and Zhang, L. (2016). Credit
card fraud detection using convolutional neural net-
works. In Lecture Notes in Computer Science (includ-
ing subseries Lecture Notes in Artificial Intelligence
and Lecture Notes in Bioinformatics), volume 9949
LNCS, pages 483–490. Springer, Cham.
Goodfellow, I., Pouget-Abadie, J., Mirza, M., Xu, B.,
Warde-Farley, D., Ozair, S., Courville, A., and Ben-
gio, Y. (2014). Generative adversarial nets. In
Advances in neural information processing systems,
pages 2672–2680.
Kamesh, V., Karthick, M., Kavin, K., Velusamy, M., and
Vidhya, R. (2019). Real-time fraud anomaly detec-
tion in e-banking using data mining algorithm. South
Asian Journal of Engineering and Technology, 8(S
1):144–148.
Krishnapriya, D. (2017). Identification of Money Laun-
dering based on Financial Action Task Force Using
Transaction Flow Analysis System. Bonfring Interna-
tional Journal of Industrial Engineering and Manage-
ment Science, 7(1):01–04.
Liu, F. T., Ting, K. M., and Zhou, Z.-H. (2008). Isolation
forest. In 2008 Eighth IEEE International Conference
on Data Mining, pages 413–422.
Mazzawi, H., Dalal, G., Rozenblatz, D., Ein-Dorx, L., Nin-
iox, M., and Lavi, O. (2017). Anomaly detection in
large databases using behavioral patterning. In Data
Engineering (ICDE), 2017 IEEE 33rd International
Conference on, pages 1140–1149.
Mosteller, F. and Tukey, J. W. (1968). Data analysis, in-
cluding statistics. In Lindzey, G. and Aronson, E., edi-
tors, Handbook of Social Psychology, Vol. 2. Addison-
Wesley.
Ngai, E. W. T., Hu, Y., Wong, Y. H., Chen, Y., and Sun, X.
(2011). The application of data mining techniques in
financial fraud detection: A classification framework
and an academic review of literature. Decision sup-
port systems, 50(3):559–569.
Quah, J. T. S. and Sriganesh, M. (2008). Real-time credit
card fraud detection using computational intelligence.
Expert systems with applications, 35(4):1721–1732.
R
¨
atsch, G., Sch
¨
olkopf, B., Mika, S., and M
¨
uller, K.-R.
(2000). SVM and boosting: One class. GMD-
Forschungszentrum Informationstechnik.
Rifai, S., Vincent, P., Muller, X., Glorot, X., and Bengio,
Y. (2011). Contractive auto-encoders: Explicit invari-
ance during feature extraction. In Proceedings of the
28th International Conference on International Con-
ference on Machine Learning, pages 833–840. Omni-
press.
Ryman-Tubb, N. F. and D’Avila Garcez, A. (2010).
SOAR - Sparse Oracle-based Adaptive Rule extrac-
tion: Knowledge extraction from large-scale datasets
to detect credit card fraud. In Proceedings of the Inter-
national Joint Conference on Neural Networks, pages
1–9. IEEE.
Sakurada, M. and Yairi, T. (2015). Anomaly Detection
Using Autoencoders with Nonlinear Dimensionality
Reduction. In Proceedings of the MLSDA 2014 2nd
Workshop on Machine Learning for Sensory Data
Analysis - MLSDA’14, pages 4–11, New York, New
York, USA. ACM Press.
Shanmugapriya, D. and Ganapathi, P. (2017). A Wrapper-
Based Classification Approach for Personal Identifi-
cation through Keystroke Dynamics Using Soft Com-
puting Techniques. In Identity Theft: Breakthroughs
in Research and Practice, pages 267–290. IGI Global.
Sundarkumar, G. G. and Ravi, V. (2015). A novel hybrid un-
dersampling method for mining unbalanced datasets
in banking and insurance. Engineering Applications
of Artificial Intelligence, 37:368–377.
Vincent, P., Larochelle, H., Bengio, Y., and Manzagol, P.-
A. (2008). Extracting and composing robust features
with denoising autoencoders. In Proceedings of the
25th international conference on Machine learning,
pages 1096–1103. ACM.
Wang, S., Liu, C., Gao, X., Qu, H., and Xu, W.
(2017). Session-Based Fraud Detection in Online E-
Commerce Transactions Using Recurrent Neural Net-
works. In Joint European Conference on Machine
Learning and Knowledge Discovery in Databases,
pages 241–252.
Zaslavsky, V. and Strizhak, A. (2006). Credit card fraud de-
tection using self-organizing maps. Information and
Security, 18:48.
Identify Theft Detection on e-Banking Account Opening
563