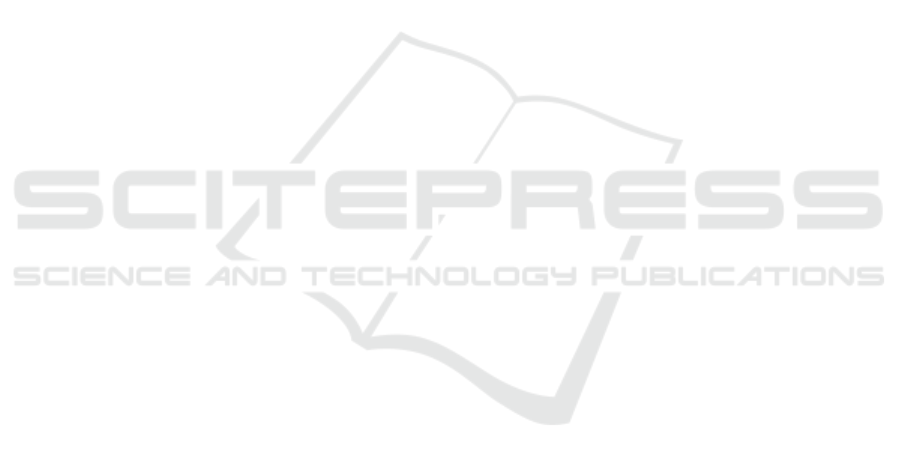
Henzler-Wildman, K. and Kern, D. (2007). Dynamic per-
sonalities of proteins. Nature, 450(7172):964–972.
Hoffmann, A. A. and Willi, Y. (2008). Detecting genetic
responses to environmental change. Nature Reviews
Genetics, 9(6):421–432.
Kauffman, S., Peterson, C., Samuelsson, B., and Troein,
C. (2003). Random boolean network models and the
yeast transcriptional network. Proceedings of the Na-
tional Academy of Sciences, 100(25):14796–14799.
Keedwell, E., Narayanan, A., and Savic, D. (2002). Mod-
elling gene regulatory data using artificial neural net-
works. In Proceedings of the 2002 International Joint
Conference on Neural Networks. IJCNN’02 (Cat. No.
02CH37290), volume 1, pages 183–188. IEEE.
Kitano, H. (2004). Biological robustness. Nature Reviews
Genetics, 5(11):826–837.
LeCun, Y., Denker, J. S., and Solla, S. A. (1990). Opti-
mal brain damage. In Advances in neural information
processing systems, pages 598–605.
Lifkooee, M. Z., Soysal,
¨
O. M., and Sekeroglu, K. (2019).
Video mining for facial action unit classification us-
ing statistical spatial–temporal feature image and log
deep convolutional neural network. Machine Vision
and Applications, 30(1):41–57.
Lones, M. A., Tyrrell, A. M., Stepney, S., and Caves, L. S.
(2010). Controlling complex dynamics with artifi-
cial biochemical networks. In Esparcia-Alc
´
azar, A. I.,
Ek
´
art, A., Silva, S., Dignum, S., and Uyar, A. S¸., ed-
itors, Genetic Programming, pages 159–170, Berlin,
Heidelberg. Springer Berlin Heidelberg.
Lu, Z., Whalen, I., Boddeti, V., Dhebar, Y., Deb, K., Good-
man, E., and Banzhaf, W. (2019). Nsga-net: neural
architecture search using multi-objective genetic algo-
rithm. In Proceedings of the Genetic and Evolutionary
Computation Conference, pages 419–427.
MacNeil, L. T. and Walhout, A. J. (2011). Gene regulatory
networks and the role of robustness and stochasticity
in the control of gene expression. Genome research,
21(5):645–657.
Marques, J., Bernardes, J., and Ayres de Cam-
pos, D. Cardiotocography data set.
https://archive.ics.uci.edu/ml/datasets/Cardiotocography.
M
¨
uller, G. B. and Olsson, L. (2003). Epigenesis and epige-
netics. Keywords and concepts in evolutionary devel-
opmental biology, 114.
Murawska, M. and Brehm, A. (2011). Chd chromatin re-
modelers and the transcription cycle. Transcription,
2(6):244–253.
Nassif, A. B., Shahin, I., Attili, I., Azzeh, M., and Shaalan,
K. (2019). Speech recognition using deep neural net-
works: A systematic review. IEEE Access, 7:19143–
19165.
Nguyen, A., Yosinski, J., and Clune, J. (2015). Deep neural
networks are easily fooled: High confidence predic-
tions for unrecognizable images. In Proceedings of
the IEEE conference on computer vision and pattern
recognition, pages 427–436.
Oh, S. J., Schiele, B., and Fritz, M. (2019). Towards
reverse-engineering black-box neural networks. In
Explainable AI: Interpreting, Explaining and Visual-
izing Deep Learning, pages 121–144. Springer.
Phillips, T. and Shaw, K. (2008). Chromatin remodeling in
eukaryotes. Nature Education, 1(1):209.
Polo, S. E. and Almouzni, G. (2006). Chromatin assembly:
a basic recipe with various flavours. Current opinion
in genetics & development, 16(2):104–111.
Riggs, A. D., Russo, V. E. A., and Martienssen, R. A.
(1996). Introduction. In Epigenetic mechanisms
of gene regulation. Cold Spring Harbor Laboratory
Press.
Shrestha, A. and Mahmood, A. (2019). Optimizing deep
neural network architecture with enhanced genetic al-
gorithm. In 2019 18th IEEE International Conference
On Machine Learning And Applications (ICMLA),
pages 1365–1370. IEEE.
Turner, A. and Dethlefs, N. (2017). Transparency of execu-
tion using epigenetic networks. In European Confer-
ence on Artificial Life, volume 14, pages 404–411.
Turner, A. P., Caves, L. S. D., Stepney, S., Tyrrell, A. M.,
and Lones, M. A. (2017). Artificial epigenetic net-
works: Automatic decomposition of dynamical con-
trol tasks using topological self-modification. IEEE
Transactions on Neural Networks and Learning Sys-
tems, 28(1):218–230.
Turner, A. P., Lones, M. A., Fuente, L. A., Stepney, S.,
Caves, L. S., and Tyrrell, A. M. (2012). Using arti-
ficial epigenetic regulatory networks to control com-
plex tasks within chaotic systems. In Lones, M. A.,
Smith, S. L., Teichmann, S., Naef, F., Walker, J. A.,
and Trefzer, M. A., editors, Information Processing
in Cells and Tissues, pages 1–11, Berlin, Heidelberg.
Springer Berlin Heidelberg.
Turner, A. P., Lones, M. A., Fuente, L. A., Stepney, S.,
Caves, L. S. D., and Tyrrell, A. (2013). The artifi-
cial epigenetic network. In 2013 IEEE International
Conference on Evolvable Systems (ICES), pages 66–
72.
Waddington, C. H. et al. (1939). An introduction to modern
genetics. An introduction to modern genetics.
White, P., Zykov, V., Bongard, J. C., and Lipson, H. (2005).
Three dimensional stochastic reconfiguration of mod-
ular robots. In Robotics: Science and Systems, pages
161–168. Cambridge.
Zhang, S., Yao, L., Sun, A., and Tay, Y. (2019). Deep
learning based recommender system: A survey and
new perspectives. ACM Computing Surveys (CSUR),
52(1):5.
Improving the Transparency of Deep Neural Networks using Artificial Epigenetic Molecules
175