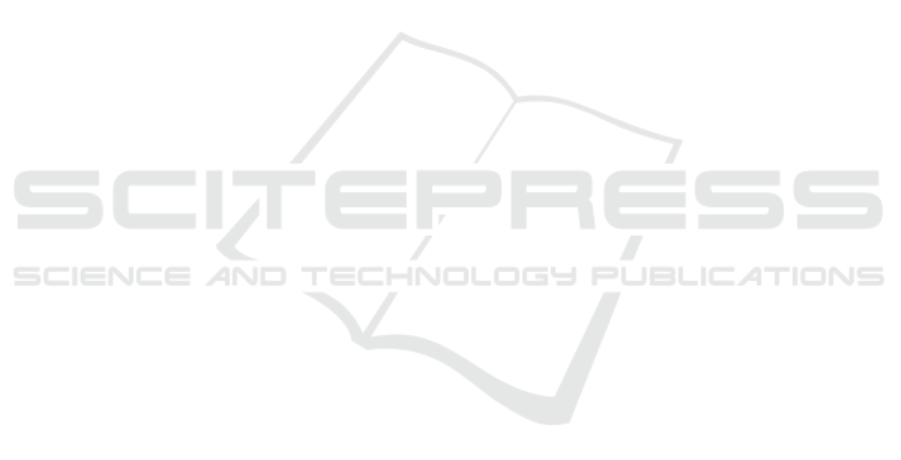
Example 6
Question: What did a British study of the way women
search for medical information online indicate? (SAT
practice test 2 article 3)
Correct answer: An experienced Internet user can,
at least in some cases, assess the trustworthiness and
probable value of a Web page in a matter of seconds.
Distractors:
1. An experienced Supernet user can, at least in
some cases, assess the trustworthiness and prob-
able value of a Web page in a matter of seconds.
2. An experienced CogNet user can, at least in some
cases, assess the trustworthiness and probable
value of a Web page in a matter of seconds.
3. An inexperienced Internet user can, at least in
some cases, assess the trustworthiness and prob-
able value of a Web page in a matter of seconds.
Example 7
What does a woman know better than a man? (SAT
test 2 article 4)
Correct answer: the cost of life.
Distractors:
1. the cost of happiness.
2. the cost of experience.
3. the risk of life.
Example 8
This example presents a distractor without sufficient
distraction.
Question: What are subject to egocentrism, social
projection, and multiple attribution errors?
Correct answer: their insights.
Distractors:
1. their perspectives.
2. their findings.
3. their valuables.
The last distractor can be spotted wrong by just look-
ing at the question: It is easy to tell that it is out of
place without the need to read the article.
5 CONCLUSIONS AND FINAL
REMARKS
We presented a novel method using various NLP tools
for generating adequate distractors for a QAP to form
an adequate MCQ on a given article. This is an in-
teresting area with important applications. Experi-
ments and evaluations on MCQs generated from the
SAT practice reading tests indicate that our approach
is promising.
A number of improvements can be explored. For
example, we may improve the ranking measure to
help select a better distractor for a target word from a
list of candidates. Another direction is explore how to
produce generative distractors using neural networks,
instead of just replacing a few target words in a given
answer.
ACKNOWLEDGMENT
This work was supported in part by funding from Eola
Solutions, Inc. We thank Hao Zhang and Changfeng
Yu for discussions.
REFERENCES
Ali, H., Chali, Y., and Hasan, S. A. (2010). Automation of
question generation from sentences. In Proceedings
of QG2010: The Third Workshop on Question Gener-
ation, pages 58–67.
Bojanowski, P., Grave, E., Joulin, A., and Mikolov, T.
(2017). Enriching word vectors with subword infor-
mation. Transactions of the Association for Computa-
tional Linguistics, 5:135–146.
Correia, R., Baptista, J., Eskenazi, M., and Mamede, N.
(2012). Automatic generation of cloze question stems.
In Caseli, H., Villavicencio, A., Teixeira, A., and
Perdig
˜
ao, F., editors, Computational Processing of the
Portuguese Language, pages 168–178, Berlin, Heidel-
berg. Springer Berlin Heidelberg.
Jiang, S. and Lee, J. (2017). Distractor generation for chi-
nese fill-in-the-blank items. In BEA@EMNLP.
Levenshtein, V. I. (1966). Binary Codes Capable of Cor-
recting Deletions, Insertions and Reversals. Soviet
Physics Doklady, 10:707.
Martha, P., Dan, G., and Paul, K. (2005). The proposition
bank: a corpus annotated with semantic roles. Com-
putational Linguistics Journal, 31(1):10–1162.
Meng1, Y., Huang, J., Wang, G., Zhang, C., Zhuang, H.,
Kaplan, L., and Han1, J. (2019). Spherical text em-
bedding. In 33rd Conference on Neural Information
Processing Systems (NeurIPS 2019).
Mikolov, T., Grave, E., Bojanowski, P., Puhrsch, C., and
Joulin, A. (2018). Advances in pre-training distributed
word representations. In Proceedings of the Interna-
tional Conference on Language Resources and Evalu-
ation (LREC 2018).
Mikolov, T., Sutskever, I., Chen, K., Corrado, G., and Dean,
J. (2013). Distributed representations of words and
phrases and their compositionality. In Proceedings of
KDIR 2020 - 12th International Conference on Knowledge Discovery and Information Retrieval
314