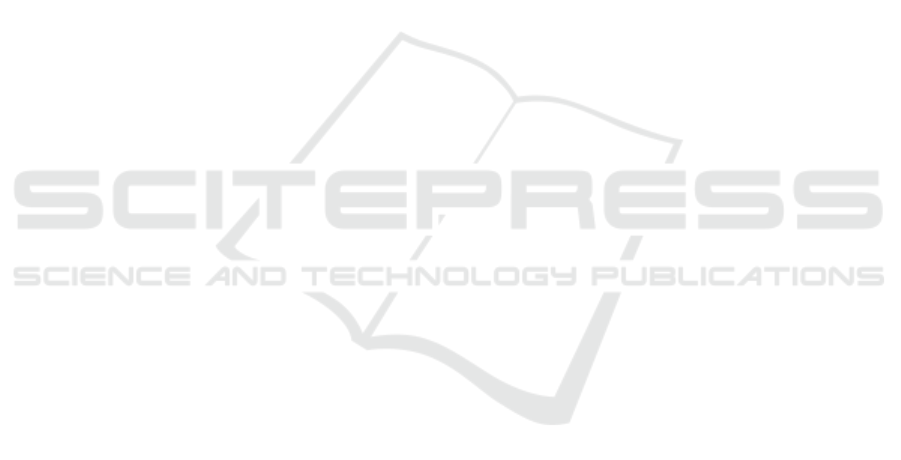
Blanchet, K., Nam, S.L., Ramalingam, B., Pozo-Martin, F.
(2017, April) Governance and capacity to manage
resilience of health systems: towards a new conceptual
framework. International Journal on Health Policy
Management, 6(8), 431-435. Retrieved from.
Dewi,S, Sitorus P,. 2016; Hubungan Kualitas Data DOTS
Program Dengan Peningkatan Kasus Tuberkulosis Di
Puskesmas Delitua.Jurnal Public Health Community
STIKes Delihusada Delitua.
Hanefeld, J., Mayhew, S., Legido-Quigley, H., Martineau,
F., Karanikolos, M., Blanchet, K., … Balabanova, D.
(2018). Towards an understanding of resilience:
responding to health systems shocks. Health Policy
Planning. 33(3), 355-367. Retrieved from.
Hartati, Dinata T, 2016; Hubungan Perilaku Organisasi
Dengan Qualitas Data SP2TP di Kabupaten Aceh
Utara, Tesis, Program Studi Kesehatan Masyarakat S2
STIKes Delihusada Delitua.
Hartzema, Abraham G; Reich, Christian G; Ryan, Patrick
B; Stang, Paul E; Madigan, David; Welebob; 2016;
Managing Data Quality for a Drug Safety Surveillance
System. Proquest
Ho, K., Al-Shorjabji, N., Brown, E., Zelmer, J., Gabor,
N., Maeder, A., Marcelo, A., … Doyle, T. (2016)
Applying the resilient health system framework for
universal health coverage. In Maeder, A., Ho, K.,
Marcelo, A., Warren, J. (Eds.), The Promise of New
Technologies in an Age of New Health Challenges
(pp. 54– 62). Amsterdam, Netherlands: IOS Press BV.
Khan, Y. O’Sullivan, T., Brown, A., Tracey, S., Gibson,
J., Généreux, M., … Schwartz, B. (2018) Public health
emergency preparedness: a framework to promote
resilience. BMC Public Health (18)1344.
Kim JH, Yim JJ; Achievements in and Challenges of
Tuberculosis Control in South Korea. Seoul National
University College of Medicine, Seoul, South Korea
2018; 21: 1913-20
Kruk, M., Myers, M., Varpilah, S.T., Dahn, B.T. (2015,
May). What is a resilient health system? Lessons from
Ebola.The Lancet Viewpoint. 385(9980), 1910-1912.
Lafond A, Field R. 2018. The Prism: Introducing an
analytical framework for understanding performance
of routine health information system in developing
(draft). RHINO 2nd International Workshop, South
Africa, MEASURE Evaluation
Lafound, Linda JS, 2017,. Performance of Routine
Information Systems Management (PRISM) Tools.
Oxford University Press
Lind A, Lind B. 2016. Practice of information system
development and use: a dialectical approach. System
Research & Behavioral Science 22: 453
Links, J., Schwartz, B.S., Lin, S., Kanarek, N., Mitrani-
Reiser, J., Sell, T.K., … Kendra J.M. (2017)
COPEWELL: A Conceptual Framework and System
Dynamics Model for Predicting Community
Functioning and Resilience After Disasters. Disaster
Medicine and Public Health Preparedness 12(1):127-
137.
Lippeveld T, Limprecht N, Gul Z. 1919. Assessment study
of the Pakistan Health Management Information
System. Government of Pakistan and USAID
Lippeveld T, Sauerborn R, Bodart C. 2018. Design and
implementation of health information systems.
Geneva: World Health Organization.
MEASURE Evaluation, 20017; A Conceptual Framework
for Data Demand and Information Use in the Health
Sector.
Mphatswe, W; Mate, K S; Bennett, B; Ngidi, H; Reddy, J;
Barker, P M; Rollins, 2016; Improving Public Health
Information: A Data Quality Intervention In Kwazulu-
Natal, South Africa. ProQuest
PRISM, 2009; Improving RHIS (Routine Health
Information System) Performance & Information Use
for Health System Management. US-AID
Purba BD, 2018; Pengaruh determinan sistem informasi
terhadap kualitas data dots program di puskesmas
kabupaten deli serdang. Researcgate,
https://www.researchgate.net/publication/331198113_
pengaruh_determinan_sistem_informasi_terhadap_kua
litas_data_dots_program_di_puskesmas_kabupaten_d
eli_serdang
Purba BD, 2019; pengaruh sosiaodemografi dan budaya
terhadap pencarian pengobatan pasien tuberculosis,
Researchgate,
https://www.researchgate.net/publication/334989132_
pengaruh_sosiaodemografi_dan_budaya_terhadap_pen
carian_pengobatan_pasien_tuberculosis
Ronveaux, O; Rickert, D; Hadler, S; Groom, H, 2016; The
Immunization Data Quality Audit: Verifying The
Quality And Consistency Of Immunization
Monitoring Systems. Proquest
Teklegiorgis, Kidist; Tadesse, Kidane; Mirutse,
Gebremeskel; Terefe, Wondwossen, 2016; Level Of
Data Quality From Health Management Information
Systems In A Resources Limited Setting And Its
Associated Factors, Eastern Ethiopia. ProQuest
US-AID, 2018.Data Quality Audit Tool.
http://www.cpc.unc.edu/measure. USA
WHO, 2016; Tuberculosis Report; WHO, Janeva
Identification Factors of Poor Quality of Data in the DOTS Program
65