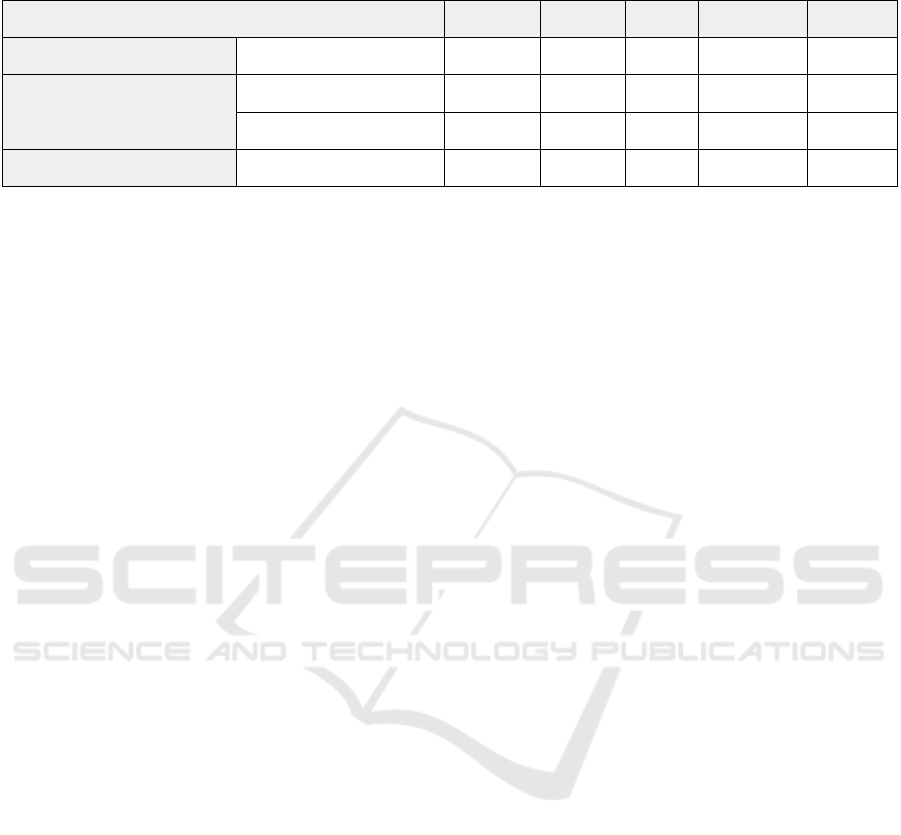
Table 1: Summary of the results obtained (TP, FP, FN, PPV in % and Se in %) using the proposed algorithm in the considered
databases. For benchmarking we used Pth = 3 & N = 5 as parameters of the algorithm.
Database Sampling Rate (Hz) TP FP FN PPV (%) Se (%)
Challenge 2014 120-1000 71529 332 884 98.88 99.52
ST-T Database (ltstdb) 250 782483 13712 8082 98.15 98.79
MIT-BIH (mitdb) 360 106590 1758 2904 98.35 97.62
MIT-BIH (nstdb) 360 23957 2907 1633 96.23 98.72
QRS detector methods under highly dynamically ac-
tivities, as a result of the shortage of publicly available
ECG databases with data acquired in such conditions.
Furthermore, the confidentiality of the implemented
methods in the common commercialized wearable de-
vices raises concerns with respect to the R-peaks de-
tection reliability. This paper explores the use case
limitations of the current approaches, and validates
an alternative approach that can be used for R-peak
detection.
ACKNOWLEDGEMENTS
The authors would like to express their gratitude
to Joana Santos, cardiopneumologist, for the man-
ual verification and annotation of the electrocar-
diograms R-peaks. We would also like to thank
the embedded software engineer at ASI, Sirisack
Samoutphonh, for the support with the implementa-
tion of the proposed method in the embedded sysmte.
This work has been partially funded by Instituto de
Telecomunicac¸
˜
oes (IT) via FCT/MCTES through na-
tional funds and when applicable co-funded EU funds
under the project UIDB/50008/2020.
REFERENCES
Acharya, U. R., Krishnan, S. M., Spaan, J. A., and Suri,
J. S. (2007). Advances in cardiac signal processing.
Springer.
Buchheit, M. (2014). Monitoring training status with HR
measures: Do all roads lead to Rome? Frontiers in
Physiology, 5(23):1–20.
Christov, I. I. (2004). Real time electrocardiogram QRS de-
tection using combined adaptive threshold. BioMedi-
cal Engineering Online, 3(28):1–9.
Elgendi, M., Eskofier, B., Dokos, S., and Abbott, D. (2014).
Revisiting QRS detection methodologies for portable,
wearable, battery-operated, and wireless ECG sys-
tems. PloS one, 9(1).
FieldWiz (2020). FieldWiz device, Advanced Sports Instru-
ments - ASI.
Francis, J. (2016). ECG monitoring leads and special
leads. Indian Pacing and Electrophysiology Journal,
16(3):92–95.
Goldberger, A. L., Amaral, L. A., Glass, L., Hausdorff,
J. M., Ivanov, P. C., Mark, R. G., Mietus, J. E., Moody,
G. B., Peng, C.-K., and Stanley, H. E. (2000). Phys-
ioBank, PhysioToolkit, and PhysioNet: components
of a new research resource for complex physiologic
signals. Circulation, 101(23):e215–e220.
Guti
´
errez-Rivas, R., Garc
´
ıa, J. J., Marnane, W. P.,
and Hern
´
andez, A. (2015). Novel real-time low-
complexity QRS complex detector based on adaptive
thresholding. IEEE Sensors Journal, 15(10):6036–
6043.
Hamilton, P. (2002). Open source ECG analysis. Computers
in Cardiology, 29:101–104.
Javaloyes, A., Sarabia, J. M., Lamberts, R. P., and Moya-
Ramon, M. (2019). Training prescription guided by
heart-rate variability in cycling. International Journal
of Sports Physiology and Performance, 14(1):23–32.
Kalidas, V. and Tamil, L. (2017). Real-time QRS de-
tector using stationary wavelet transform for auto-
mated ECG analysis. In Proc. of the IEEE Int’l Conf.
on Bioinformatics and Bioengineering (BIBE), pages
457–461.
Laborde, S., Br
¨
ull, A., Weber, J., and Anders, L. S. (2011).
Trait emotional intelligence in sports: A protective
role against stress through heart rate variability? Per-
sonality and Individual Differences, 51(1):23–27.
Lane, R. D., McRae, K., Reiman, E. M., Chen, K., Ah-
ern, G. L., and Thayer, J. F. (2009). Neural correlates
of heart rate variability during emotion. Neuroimage,
44(1):213–222.
Liu, F., Liu, C., Jiang, X., Zhang, Z., Zhang, Y., Li, J., and
Wei, S. Performance analysis of ten common QRS
detectors on different ECG application cases. Journal
of Healthcare Engineering, 2018.
Lourenc¸o, A., da Silva, H. P., Lourenc¸o, R., Leite, P., and
Fred, A. (2012). Real time electrocardiogram segmen-
tation for finger based ECG biometrics. In Proc. Int’l
Conf. on Bio-inspired Systems and Signal Processing
(BIOSIGNALS), pages 49–54.
Lourenc¸o, A., Pl
´
acido da Silva, H., Carreiras, C., Priscila
Alves, A., and Fred, A. L. N. (2014). A web-based
platform for biosignal visualization and annotation.
Multimedia Tools and Applications, 70:433–460.
Lourenc¸o, A., Silva, H., and Fred, A. (2012). ECG-based
biometrics: A real time classification approach. In
KDIR 2020 - 12th International Conference on Knowledge Discovery and Information Retrieval
308