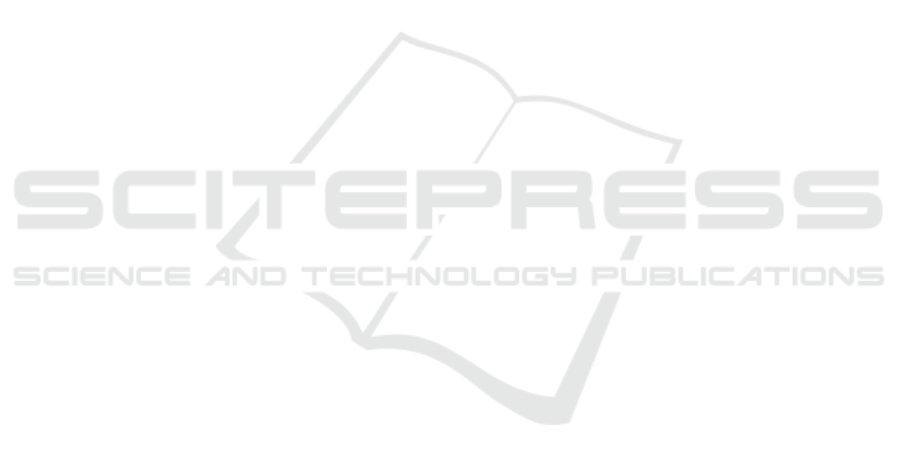
Distributed Computing and Networking, ICDCN ’16,
pages 47:1–47:5, New York, NY, USA. ACM.
Bhuyan, M. H., Bhattacharyya, D. K., and Kalita, J. K.
(2014). Network anomaly detection: Methods, sys-
tems and tools. IEEE Communications Surveys Tuto-
rials, 16(1):303–336.
Cover, T. and Hart, P. (1967). Nearest neighbor pattern clas-
sification. IEEE transactions on information theory,
13(1):21–27.
El-Khatib, K. (2010). Impact of Feature Reduction on the
Efficiency of Wireless Intrusion Detection Systems.
IEEE Transactions on Parallel and Distributed Sys-
tems, 21(8):1143–1149.
Frank, E., Hall, M. A., and Witten, I. H. (2016). Data min-
ing: practical machine learning tools and techniques.
Morgan Kaufmann, 4 edition.
Goldberg, D. E. (1989). Genetic Algorithms in Search, Op-
timization and Machine Learning. Addison-Wesley
Longman Publishing Co., Inc., Boston, MA, USA, 1st
edition.
Guennoun, M., Lbekkouri, A., and El-Khatib, K. (2008).
Selecting the Best Set of Features for Efficient In-
trusion Detection in 802.11 Networks. In Informa-
tion and Communication Technologies: From Theory
to Applications, 2008. ICTTA 2008. 3rd International
Conference on, pages 1–4.
IEEE (2016). Ieee standard for information technol-
ogy—telecommunications and information exchange
between systems local and metropolitan area net-
works—specific requirements - part 11: Wireless lan
medium access control (mac) and physical layer (phy)
specifications. IEEE Std 802.11-2016 (Revision of
IEEE Std 802.11-2012), pages 1–3534.
Kolias, C., Kambourakis, G., Stavrou, A., and Gritzalis, S.
(2016). Intrusion detection in 802.11 networks: Em-
pirical evaluation of threats and a public dataset. IEEE
Communications Surveys Tutorials, 18(1):184–208.
Kolias, C., Kolias, V., and Kambourakis, G. (2017). Ter-
mid: A distributed swarm intelligence-based approach
for wireless intrusion detection. Int. J. Inf. Secur.,
16(4):401–416.
Lanze, F., Panchenko, A., Braatz, B., and Engel, T. (2014).
Letting the Puss in Boots Sweat: Detecting Fake Ac-
cess Points Using Dependency of Clock Skews on
Temperature. In Proceedings of the 9th ACM Sympo-
sium on Information, Computer and Communications
Security, ASIA CCS ’14, pages 3–14.
Martino, A., Frattale Mascioli, F. M., and Rizzi, A. (2020a).
On the optimization of embedding spaces via infor-
mation granulation for pattern recognition. In 2020
International Joint Conference on Neural Networks
(IJCNN). Accepted for Publication.
Martino, A., Giuliani, A., and Rizzi, A. (2019a). (hy-
per)graph embedding and classification via simplicial
complexes. Algorithms, 12(11).
Martino, A., Giuliani, A., Todde, V., Bizzarri, M., and
Rizzi, A. (2020b). Metabolic networks classification
and knowledge discovery by information granulation.
Computational Biology and Chemistry, 84:107187.
Martino, A., Rizzi, A., and Frattale Mascioli, F. M. (2017).
Efficient approaches for solving the large-scale k-
medoids problem. In Proceedings of the 9th Inter-
national Joint Conference on Computational Intelli-
gence - Volume 1: IJCCI,, pages 338–347. INSTICC,
SciTePress.
Martino, A., Rizzi, A., and Frattale Mascioli, F. M.
(2019b). Efficient approaches for solving the large-
scale k-medoids problem: Towards structured data.
In Sabourin, C., Merelo, J. J., Madani, K., and War-
wick, K., editors, Computational Intelligence: 9th In-
ternational Joint Conference, IJCCI 2017 Funchal-
Madeira, Portugal, November 1-3, 2017 Revised Se-
lected Papers, pages 199–219. Springer International
Publishing, Cham.
Powers, D. M. W. (2011). Evaluation: From precision, re-
call and f-measure to roc., informedness, markedness
& correlation. Journal of Machine Learning Tech-
nologies, 2(1):37–63.
Qin, Y., Li, B., Yang, M., and Yan, Z. (2018). Attack detec-
tion for wireless enterprise network: a machine learn-
ing approach. In 2018 IEEE International Conference
on Signal Processing, Communications and Comput-
ing (ICSPCC), pages 1–6.
Rizzi, A., Granato, G., and Baiocchi, A. (2020). Frame-by-
frame wi-fi attack detection algorithm with scalable
and modular machine-learning design. Applied Soft
Computing, 91:106188.
Roux, J., Alata, E., Auriol, G., Ka
ˆ
aniche, M., Nicomette,
V., and Cayre, R. (2018). Radiot: Radio communi-
cations intrusion detection for iot - a protocol inde-
pendent approach. In 2018 IEEE 17th International
Symposium on Network Computing and Applications
(NCA), pages 1–8.
Sethuraman, S. C., Dhamodaran, S., and Vijayakumar, V.
(2019). Intrusion detection system for detecting wire-
less attacks in ieee 802.11 networks. IET Networks,
8(4):219–232.
Sperotto, A., Schaffrath, G., Sadre, R., Morariu, C., Pras,
A., and Stiller, B. (2010). An Overview of IP Flow-
Based Intrusion Detection. IEEE Communications
Surveys Tutorials, 12(3):343–356.
Takahashi, D., Xiao, Y., Zhang, Y., Chatzimisios, P., and
Chen, H.-H. (2010). IEEE 802.11 User Fingerprinting
and Its Applications for Intrusion Detection. Comput.
Math. Appl., 60(2):307–318.
Theodoridis, S. and Koutroumbas, K. (2008). Pattern
Recognition. Academic Press, 4 edition.
Thing, V. L. L. (2017). IEEE 802.11 Network Anomaly
Detection and Attack Classification: A Deep Learning
Approach. In 2017 IEEE Wireless Communications
and Networking Conference (WCNC), pages 1–6.
Youden, W. J. (1950). Index for rating diagnostic tests. Can-
cer, 3(1):32–35.
NCTA 2020 - 12th International Conference on Neural Computation Theory and Applications
422