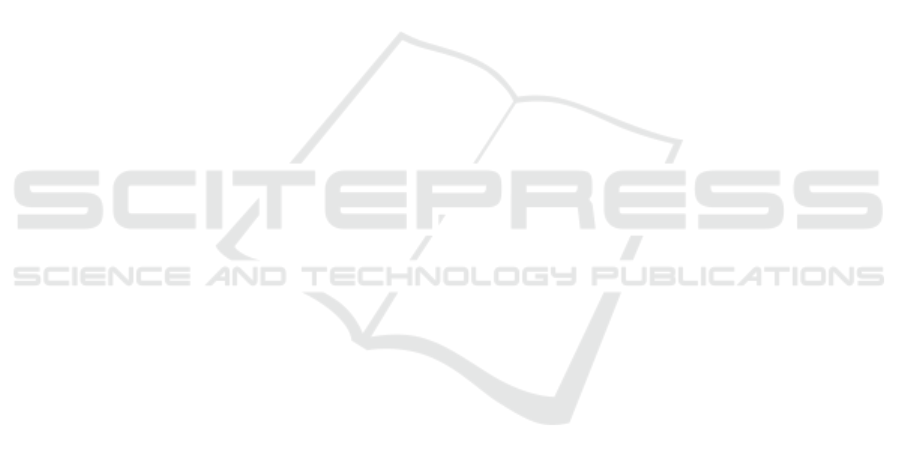
sibility of improving the informative value of text,
through sourcing additional information from social
forums. Additionally, we look to determine if Red-
dit or Twitter is a superior source of information for
augmenting information snippets. In this paper, using
extracts from an article regarding a recent news event,
we compare Reddit and Twitter as alternative sources
of auxiliary information and measure the relevance of
comments found there to a set of users. Text simi-
larity is applied to a body of text and a selection of
microblogs to link comments from Reddit and tweets
from Twitter with paragraphs written on a particular
topic. Subsequently, a group survey is performed to
evaluate the results; a statistical analysis is then ap-
plied on the user feedback to determine which were
significant. Our conclusions show that relevant infor-
mation can be identified which improves the dataset;
additionally, the results show that Twitter proved to be
a more appropriate source. Included will be a number
of explanations as to why this might be the case.
2 RELATED WORK
Hsu et al. (Hsu et al., 2009) present a study which
aims to rank comments found on articles in Digg (a
popular online opinion editorial). They apply logistic
regression using a selection of metadata found relat-
ing to the comments and the social connectedness of
the comment makers. Interestingly, they found that
visibility is one of the most influential factors in how
a comment is rated. Visibility is affected by how
quickly one responds to an article. Visibility can be
influenced by a number of factors external to the ac-
tual content, such as time of posting or thread bias
(Hsu et al., 2009). By focusing on the text alone, one
can then promote content that is relevant, rather than
content that is dependent on up-votes or retweets to
gain visibility.
Shmueli et al. (Shmueli et al., 2012) use co-
commenting and textual tags to implement a collab-
orative filtering model which recommends news ar-
ticles to users. Past comments and social connect-
edness are used as indicators for recommendations.
Others who have investigated this approach include
work by Li (Li et al., 2010) and Bach (Bach et al.,
2016). Such an approach lends itself well for iden-
tifying related content of interest, though it does not
address other issues such as problems associated with
the echo-chamber.
Another approach proposed in the literature clus-
ters trending tweets together and extracts common
terms (Rehem et al., 2016). These terms are used to
form a query which the authors use to enter into a
search engine. They extract the most related news ar-
ticles to the query and recommend them to the user.
As a recommender system this approach is not very
personalised (as no input is made of user tastes), it is
based on the assumption that popular news is of inter-
est to the user. It does however add a level of serendip-
ity and helps to combat negative effects such as the
filter bubble by not over-fitting to the user’s tastes.
A similar approach to the one outlined in this pa-
per, is presented by Aker et al,. (Aker et al., 2016)
who aim to link comments to articles. They linked
comments to articles at a sentence level, where the ar-
ticle is first reduced to sentences and each sentence is
then compared to the related comments. Their dataset
was constructed using articles published online in the
Guardian newspaper website between the months of
June-July 2014. They amassed a total of 3,362 arti-
cles with an average of 425.95 comments per article.
In addition to linking comments and sentences, they
perform sentiment analysis, to determine whether the
comments agreed or disagreed with a given article.
As distance metrics they employed Jaccard and Co-
sine distance. As well as using syntactical similar-
ities they analysed connections using distributional
similarities between terms. Distributional similarity
is the idea that words that co-occur regularly have a
similarity of meaning. Using two additional sources
(BNC corpora and Wikipedia) they constructed sim-
ilarity vectors and incorporated those into their sys-
tem. They include on average about 425 comments
per article. One limitation of their approach is that
they rely on manual labelling to determine polarity
in the debate, which is an acceptable early first step.
Ultimately, a functional system would have to iden-
tify indicators automatically for any degree of prac-
tical application. Bias polarity could potentially be
inferred from the sources used in this experiment, by
determining poster demographic in a given subreddit,
or through looking at additional tweets from the indi-
viduals who retweet a given text.
Research by Becker (Becker et al., 2010) utilises
comments found in flickr to identify events, as deter-
mined from observing active conversation found on
social media. The authors identify distinctions be-
tween event detection in social media with event de-
tection in more standard datasets; namely, that there
is less structure and more noise in social media data.
They cluster comments into related groups and from
the resulting clusters deduce if a particular event is
happening. Similarly, a lot of work in this field
focuses on summarising user comments rather than
mapping them to points made in any correspond-
ing news article (Ma et al., 2012) (Hu et al., 2008)
(Khabiri et al., 2011).
KDIR 2020 - 12th International Conference on Knowledge Discovery and Information Retrieval
278