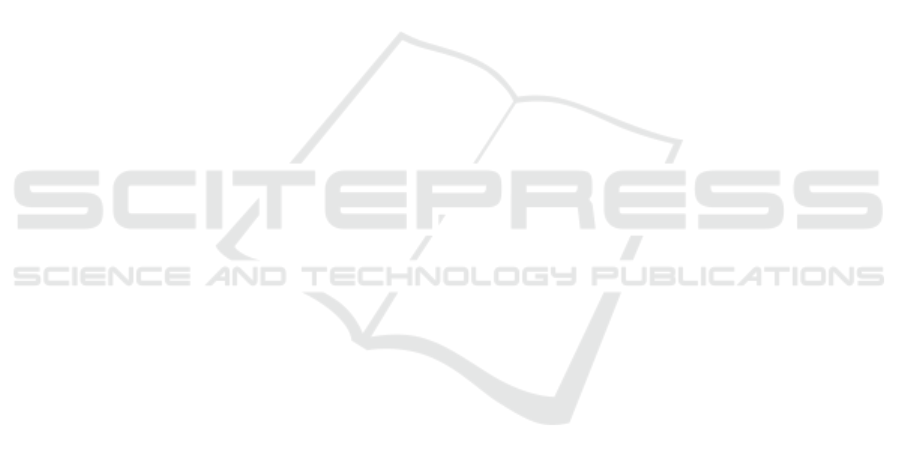
search and Development in Information Retrieval, SI-
GIR ’09, pages 267–274, New York, NY, USA. ACM.
Herskovic, J. R., Tanaka, L. Y., Hersh, W., and Bernstam,
E. V. (2007). A day in the life of PubMed: analysis
of a typical day’s query log. Journal of the American
Medical Informatics Association, 14(2):212–220.
Hoffart, J., Altun, Y., and Weikum, G. (2014). Discovering
emerging entities with ambiguous names. In WWW
2014 - Proceedings of the 23rd International Confer-
ence on World Wide Web, WWW ’14, pages 385–395,
New York, NY, USA. ACM.
Honnibal, M. and Montani, I. (2017). spaCy 2: Natural lan-
guage understanding with Bloom embeddings, convo-
lutional neural networks and incremental parsing.
Huth, E. J. (1989). The information explosion. Bulletin of
the New York Academy of Medicine, 65(6):647.
Kleppmann, M. (2017). Designing data-intensive appli-
cations: the big ideas behind reliable, scalable, and
maintainable systems. O’Reilly Media, Boston, first
edition edition. OCLC: ocn893895983.
Krasner, G. E., Pope, S. T., and others (1988). A description
of the model-view-controller user interface paradigm
in the smalltalk-80 system. Journal of object oriented
programming, 1(3):26–49.
Lafferty, J., McCallum, A., and Pereira, F. C. N. (2001).
Conditional random fields: Probabilistic models for
segmenting and labeling sequence data.
Lee, J., Yoon, W., Kim, S., Kim, D., Kim, S., So, C. H.,
and Kang, J. (2019). BioBERT: a pre-trained biomed-
ical language representation model for biomedical text
mining. pages 1–8.
Lema
ˆ
ıtre, G., Nogueira, F., and Aridas, C. K. (2017).
Imbalanced-learn: A Python Toolbox to Tackle the
Curse of Imbalanced Datasets in Machine Learning.
Journal of Machine Learning Research, 18(17):1–5.
Ling Charles X., , and Sheng, V. S. (2010). Class Imbal-
ance Problem. In Sammut Claude, , and Webb, G. I.,
editors, Encyclopedia of Machine Learning, page 171.
Springer US, Boston, MA.
Lipscomb, C. E. (2000). Medical subject headings
(MeSH). Bulletin of the Medical Library Association,
88(3):265.
Manning, C. D., Raghavan, P., and Sch
¨
utze, H. (2008). In-
troduction to information retrieval. Cambridge Uni-
versity Press, New York.
Meij, E., Balog, K., and Odijk, D. (2013). Entity Link-
ing and Retrieval. In Proceedings of the 36th Interna-
tional ACM SIGIR Conference on Research and De-
velopment in Information Retrieval, SIGIR ’13, page
1127, New York, NY, USA. ACM.
Mosa, A. S. M. and Yoo, I. (2013). A study on pubmed
search tag usage pattern: Association rule mining of a
full-day pubmed query log. BMC Medical Informatics
and Decision Making, 13(1).
Nadeau, D. and Sekine, S. (2007). A survey of named entity
recognition and classification. Lingvisticae Investiga-
tiones, 30(1):3–26.
Nawroth, C., Duttenh
¨
ofer, A., and Hemmje, M. (2020). Ar-
gumentationsunterst
¨
utzung durch emergentes Wissen
in der Medizin. In to appear in: (Wilhelm Bauer,
Joachim Warschat, Innovation durch Natural Lan-
guage Processing - Mit K
¨
unstlicher Intelligenz die
Wettbewerbsf
¨
ahigkeit verbessern.
Nawroth, C., Engel, F., Eljasik-Swoboda, T., and Hemmje,
M. (2018). Towards enabling emerging named entity
recognition as a clinical information and argumenta-
tion support. In DATA 2018 - Proceedings of the 7th
International Conference on Data Science, Technol-
ogy and Applications.
Nawroth, C., Engel, F., Mc Kevitt, P., and Hemmje, M. L.
(2019). Emerging Named Entity Recognition on Re-
trieval Features in an Affective Computing Corpus. In
2019 IEEE International Conference on Bioinformat-
ics and Biomedicine (BIBM), pages 2860–2868.
Norman, D. A. and Draper, S. W. (1986). User centered
system design: New perspectives on human-computer
interaction. CRC Press.
of Bielefeld, U. (2017). Rationalizing Recommendations
(RecomRatio): Project-Homepage. Bielefeld.
Petkova, D. and Croft, W. B. (2007). Proximity-based doc-
ument representation for named entity retrieval. In
Proceedings of the sixteenth ACM conference on Con-
ference on information and knowledge management,
pages 731–740. ACM, Lisbon, Portugal.
Piccinno, F. and Ferragina, P. (2014). From TagME to WAT:
A New Entity Annotator. In Proceedings of the First
International Workshop on Entity Recognition & Dis-
ambiguation, ERD ’14, pages 55–62, New York, NY,
USA. ACM.
Sang, Erik F. Tjong Kim and de Meulder, F. (2003). In-
troduction to the CoNLL-2003 shared task: language-
independent named entity recognition. In Proceed-
ings of the seventh conference on Natural language
learning at HLT-NAACL 2003 - Volume 4, pages 142–
147. Association for Computational Linguistics, Ed-
monton, Canada.
Vu, B. and Hemmje, M. (2019). Supporting Taxonomy De-
velopment and Evolution by Means of Crowdsourc-
ing:. In Proceedings of the 11th International Joint
Conference on Knowledge Discovery, Knowledge En-
gineering and Knowledge Management, pages 351–
358, Vienna, Austria. SCITEPRESS - Science and
Technology Publications.
Wang, B., Yang, B., Shan, S., and Chen, H. (2019). De-
tecting Hot Topics From Academic Big Data. IEEE
Access, 7:185916–185927. Publisher: IEEE.
Yao, K., Zweig, G., Hwang, M. Y., Shi, Y., and Yu, D.
(2013). Recurrent neural networks for language un-
derstanding. In Yao, K., Zweig, G., Hwang, M.-Y.,
Shi, Y., and Yu, D., editors, Proceedings of the Annual
Conference of the International Speech Communica-
tion Association, INTERSPEECH, pages 2524–2528.
Zheng, A. and Casari, A. Feature engineering for machine
learning : principles and techniques for data scien-
tists.
Zhou, G. and Su, J., editors (2002). Named entity recogni-
tion using an HMM-based chunk tagger. Association
for Computational Linguistics.
Emerging Named Entity Recognition in a Medical Knowledge Management Ecosystem
41