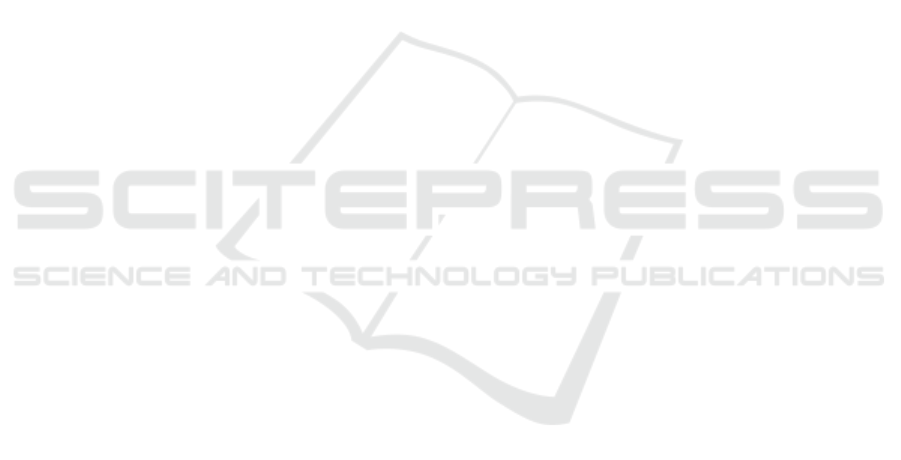
REFERENCES
Bacciu, D., Micheli, A., and Sperduti, A. (2018). Gen-
erative kernels for tree-structured data. IEEE trans-
actions on neural networks and learning systems,
29(10):4932–4946.
Bai, L., Hancock, E. R., Han, L., and Ren, P. (2012). Graph
clustering using graph entropy complexity traces. In
Proceedings of the 21st International Conference on
Pattern Recognition (ICPR2012), pages 2881–2884.
Baldini, L., Martino, A., and Rizzi, A. (2019a). Stochastic
information granules extraction for graph embedding
and classification. In Proceedings of the 11th Inter-
national Joint Conference on Computational Intelli-
gence - Volume 1: NCTA, (IJCCI 2019), pages 391–
402. INSTICC, SciTePress.
Baldini, L., Martino, A., and Rizzi, A. (2019b). Towards
a class-aware information granulation for graph em-
bedding and classification. In Computational Intel-
ligence: 11th International Joint Conference, IJCCI
2019 Vienna, Austria, September 17-19, 2019 Revised
Selected Papers. To appear in.
Bargiela, A. and Pedrycz, W. (2003). Granular comput-
ing: an introduction. Kluwer Academic Publishers,
Boston.
Bargiela, A. and Pedrycz, W. (2006). The roots of granular
computing. In 2006 IEEE International Conference
on Granular Computing, pages 806–809.
Bianchi, F. M., Grattarola, D., Alippi, C., and Livi, L.
(2019). Graph neural networks with convolutional
arma filters. arXiv preprint arXiv:1901.01343.
Bianchi, F. M., Livi, L., Rizzi, A., and Sadeghian, A.
(2014a). A granular computing approach to the de-
sign of optimized graph classification systems. Soft
Computing, 18(2):393–412.
Bianchi, F. M., Scardapane, S., Livi, L., Uncini, A., and
Rizzi, A. (2014b). An interpretable graph-based im-
age classifier. In 2014 International Joint Conference
on Neural Networks (IJCNN), pages 2339–2346.
Bianchi, F. M., Scardapane, S., Rizzi, A., Uncini, A.,
and Sadeghian, A. (2016). Granular computing tech-
niques for classification and semantic characterization
of structured data. Cognitive Computation, 8(3):442–
461.
Borgwardt, K. M. and Kriegel, H. P. (2005). Shortest-path
kernels on graphs. In Fifth IEEE International Con-
ference on Data Mining (ICDM’05), pages 8 pp.–.
Bunke, H. (2003). Graph-based tools for data mining and
machine learning. In Perner, P. and Rosenfeld, A., ed-
itors, Machine Learning and Data Mining in Pattern
Recognition, pages 7–19, Berlin, Heidelberg. Springer
Berlin Heidelberg.
Chang, C.-C. and Lin, C.-J. (2011). Libsvm: A library for
support vector machines. ACM transactions on intel-
ligent systems and technology (TIST), 2(3):27.
Cinti, A., Bianchi, F. M., Martino, A., and Rizzi, A. (2020).
A novel algorithm for online inexact string matching
and its fpga implementation. Cognitive Computation,
12(2):369–387.
Cover, T. and Hart, P. (1967). Nearest neighbor pattern clas-
sification. IEEE transactions on information theory,
13(1):21–27.
Ding, S., Du, M., and Zhu, H. (2015). Survey on granularity
clustering. Cognitive neurodynamics, 9(6):561–572.
Frattale Mascioli, F. M., Rizzi, A., Panella, M., and Mar-
tinelli, G. (2000). Scale-based approach to hierarchi-
cal fuzzy clustering. Signal Processing, 80(6):1001 –
1016.
Ghosh, S., Das, N., Gonçalves, T., Quaresma, P., and
Kundu, M. (2018). The journey of graph kernels
through two decades. Computer Science Review,
27:88 – 111.
Hamilton, W., Ying, Z., and Leskovec, J. (2017). In-
ductive representation learning on large graphs. In
Advances in neural information processing systems,
pages 1024–1034.
Han, L., Hancock, E. R., and Wilson, R. C. (2011). Charac-
terizing graphs using approximate von neumann en-
tropy. In Vitrià, J., Sanches, J. M., and Hernández,
M., editors, Pattern Recognition and Image Analysis,
pages 484–491, Berlin, Heidelberg. Springer Berlin
Heidelberg.
Kipf, T. N. and Welling, M. (2016). Semi-supervised clas-
sification with graph convolutional networks. arXiv
preprint arXiv:1609.02907.
Kondor, R. I. and Lafferty, J. (2002). Diffusion kernels on
graphs and other discrete structures. In Proceedings of
the 19th international conference on machine learn-
ing, volume 2002, pages 315–322.
Leone Sciabolazza, V. and Riccetti, L. (2020). Diffusion de-
lay centrality: Decelerating diffusion processes across
networks. Available at SSRN 3653030.
Levenshtein, V. I. (1966). Binary codes capable of correct-
ing deletions, insertions, and reversals. Soviet physics
doklady, 10(8):707–710.
Li, W. (2013). Modularity embedding. In Lee, M., Hirose,
A., Hou, Z.-G., and Kil, R. M., editors, Neural Infor-
mation Processing, pages 92–99, Berlin, Heidelberg.
Springer Berlin Heidelberg.
Livi, L., Del Vescovo, G., Rizzi, A., and Frattale Mascioli,
F. M. (2014). Building pattern recognition applica-
tions with the SPARE library. CoRR, abs/1410.5263.
Livi, L. and Rizzi, A. (2013). Graph ambiguity. Fuzzy Sets
and Systems, 221:24–47.
Martino, A., De Santis, E., Giuliani, A., and Rizzi, A.
(2020a). Modelling and recognition of protein contact
networks by multiple kernel learning and dissimilarity
representations. Entropy, 22(7).
Martino, A., Giuliani, A., and Rizzi, A. (2018a). Gran-
ular computing techniques for bioinformatics pat-
tern recognition problems in non-metric spaces. In
Pedrycz, W. and Chen, S.-M., editors, Computational
Intelligence for Pattern Recognition, pages 53–81.
Springer International Publishing, Cham.
Martino, A., Giuliani, A., and Rizzi, A. (2019a). (hy-
per)graph embedding and classification via simplicial
complexes. Algorithms, 12(11).
Martino, A., Giuliani, A., Todde, V., Bizzarri, M., and
Rizzi, A. (2020b). Metabolic networks classification
NCTA 2020 - 12th International Conference on Neural Computation Theory and Applications
348