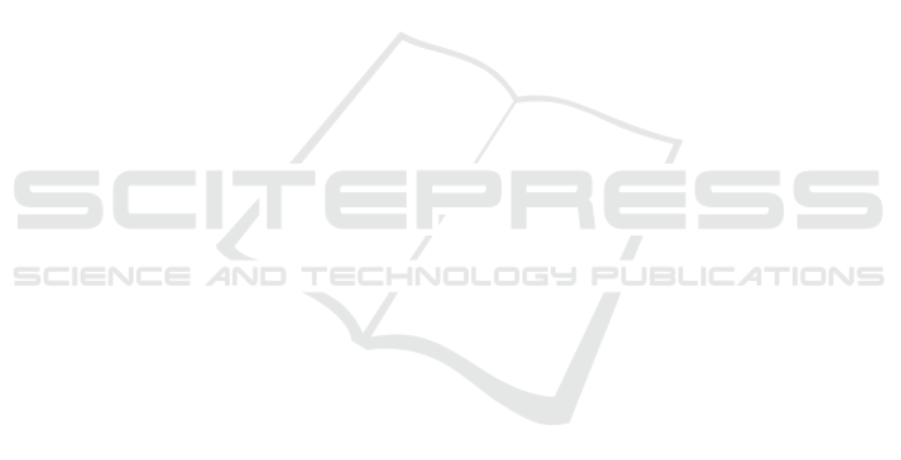
Experiments results show that regardless of the N
s
parameter, when the proposed framework applied, RS
models show better performance than Random RS for
both datasets. It can be seen from the results that
when RS does not have any information about the
new session (cold-start session), the performance of
RS is low. However, where a session has interacted
items, the performance of the RS models improves
significantly. For example, when sessions have inter-
acted items, experiment results show that Item-Item
KNN based RS can show around 41% and 55 % re-
call scores in ds 1 and ds 2 respectively.
On the other hand, when the proposed framework
is applied to two well-known RS models, it can be
seen that the recall score can increase significantly in
comparison with random RS model in cold-start ses-
sions. In the results table, we also show the impact of
N
s
parameter on RS performance. The results indicate
that increasing N
s
parameter after a certain point does
not help improve the results. We found that N
s
= 30
is the best value for achieving the best RS accuracy
score. Moreover, in each N
s
parameter and dataset,
the experiment results showed that Item-Item KNN
based RS is better than Session Popularity based RS
model.
4.5 Conclusion
Cold-start problems are major problems of the RS. In
this paper, we introduced a novel framework that can
deal with the cold-start problem for the new sessions
that have no prior user information such as item in-
teractions. The proposed framework can find similar
sessions based on session features such as time ses-
sion started, users’ location, users’ platform, etc. and
create recommendations from these sessions. Com-
putational experiments using two datasets proved that
the proposed framework significantly improved rec-
ommendation accuracy and alleviate cold-start user
session problem. We applied the proposed framework
on Item-Item KNN and Session Popularity based RS
models. Nevertheless, the proposed framework is a
general framework that can be applied to different
kinds of RS model, including Deep-Learning based
models (Hidasi et al., 2015; Gabriel De Souza et al.,
2019; Ludewig et al., 2019).
For future work, other session-based datasets
could be tested on the framework to substantiate the
robustness of the proposed framework. Also, clus-
tering approach (Zhang et al., 2013; Yanxiang et al.,
2013; Bader-El-Den et al., 2018) can be applied to
find similar sessions. For example, in the first step,
sessions can be clustered into groups, and based on
new session features the cluster group that session
belongs to can be identified then most similar ses-
sions from that cluster can be used in order to create
a recommendation list. Also, other available session
features can be included for nearest neighbour model
creation to find similar sessions that can represent new
sessions more accurately.
REFERENCES
Aharon, M., Anava, O., Avigdor-Elgrabli, N., Drachsler-
Cohen, D., Golan, S., and Somekh, O. (2015). Ex-
cuseme: Asking users to help in item cold-start rec-
ommendations. In Proceedings of the 9th ACM Con-
ference on Recommender Systems, pages 83–90.
Aledo, J. A., G
´
amez, J. A., and Molina, D. (2017). Tack-
ling the supervised label ranking problem by bagging
weak learners. Information Fusion, 35:38–50.
Anwaar, F., Iltaf, N., Afzal, H., and Nawaz, R. (2018). Hrs-
ce: A hybrid framework to integrate content embed-
dings in recommender systems for cold start items.
Journal of computational science, 29:9–18.
Bader-El-Den, M., Teitei, E., and Perry, T. (2018). Biased
random forest for dealing with the class imbalance
problem. IEEE transactions on neural networks and
learning systems, 30(7):2163–2172.
Bouadjenek, M. R., Hacid, H., and Bouzeghoub, M. (2016).
Social networks and information retrieval, how are
they converging? a survey, a taxonomy and an analy-
sis of social information retrieval approaches and plat-
forms. Information Systems, 56:1–18.
Carrer-Neto, W., Hern
´
andez-Alcaraz, M. L., Valencia-
Garc
´
ıa, R., and Garc
´
ıa-S
´
anchez, F. (2012). Social
knowledge-based recommender system. application to
the movies domain. Expert Systems with applications,
39(12):10990–11000.
Castillejo, E., Almeida, A., and L
´
opez-De-Ipi
˜
na, D. (2012).
Alleviating cold-user start problem with users’ social
network data in recommendation systems. In Work-
shop on Preference Learning: Problems and Applica-
tions in AI (PL-12) at ECAI, pages 28–33.
Esmeli, R., Bader-El-Den, M., and Abdullahi, H. (2019a).
Improving session based recommendation by diver-
sity awareness. In UK Workshop on Computational
Intelligence, pages 319–330. Springer.
Esmeli, R., Bader-El-Den, M., Abdullahi, H., and Hender-
son, D. (2020). Improving session-based recommen-
dation adopting linear regression based re-ranking. In
IEEE World Congress on Computational Intelligence
(WCCI) 2020. Institute of Electrical and Electronics
Engineers.
Esmeli, R., Bader-El-Den, M., and Mohasseb, A. (2019b).
Context and short term user intention aware hybrid
session based recommendation system. In 2019 IEEE
International Symposium on INnovations in Intelli-
gent SysTems and Applications (INISTA), pages 1–6.
IEEE.
Gabriel De Souza, P. M., Jannach, D., and Da Cunha, A. M.
(2019). Contextual hybrid session-based news recom-
Session Similarity based Approach for Alleviating Cold-start Session Problem in e-Commerce for Top-N Recommendations
185