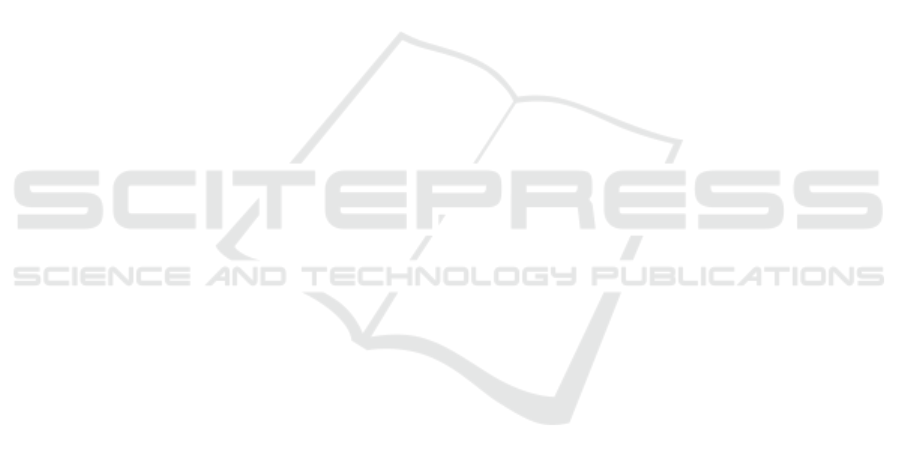
ume 116, AISC ’11, pages 57–66, Darlinghurst, Aus-
tralia, Australia. Australian Computer Society, Inc.
Chao, C.-F. and Horng, M.-H. (2015). The construction
of support vector machine classifier using the firefly
algorithm. Computat Intell Neurosci.
Clark, E. M., Jones, C. A., Williams, J. R., Kurti, A. N.,
Norotsky, M. C., Danforth, C. M., and Dodds, P. S.
(2016). Vaporous marketing: Uncovering pervasive
electronic cigarette advertisements on twitter. PLOS
ONE, 11(7):1–14.
Compton, R., Lee, C., Lu, T.-C., Silva, L. D., and Macy,
M. (2013). Detecting future social unrest in unpro-
cessed twitter data: “emerging phenomena and big
data”. 2013 IEEE International Conference on Intel-
ligence and Security Informatics, pages 56–60.
Cortes, C. and Vapnik, V. (1995). Support Vector Networks.
Mach Learn, 20:273–297.
Das, A., Gollapudi, S., Kiciman, E., and Varol, O.
(2016). Information dissemination in heterogeneous-
intent networks. In Proceedings of the 8th ACM Con-
ference on Web Science, WebSci ’16, pages 259–268,
New York, NY, USA. ACM.
Echeverr
´
ıa, J. and Zhou, S. (2017). The ’star wars’ botnet
with ¿350k twitter bots. CoRR, abs/1701.02405 arXiv
preprint arXiv:1701.02405.
elevenpaths (2018). Twitter bot-
nets detection in sports event.
https://blog.en.elevenpaths.com/2018/12/detection-
botnet-twitter-cybersecurity.html. (visited January
2019).
Ferrara, E., Varol, O., Menczer, F., and Flammini, A.
(2016a). Detection of promoted social media cam-
paigns. In Proceedings of the Tenth International Con-
ference on Web and Social Media, Cologne, Germany,
May 17-20, 2016., pages 563–566.
Ferrara, E., Wang, W., Varol, O., Flammini, A., and Gal-
styan, A. (2016b). Predicting online extremism, con-
tent adopters, and interaction reciprocity. In Social
Informatics - 8th International Conference, SocInfo
2016, Bellevue, WA, USA, November 11-14, 2016,
Proceedings, Part II, pages 22–39.
Goebel, J. and Holz, T. (2007). Rishi: Identify bot contam-
inated hosts by irc nickname evaluation. In Proceed-
ings of the First Conference on First Workshop on Hot
Topics in Understanding Botnets, HotBots’07, pages
8–8, Berkeley, CA, USA. USENIX Association.
Gorman, G. (2009). Google groups trojan.
http://www.symantec.com/connect/blogs/google-
groups-trojan. (visited January 2019).
Haustein, S., Bowman, T. D., Holmberg, K., Tsou, A., Sug-
imoto, C. R., and Larivi
`
ere, V. (2016). Tweets as im-
pact indicators: Examining the implications of auto-
mated ”bot” accounts on twitter. J. Assoc. Inf. Sci.
Technol., 67(1):232–238.
Jari, C., Wen, L., and Kang, L. (2008). Support vector ma-
chine classification for large data set vis minmum en-
closing ball clustering. Neurocomputing, 71:611–619.
Kohavi, R. (1995). A study of cross-validation and
bootstrap for accuracy estimation and model selec-
tion. In Proceedings of the Fourteenth International
Joint Conference on Artificial Intelligence, IJCAI 95,
Montr
´
eal Qu
´
ebec, Canada, August 20-25 1995, 2 Vol-
umes, pages 1137–1145. Morgan Kaufmann.
Liaw, A. and Wiener, M. (2001). Classification and regres-
sion by randomforest. Forest, 23.
Liu, X., Li, Q., Nourbakhsh, A., Fang, R., Thomas, M.,
Anderson, K., Kociuba, R., Vedder, M., Pomerville,
S., Wudali, R., Martin, R., Duprey, J., Vachher, A.,
Keenan, W., and Shah, S. (2016). Reuters tracer: A
large scale system of detecting & verifying real-
time news events from twitter. In Proceedings of the
25th ACM International on Conference on Informa-
tion and Knowledge Management, CIKM ’16, pages
207–216, New York, NY, USA. ACM.
Lokot, T. and Diakopoulos, N. (2016). News bots: Au-
tomating news and information dissemination on twit-
ter. Digital Journalism, 4(6):682–699.
Mathews, P., Gray, C., Mitchell, L., Nguyen, G. T., and
Bean, N. G. (2018). SMERC: social media event re-
sponse clustering using textual and temporal informa-
tion. CoRR, abs/1811.05063.
Min, J. H. and Lee, Y. (2005). Bankruptcy prediction using
support vector machine with optimal choice of kernel
function parameters. Expert Syst. Appl., 28(4):603–
614.
Quinlan, J. (1986). Induction of decision trees. Mach Learn,
1:181–186.
Romera, R. (2010). Discerning relationships: The mexican
botnet connection. Trend Micro.
Savage, S., Monroy-Hernandez, A., and H
¨
ollerer, T. (2016).
Botivist: Calling volunteers to action using online
bots. In Proceedings of the 19th ACM Conference
on Computer-Supported Cooperative Work & Social
Computing, CSCW ’16, pages 813–822, New York,
NY, USA. ACM.
Takeichi, Y., Sasahara, K., Suzuki, R., and Arita, T. (2015).
Concurrent bursty behavior of social sensors in sport-
ing events. PloS one, 10 12:e0144646.
Varol, O., Ferrara, E., Davis, C. A., Menczer, F., and Flam-
mini, A. (2017). Online human-bot interactions: De-
tection, estimation, and characterization. In Interna-
tional AAAI Conference on Web and Social Media,
page 280–289. AAAI, AAAI.
Wang, G., Konolige, T., Wilson, C., Wang, X., Zheng, H.,
and Zhao, B. Y. (2013). You are how you click: Click-
stream analysis for sybil detection. In Proceedings of
the 22Nd USENIX Conference on Security, SEC’13,
pages 241–256, Berkeley, CA, USA. USENIX Asso-
ciation.
Yang, Z., Wilson, C., Wang, X., Gao, T., Zhao, B. Y., and
Dai, Y. (2014). Uncovering social network sybils in
the wild. ACM Trans. Knowl. Discov. Data, 8(1):2:1–
2:29.
Zheng, X., Zeng, Z., Chen, Z., Yu, Y., and Rong, C. (2015).
Detecting spammers on social networks. Neurocom-
puting, 159:27 – 34.
ECTA 2020 - 12th International Conference on Evolutionary Computation Theory and Applications
154