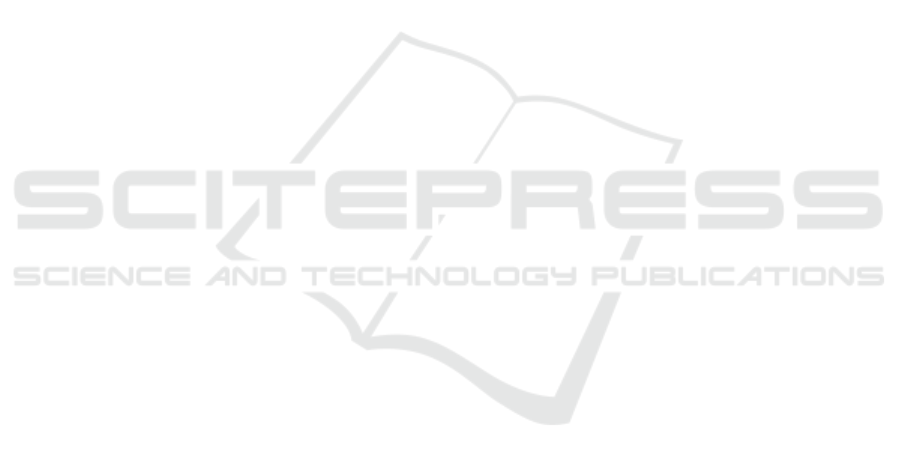
The work is extendable in several directions, such
as the embedding of the KG learning process within
neural networks, the semantic enrichment through
classifier systems for relation augmentation, and the
use of probabilistic reasoning. We also plan to carry
out a comprehensive validation of our contribution.
REFERENCES
Allen, C., Balazevic, I., and Hospedales, T. M. (2019). On
understanding knowledge graph representation. arXiv
preprint arXiv:1909.11611.
Asim, M. N., Wasim, M., Khan, M. U. G., et al. (2018).
A survey of ontology learning techniques and appli-
cations. Database, 2018.
Bizer, C., Lehmann, J., Kobilarov, G., et al. (2009). DB-
pedia - A crystallization point for the Web of Data.
Journal of web semantics, 7(3):154–165.
Bollacker, K., Evans, C., Paritosh, P., et al. (2008). Free-
base: a collaboratively created graph database for
structuring human knowledge. In Proceedings of
the 2008 ACM SIGMOD international conference on
Management of data, pages 1247–1250.
Bosselut, A., Rashkin, H., Sap, M., et al. (2019). Comet:
Commonsense transformers for automatic knowledge
graph construction. arXiv preprint arXiv:1906.05317.
Buitelaar, P., Cimiano, P., and Magnini, B. (2005). Ontology
learning from text: methods, evaluation and applica-
tions, volume 123. IOS press.
Carbonaro, A. (2012). Interlinking e-learning resources
and the web of data for improving student experience.
Journal of e-Learning and Knowledge Society, 8(2).
Carbonaro, A., Piccinini, F., and Reda, R. (2018). Integrat-
ing heterogeneous data of healthcare devices to enable
domain data management. Journal of e-Learning and
Knowledge Society.
Domeniconi, G., Masseroli, M., Moro, G., and Pinoli, P.
(2014a). Discovering new gene functionalities from
random perturbations of known gene ontological an-
notations. In KDIR 2014 - Proceedings of the In-
ternational Conference on Knowledge Discovery and
Information Retrieval, Rome, Italy, pages 107–116.
SciTePress.
Domeniconi, G., Masseroli, M., Moro, G., and Pinoli, P.
(2016a). Cross-organism learning method to discover
new gene functionalities. Computer Methods and Pro-
grams in Biomedicine, 126:20–34.
Domeniconi, G., Moro, G., Pagliarani, A., and Pasolini, R.
(2017). On deep learning in cross-domain sentiment
classification. In Proceedings of the 9th International
Joint Conference on Knowledge Discovery, Knowl-
edge Engineering and Knowledge Management - (Vol-
ume 1), Funchal, Madeira, Portugal, 2017, pages 50–
60. SciTePress.
Domeniconi, G., Moro, G., Pasolini, R., and Sartori, C.
(2014b). Cross-domain text classification through it-
erative refining of target categories representations. In
Fred, A. L. N. and Filipe, J., editors, KDIR 2014 - Pro-
ceedings of the International Conference on Knowl-
edge Discovery and Information Retrieval, Rome,
Italy, 21 - 24 October, 2014, pages 31–42. SciTePress.
Domeniconi, G., Moro, G., Pasolini, R., and Sartori, C.
(2014c). Iterative refining of category profiles for
nearest centroid cross-domain text classification. In
International Joint Conference on Knowledge Discov-
ery, Knowledge Engineering, and Knowledge Man-
agement, pages 50–67. Springer.
Domeniconi, G., Moro, G., Pasolini, R., and Sartori, C.
(2015). A Study on Term Weighting for Text Cate-
gorization: A Novel Supervised Variant of tf. idf. In
DATA, pages 26–37.
Domeniconi, G., Semertzidis, K., Lopez, V., Daly, E. M.,
Kotoulas, S., et al. (2016b). A novel method for
unsupervised and supervised conversational message
thread detection. In DATA, pages 43–54.
Ehrlinger, L. and W
¨
oß, W. (2016). Towards a Definition
of Knowledge Graphs. SEMANTiCS (Posters, Demos,
SuCCESS), 48.
Frisoni, G., Moro., G., and Carbonaro., A. (2020). Learn-
ing interpretable and statistically significant knowl-
edge from unlabeled corpora of social text messages:
A novel methodology of descriptive text mining. In
Proceedings of the 9th International Conference on
Data Science, Technology and Applications - Volume
1: DATA,, pages 121–132. INSTICC, SciTePress.
Gangemi, A. (2013). A comparison of knowledge extrac-
tion tools for the semantic web. In Cimiano, P., Cor-
cho, O., et al., editors, The Semantic Web: Seman-
tics and Big Data, pages 351–366, Berlin, Heidelberg.
Springer Berlin Heidelberg.
Hofmann, T. (2013). Probabilistic latent semantic analysis.
arXiv preprint arXiv:1301.6705.
Landauer, T. K. and Dumais, S. T. (1997). A solution to
Plato’s problem: The latent semantic analysis theory
of acquisition, induction, and representation of knowl-
edge. Psychological review, 104(2):211.
Li, J., Sun, A., Han, J., et al. (2020). A survey on deep learn-
ing for named entity recognition. IEEE Transactions
on Knowledge and Data Engineering.
Lin, B. Y., Chen, X., Chen, J., et al. (2019). Kagnet:
Knowledge-aware graph networks for commonsense
reasoning. arXiv preprint arXiv:1909.02151.
Maedche, A. and Staab, S. (2001). Ontology learning for
the semantic web. IEEE Intelligent systems, 16(2):72–
79.
Martins, P. H., Marinho, Z., and Martins, A. F. (2019). Joint
learning of named entity recognition and entity link-
ing. arXiv preprint arXiv:1907.08243.
Miller, G. A. (1998). WordNet: An electronic lexical
database. MIT press.
Moro, G., Pagliarani, A., Pasolini, R., and Sartori, C.
(2018). Cross-domain & in-domain sentiment anal-
ysis with memory-based deep neural networks. In
KDIR, pages 125–136.
O’Connor, M. J. and Das, A. K. (2009). Sqwrl: a query
language for owl. In OWLED, volume 529.
Unsupervised Descriptive Text Mining for Knowledge Graph Learning
323