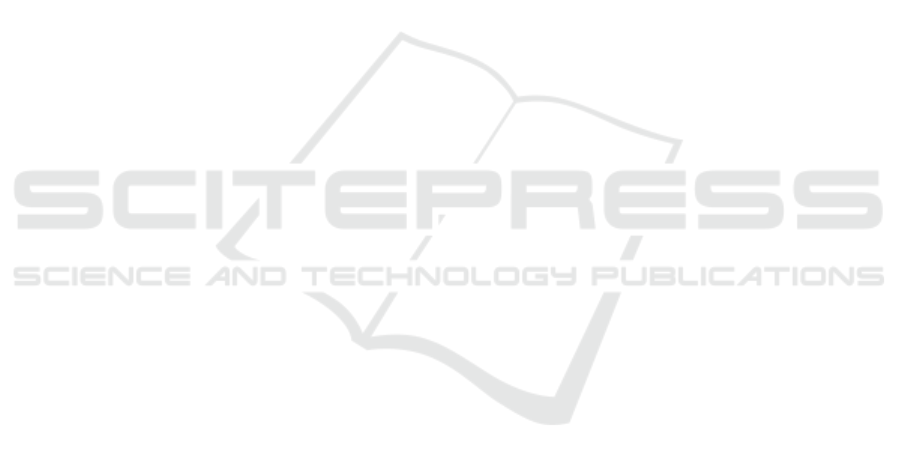
validated with literary sentences, taken from specially
structured, literary corpora.
Our results show that this protocol is well suited
to the task of detection and classification of emotions
in literary manuscripts.
Literary works in various forms, such as para-
graphs, verses, sentences, or phrases, are difficult to
evaluate automatically, because they have a rich ex-
pressive diversity and present a high complexity in the
employment of language. Moreover, there is the issue
of multi-emotional sentences, with possible ambigu-
ity in meaning, that adds to the complexity of the task.
However, we have shown that fuzzy logic provides an
interesting and efficient approach to the problem of
analysing these types of corpora.
In its current version, FLE has been designed to
treat only text written in Spanish. However, the proto-
col is modular and simple to adapt to languages other
than Spanish. In particular, it should be possible to
adapt our FLE implementation to other romance lan-
guages, such as French, Portuguese, Italian, Catalan,
among others, with a reasonable amount of effort.
We plan to improve our model through the manip-
ulation of the linguistic values or by combining other
membership functions. We also consider the possibil-
ity of evaluating our protocol on artificial sentences,
generated by Natural Language Generation (NLG) al-
gorithms (Moreno-Jim
´
enez et al., 2020; Ke and Xiao-
jun, 2018).
ACKNOWLEDGEMENTS
This work is funded by Consejo Nacional de
Ciencia y Tecnolog
´
ıa (Conacyt, Mexico), grant
number 661101 and partially by the Univer-
sit
´
e d’Avignon/Laboratoire Informatique d’Avignon
(LIA), France.
REFERENCES
Arguedas, M., Xhafa, F., Casillas, L., Daradoumis, T., Pe
˜
na,
A., and Caball
´
e, S. (2018). A model for providing
emotion awareness and feedback using fuzzy logic in
online learning. In Soft Comput, volume 22, pages
963–977.
Cambria, E. (2016). Affective computing and sentiment
analysis. IEEE Intelligent Systems, 31(2):102–107.
Clark, E., Ji, Y., and Smith, N. A. (2018). Neural text gen-
eration in stories using entity representations as con-
text. In NAACL-HLT, volume 1, pages 2250–2260,
New Orleans, Louisiana. ACL.
Dragoni, M., Tettamanzi, A. G. B., and da Costa Pereira,
C. (2015). Propagating and aggregating fuzzy polar-
ities for concept-level sentiment analysis. Cognitive
Computation, 7:186–197.
Howells, K. and Ertugan, A. (2017). Applying fuzzy logic
for sentiment analysis of social media network data in
marketing. In 9th International Conference on Theory
and Application of Soft Computing, Computing with
Words and Perception, volume 120, pages 664–670.
Elsevier.
Indhuja, K. and Reghu, R. P. C. (2014). Fuzzy logic based
dentiment snalysis of product review documents. In
1st ICCSC, pages 18–22, Trivandrum, India. IEEE.
Iria, d. C., Cabr
´
e, M. T., SanJuan, E., Sierra, G., Torres-
Moreno, J., and Vivaldi, J. (2011). Automatic special-
ized vs. non-specialized sentence differentiation. In
CICLing 2011, pages 266–276, Tokyo, Japan.
Jones, K. S. (1972). A statistical interpretation of term
specificity and its application in retrieval. Journal of
Documentation, 28:11–21.
Ke, W. and Xiaojun, W. (2018). SentiGAN: Generating
sentimental texts via mixture adversarial networks.
In Proceedings of the Twenty-Seventh International
Joint Conference on Artificial Intelligence (IJCAI-18),
pages 4446–4452, Stockholm, Sweden. IJCAI-ECAI,
AAAI Press.
Matiko, J. W., Beeby, S. P., and Tudor, J. (2014). Fuzzy
logic based emotion classification. In 2014 IEEE In-
ternational Conference on Acoustics, Speech and Sig-
nal Processing (ICASSP), pages 4389–4393. IEEE.
Moreno-Jim
´
enez, L. G., Torres-Moreno, J. M., Wedemann,
R. S., and SanJuan, E. (2020). Generaci
´
on autom
´
atica
de frases literarias. Linguam
´
atica, 12(1):15–30.
Padr
´
o, L. and Stanilovsky, E. (2012). FreeLing 3.0: To-
wards wider multilinguality. In 8th International
Conference on Language Resources and Evaluation
(LREC 2012), pages 2473–2479, Istanbul, Turkey.
ELRA.
Pang, B. and Lee, L. (2008). Opinion mining and sentiment
analysis. Foundations and Trends
R
in Information
Retrieval, 2(1–2):1–135.
Siddiqui, M., Wedemann, R. S., and Jensen, H. J. (2018).
Avalanches and generalized memory associativity in a
network model for conscious and unconscious mental
functioning. Physica A, 490:127–138.
Tashtoush, Y. M. and Al Aziz Orabi, D. A. (2019). Tweets
emotion prediction by using fuzzy logic system. In 6th
International Conference on Social Networks Analy-
sis, Management and Security (SNAMS), pages 83–90.
IEEE.
Torres-Moreno, J.-M. (2012). Beyond stemming and
lemmatization: Ultra-stemming to improve automatic
text summarization. CoRR, abs/1209.3126.
Torres-Moreno, J.-M. and Moreno-Jim
´
enez, L.-G. (2020).
LiSSS: A toy corpus of literary Spanish sentences sen-
timent for emotions detection. arXiv, 2005.08223v1.
Vashishtha, S. and Susan, S. (2020). Inferring sentiments
from supervised classification of text and speech
cues using fuzzy rules. Procedia Computer Science,
167:1370 – 1379.
Wedemann, R. S. and Plastino, A. R. (2016). F
´
ısica es-
tad
´
ıstica, redes neuronales y Freud. Revista N
´
ucleos,
3:4–10.
Zadeh, L. (1965). Fuzzy sets. Information and Control,
8(3):338 – 353.
FLE: A Fuzzy Logic Algorithm for Classification of Emotions in Literary Corpora
209