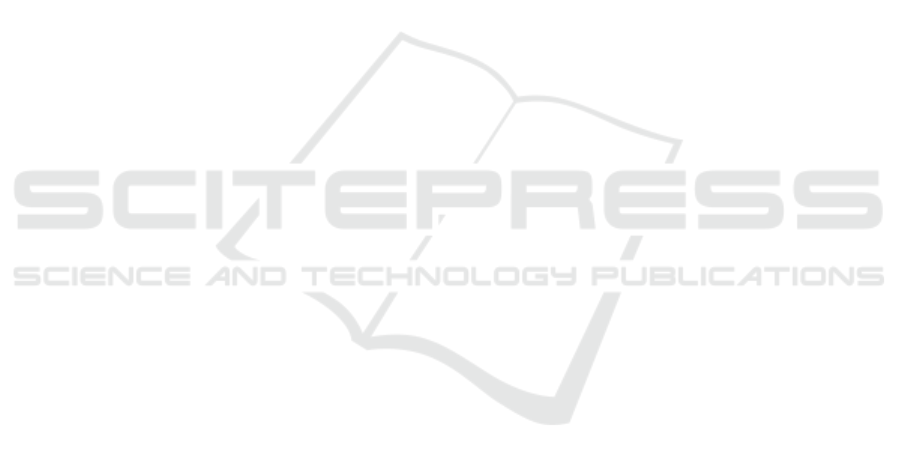
preventive measures to prevent occurrence and
corrective action in response if the impact occurs.
Based on this study, there are 10 highest risks in
management system process integration and 5 risks
occuring in scope component/clause.
ACKNOWLEDGMENTS
The authors would like to thank the financial support
provided by University of Indonesia University
through the PITTA 2019 funding scheme managed
by Directorate for Research and Public Services
(DRPM) University of Indonesia.
REFERENCES
Ajzen, I. (1991). The theory of planned behavior.
Organizational behavior and human decision
processes, 50(2):179–211.
Altintzoglou, T., Vanhonacker, F., Verbeke, W., and Luten,
J. (2011). Association of health involvement and
attitudes towards eating fish on farmed and wild
fish consumption in belgium, norway and spain.
Aquaculture International, 19(3):475–488.
Badr, L. M., Salwa, O., and Ahmed, Y. (2015). Perceived
barriers to consumption of freshwater fish in morocco.
British Food Journal, 117(1):274–285.
Bagozzi, R. P. and Yi, Y. (1988). On the evaluation of
structural equation models. Journal of the academy
of marketing science, 16(1):74–94.
Birch, D. and Lawley, M. (2012). Buying seafood:
Understanding barriers to purchase across
consumption segments. Food quality and preference,
26(1):12–21.
Cardoso, C., Lourenc¸o, H., Costa, S., Gonc¸alves, S.,
and Nunes, M. L. (2013). Survey into the
seafood consumption preferences and patterns in
the portuguese population. gender and regional
variability. Appetite, 64:20–31.
Carlucci, D., Nocella, G., De Devitiis, B., Viscecchia,
R., Bimbo, F., and Nardone, G. (2015). Consumer
purchasing behaviour towards fish and seafood
products. patterns and insights from a sample of
international studies. Appetite, 84:212–227.
Chin, W. W. et al. (1998). The partial least squares approach
to structural equation modeling. Modern methods for
business research, 295(2):295–336.
Esposito Vinzi, V., Chin, W. W., Henseler, J., and Wang, H.
(2010). Handbook of partial least squares: Concepts,
methods and applications. Heidelberg, Dordrecht,
London, New York: Springer.
Fornell, C. and Larcker, D. F. (1981). Structural equation
models with unobservable variables and measurement
error: Algebra and statistics.
Grieger, J. A., Miller, M., and Cobiac, L. (2012).
Knowledge and barriers relating to fish consumption
in older australians. Appetite, 59(2):456–463.
Hair, J. F., Hult, G. T. M., and Christian, M. Ringle. &
marko sarstedt. 2017. a primer on partial least squares
structural equation modeling (pls-sem).
Hair, J. F., Hult, G. T. M., Ringle, C. M., and Sarstedt, M.
(2013). A Primer on Partial Least Squares Structural
Equation Modeling (PLS-SEM). Thousand Oaks
Sage.
Hair, J. F., J., S., M., H., L., and Kuppelwieser, G.
(2014). V. Partial least squares structural equation
modeling (PLS-SEM) , European Business Review,
26(2):106–121.
Henseler, J., Ringle, C. M., and Sarstedt, M. (2015). A
new criterion for assessing discriminant validity in
variance-based structural equation modeling. Journal
of the academy of marketing science, 43(1):115–135.
Henseler, J., Ringle, C. M., and Sinkovics, R. R. (2009).
The use of partial least squares path modeling in
international marketing. In New challenges to
international marketing, pages 277–319. Emerald
Group Publishing Limited.
Hulland, J. (1999). Use of partial least squares (pls)
in strategic management research: A review of
four recent studies. Strategic management journal,
20(2):195–204.
Khan, A. Q., Aldosari, F., and Hussain, S. M. (2018).
Fish consumption behavior and fish farming attitude
in kingdom of saudi arabia (ksa). Journal of the Saudi
Society of Agricultural Sciences, 17(2):195–199.
Kline, R. B. (2005). Principles and practice of structural
equation modeling 2nd ed. New York: Guilford.
Meiselman, H. L. (1996). The contextual basis for
food acceptance, food choice and food intake: the
food, the situation and the individual. In Food
choice, acceptance and consumption, pages 239–263.
Springer.
Milo
ˇ
sevi
´
c, J.,
ˇ
Ze
ˇ
zelj, I., Gorton, M., and Barjolle, D.
(2012). Understanding the motives for food choice
in western balkan countries. Appetite, 58(1):205–214.
Moore, R. and Lopes, J. (1999). Paper templates.
In TEMPLATE’06, 1st International Conference on
Template Production. SCITEPRESS.
Murray, G., Wolff, K., and Patterson, M. (2017). Why eat
fish? factors influencing seafood consumer choices
in british columbia, canada. Ocean & Coastal
Management, 144:16–22.
Myrland, Ø., Trondsen, T., Johnston, R. S., and Lund,
E. (2000). Determinants of seafood consumption in
norway: lifestyle, revealed preferences, and barriers
to consumption. Food quality and Preference,
11(3):169–188.
Olsen, S. O. (2003). Understanding the relationship
between age and seafood consumption: the mediating
role of attitude, health involvement and convenience.
Food quality and Preference, 14(3):199–209.
ICoSET 2019 - The Second International Conference on Science, Engineering and Technology
96