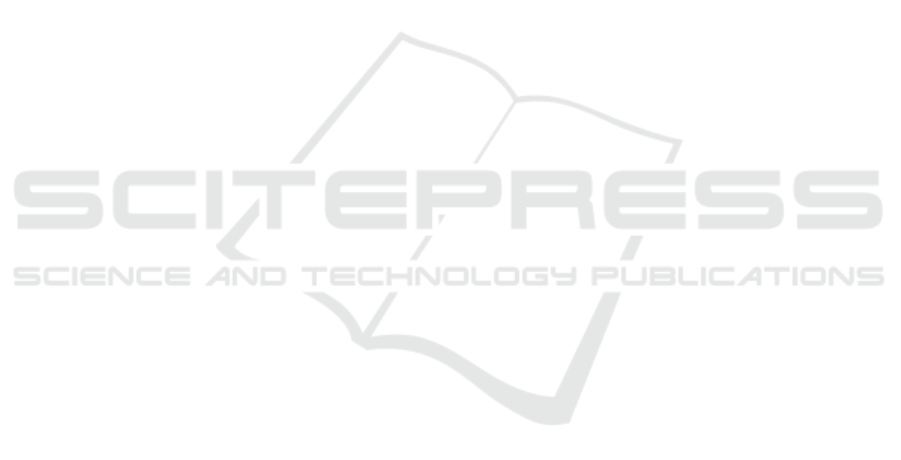
more clusters with one collection each.
6 CONCLUSIONS AND FUTURE
WORK
A good practice on metadata descriptions consists of
using specific values to identify discrete entities. This
is the same approach that leads to the Linked Open
Data, when specifying entities from other, open, col-
lections. Additionally, quite often these entities are
much fewer than the collection items (e.g. the num-
ber of languages of the items, or their type), leading
to many value repetitions. This occurs more on some
Dublin Core elements (like language and type), than
on some others (like identifier and date). But it does
not occur as often as we would expect: Quite often,
there are many values corresponding to the same en-
tity.
We started by studying the language element.
Even though the language specification information
is handled in standard ways in many computer appli-
cations, on many harvested metadata the Dublin Core
element language lacks any standardization.
We examined the language values found and their
frequencies. In many cases we found illegal or prob-
lematic values and we classified them into categories.
We used dendrograms to show the similarity of the
language values among collections.
Nevertheless, there are more common understand-
ing (and also standards) on what the language entities
are and how to denote them. And still, many items
do not adopt the same values, and provide many “un-
usual” values.
We repeated the procedure for the type, format,
relation, coverage and publisher elements. The sit-
uation on all these elements was similar. We could
observe the value clustering differences by using den-
drograms.
We conclude that we need more standardization
on values, more so on language values, so that state-
ments across collections follow some good practices.
The situation is similar to the other Dublin Core ele-
ments, although not identical.
To collect all possible records, we adapted the har-
vested procedure to handle both reasonable timeouts
and large number or records, using a repeated harvest-
ing procedure for many small timeout intervals.
In the future, we could study unique and low rep-
etition values that are similar to other values, on el-
ements with high usual repetition and also repeated
values on elements with low usual repetition in order
to derive rules and guidelines for automatically creat-
ing value mappings.
REFERENCES
Baca, M. (2003). Practical issues in applying metadata
schemas and controlled vocabularies to cultural her-
itage information. Cataloging & Classification Quar-
terly, 36(3–4):47–55.
Fuhr, N., Tsakonas, G., Aalberg, T., Agosti, M., Hansen,
P., Kapidakis, S., Klas, C.-P., Kov
´
acs, L., Landoni,
M., Micsik, A., Papatheodorou, C., Peters, C., and
Sølvberg, I. (2007). Evaluation of digital libraries. In-
ternational Journal on Digital Libraries, 8(1):21–38.
Harper, C. A. (2016). Metadata analytics, visualization, and
optimization: Experiments in statistical analysis of the
digital public library of america (dpla). Code4Lib, 33.
Harper, C. A. and Tillett, B. B. (2007). Library of congress
controlled vocabularies and their application to the se-
mantic web. Cataloging & Classification Quarterly,
43(3–4):47–68.
Hughes, B. (2005). Metadata quality evaluation: experience
from the open language archives community. Lecture
Notes in Computer Science, 3334.
Kapidakis, S. (2018). Metadata Synthesis and Updates on
Collections Harvested using the Open Archive Initia-
tive Protocol for Metadata Harvesting. 22nd Interna-
tional Conference on Theory and Practice of Digital
Libraries, TPDL 2018, LNCS 10450, Springer, pages
16–31.
Kapidakis, S. (2019). Repeated values on Collections
Harvested using the Open Archive Initiative Protocol
for Metadata Harvesting. 11th International Confer-
ence on Management of Digital EcoSystems, MEDES
2019, November 12–14, 2019, Limassol, Cyprus,
ACM 2019, ISBN 978-1-4503-6238-2.
Kir
´
aly, P., Stiller, J., Charles, V., Bailer, W., and Freire, N.
(2019). Evaluating data quality in europeana: Met-
rics for multilinguality. In Garoufallou, E., Sartori,
F., Siatri, R., and Zervas, M., editors, Metadata and
Semantic Research, pages 199–211, Cham. Springer
International Publishing.
Maltese, V. (2018). Digital transformation challenges for
universities: Ensuring information consistency across
digital services. Cataloging & Classification Quar-
terly, 56(7):592–606.
Moreira, B. L., Gonc¸alves, M. A., Laender, A. H., and Fox,
E. A. (2009). Automatic evaluation of digital libraries
with 5squal. Journal of Informetrics, 3(2):102–123.
Vullo, G., Clavel, G., Ferro, N., Higgins, S., van Horik, R.,
Horstmann, W., and Kapidakis, S. (2010). : Quality
interoperability within digital libraries: the DL. org
perspective. In: 2nd DL. org Workshop in conjunction
with ECDL, 2010:9–10.
Wilkinson, M. D. e. a. (2016). The fair guiding principles
for scientific data management and stewardship. Sci.
Data, 3(160018).
Zhang, Y. (2010). Developing a holistic model for digital
library evaluation. Journal of the American Society for
Information Science and Technology, 61(1):88–110.
KEOD 2020 - 12th International Conference on Knowledge Engineering and Ontology Development
188