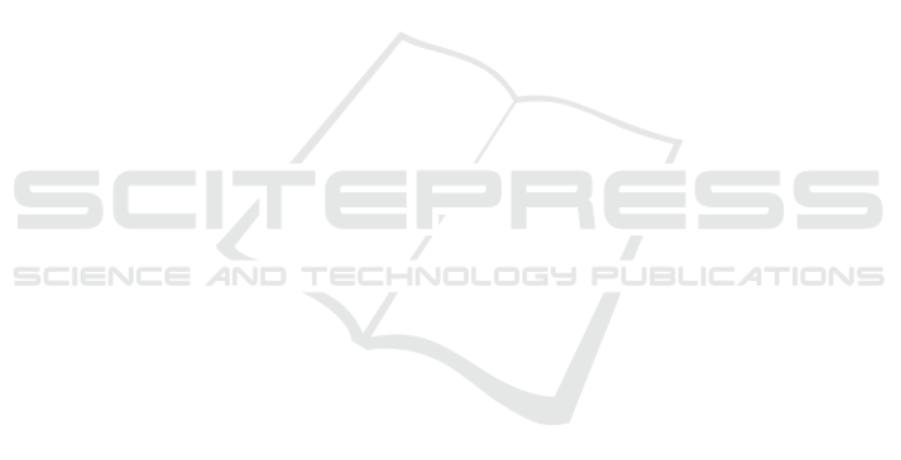
ond, the construction of seed graph can adopt the En-
terprise Architecture, which provides a comprehen-
sive view of business; and the last, expert knowledge
needs crowd sourcing too, especially when the knowl-
edge are cross different domains. And the validation
of expert driven crowd sourcing is another challenge.
5 CONCLUSION
This paper proposes a framework to solve enterprise
knowledge graph cold start problem. The proposed
framework takes the advantage of graph-formed ex-
pert knowledge, and use it to guide the clustering
method, which integrates expert knowledge with data
driven clustering.
Coarser cluster level linkage, and finer entity level
linkage are adopted base on entity vectorization. In-
cremental validation is used to gradually add the
words in graph. The framework is validated through
an enterprise finance knowledge graph to support rea-
sonable knowledge consumption. Search engines and
Q&A apps will be constructed base on this graph, and
the current available data-driven techniques will be
used to expand and fine tune the graph.
REFERENCES
Carlson, A., Betteridge, J., Kisiel, B., Settles, B., Jr., E.
R. H., and Mitchell1, T. M. (2010). Toward an archi-
tecture for never-ending language learning.
Dong, X. L. (2018). Challenges and innovations in building
a product knowledge graph. In 24th ACM SIGKDD
International Conference on Knowledge Discovery
&Data Mining, pages 2869–2869.
Duan, R. and Xiao, Y. (2019). Enterprise knowledge graph
from specific business task to enterprise knowledge
management. In Proceedings of the 28th ACM Inter-
national Conference on Information and Knowledge
Management, CIKM ’19, page 2965–2966.
Ferrucci, D. and Brown, E. (2011). Adaptwatson: A
methodology for developing and adapting watson
technology. IBM, Armonk, NY, IBM Res. Rep.,
RC25244.
Fortunato, S. (2009). Community detection in graphs.
Physics Reports, 486(3):75–174.
Galkin, M., Auer, S., Vidal, M.-E., and Scerri, S. (2017).
Enterprise knowledge graphs: A semantic approach
for knowledge management in the next generation of
enterprise information systems. In International Con-
ference on Enterprise Information Systems (ICEIS),
volume 2, pages 88–98, Porto, Portugal. SciTePress.
Geng, L., Semerci, M., Yener, B., and Zaki, M. J. (2012).
Effective graph classification based on topological and
label attributes. Statistical Analysis & Data Mining
the Asa Data Science Journal, 5(4):265–283.
Liu, J., Shang, J., Wang, C., Ren, X., and Han, J. (2015).
Mining quality phrases from massive text corpora.
In 2015 ACM SIGMOD International Conference on
Management of Data, pages 1729–1744. ACM.
L
¨
u, L. and Zhou, T. (2011). Link prediction in complex
networks: A survey. Physica A: statistical mechanics
and its applications, 390(6):1150–1170.
Mart
´
ınez, V., Berzal, F., and Cubero, J.-C. (2017). A survey
of link prediction in complex networks. ACM Com-
puting Surveys (CSUR), 49(4):69.
Menon, A. K. and Elkan, C. (2011). Link prediction
via matrix factorization. In Joint european confer-
ence on machine learning and knowledge discovery
in databases, pages 437–452. Springer.
Pan, J. Z., Vetere, G., Gomez-Perez, J. M., and Wu,
H. (2017). Exploiting Linked Data and Knowledge
Graphs in Large Organisations. Springer Publishing
Company, Incorporated, 1st edition.
Ramaswami, P. (2007). A remedy for your health-related
questions: health info in the knowledge graph.
Rogers, D. and Hahn, M. (2010). Extended-connectivity
fingerprints. Journal of Chemical Information & Mod-
eling, 50(5):742–754.
Shang, J., Liu, J., Meng, J., Xiang, R., Voss, C. R., and Han,
J. (2017). Automated phrase mining from massive text
corpora. IEEE Transactions on Knowledge & Data
Engineering, PP(99):1–1.
Shervashidze, N., Vishwanathan, S. V. N., Petri, T.,
Mehlhorn, K., and Borgwardt, K. M. (2009). Ef-
ficient graphlet kernels for large graph comparison.
In 12th International Conference on Artificial Intel-
ligence and Statistics, pages 488–495.
Suchanek, F. M., Kasneci, G., and Weikum, G. (2007).
Yago: a core of semantic knowledge. pages 697–706.
Taskar, B., Wong, M.-F., Abbeel, P., and Koller, D. (2004).
Link prediction in relational data. In Advances in neu-
ral information processing systems, pages 659–666.
Wang, J., Zhang, Z., and Zha, H. (2005). Adaptive manifold
learning. In Advances in neural information process-
ing systems, pages 1473–1480.
Wu, X., Zhao, L., and Akoglu, L. (2018). A quest for struc-
ture: Jointly learning the graph structure and semi-
supervised classification. In 27th ACM International
Conference on Information and Knowledge Manage-
ment, CIKM ’18, pages 87–96. ACM.
Zhang, X. and Lee, W. S. (2007). Hyperparameter learn-
ing for graph based semi-supervised learning algo-
rithms. In Advances in neural information processing
systems, pages 1585–1592.
Zhu, X., Ghahramani, Z., and Lafferty, J. D. (2003). Semi-
supervised learning using gaussian fields and har-
monic functions. In 20th International conference on
Machine learning (ICML-03), pages 912–919.
KMIS 2020 - 12th International Conference on Knowledge Management and Information Systems
160