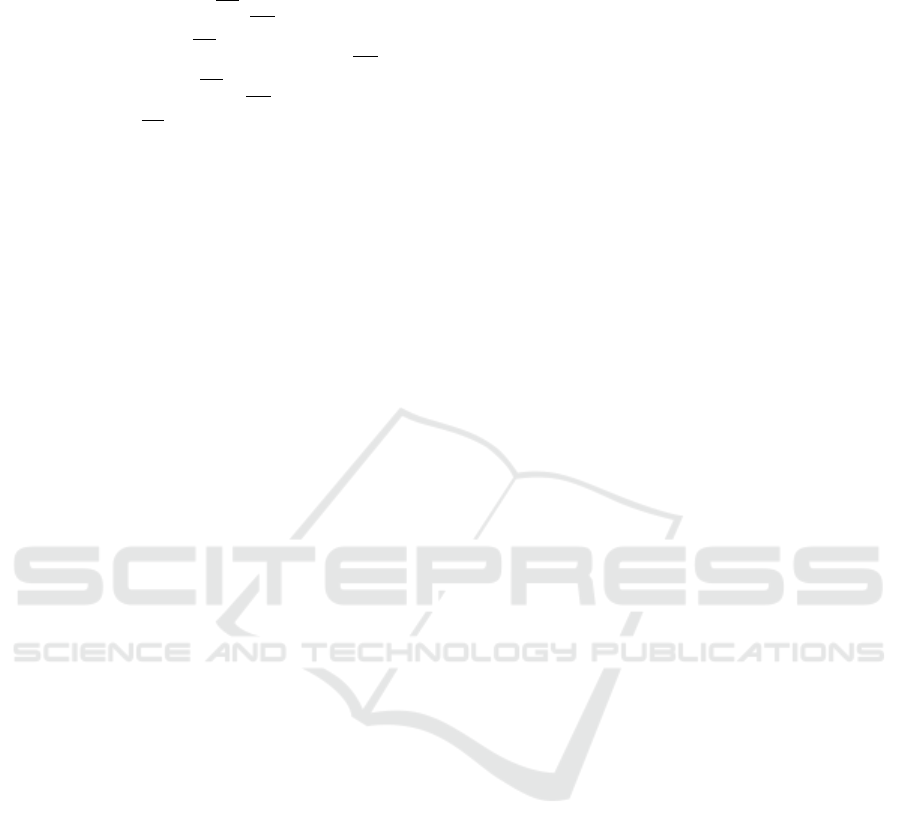
strong.
Secondly, if f (x
0
) > y
i+1
2
, let g ∈ G
←→
f
with the
constraint that g(x) = y
i+1
2
on JxK
I
S
. Such a function
g exists, because f
←→
≤ y
i+1
2
<
←→
f holds on JxK
I
S
.
Then, g
←→
= y
i+1
2
<
←→
f on JxK
I
S
, i.e., f
IFS
bw
6= g
IFS
bw
,
which contradicts the condition that f
IFS
bw
is roughly
strong.
Corollary 2. A rough real function f ∈ J
I
is (S
I
,P
J
)–
continuous on I if and only if Π
−
f
= Π
←→
f
.
Proof. f is roughly continuous
⇔ f
IFS
bw
is roughly strong by Proposition 8
⇔ f
IFS
pw
= f
IFS
bw
by Proposition 5
⇔ Π
−
f
= Π
←→
f
by Propositions 3 2.
Corollary 3. A rough real function f ∈ J
I
is (S
I
,P
J
)–
continuous on I if and only if G
−
f
= G
←→
f
.
Proof. f is roughly continuous
⇔ f
IFS
bw
is roughly strong by Proposition 8
⇔ f
IFS
pw
= f
IFS
bw
by Proposition 5
⇔ G
−
f
= G
←→
f
by Corollary 1 2.
5 CONCLUSION AND FUTURE
WORK
Rough continuity is a central notion in rough calculus.
This paper has characterized the rough continuity in
three different ways in terms of intuitionistic fuzzy
set theory.
This characterization establishes a connection be-
tween the two theories of uncertainty management,
the rough set theory and intuitionistic fuzzy set
theory. It may allow the application of the means of
intuitionistic fuzzy calculus in rough calculus.
In the future, the investigations can be continued
in several directions. This article has addressed only
one important concept of rough calculus, namely, the
rough continuity. First of all, rough continuity has
some additional features, such as rough discontinuity,
rough Darboux property or Intermediate Value
Property (IVP). The question is how they could also
be captured with the help of IFS tools. Moreover,
the relationships between additional notions of rough
calculus and IFS can also be studied.
Classical Pawlak’s rough set theory has many
different generalizations. The question is whether
they can be captured with IFS tools in one way or
another.
ACKNOWLEDGEMENTS
The author would like to thank the anonymous
referees for their useful comments and suggestions.
REFERENCES
Aquino, G., Rubio, J. D. J., Pacheco, J., Gutierrez, G. J.,
Ochoa, G., Balcazar, R., Cruz, D. R., Garcia, E.,
Novoa, J. F., and Zacarias, A. (2020). Novel nonlinear
hypothesis for the delta parallel robot modeling. IEEE
Access, 8:46324–46334.
Atanassov, K. and Gargov, G. (1989). Interval valued
intuitionistic fuzzy sets. Fuzzy Sets and Systems,
31(3):343–349.
Atanassov, K. T. (1986). Intuitionistic fuzzy sets. Fuzzy
Sets and Systems, 20(1):87–96.
Atanassov, K. T. (1999). Intuitionistic Fuzzy Sets: Theory
and Applications. Studies in Fuzziness and Soft Com-
puting. Physica-Verlag HD.
Atanassov, K. T. (2012). On Intuitionistic Fuzzy Sets The-
ory, volume 283 of Studies in Fuzziness and Soft Com-
puting. Springer Publishing Company, Incorporated.
Biswas, R. (2000). Rough sets are fuzzy sets. BUSEFAL,
(83):24–30.
Bustince, H. and Burillo, P. (1996). Vague sets are intuition-
istic fuzzy sets. Fuzzy Sets and Systems, 79(3):403–
405.
Chakraborty, M. (2011). On fuzzy sets and rough sets from
the perspective of indiscernibility. In Banerjee, M. and
Seth, A., editors, Logic and Its Applications. 4th In-
dian Conference, ICLA 2011 Delhi, India, January 5-
11, 2011, Proceedings, volume 6521 of LNAI, pages
22–37, Berlin Heidelberg. Springer-Verlag.
Chiang, H., Chen, M., and Huang, Y. (2019). Wavelet-
based EEG processing for epilepsy detection using
fuzzy entropy and associative Petri Net. IEEE Access,
7:103255–103262.
Cornelis, C., De Cock, M., and Kerre, E. (2003). Intuition-
istic fuzzy rough sets: at the crossroads of imperfect
knowledge. Expert Systems, 20(5):260–270.
Csajb
´
ok, Z. E. (2019). On the roughly continuous real func-
tions. In Mih
´
alyde
´
ak, T., Min, F., Wang, G., Baner-
jee, M., D
¨
untsch, I., Suraj, Z., and Ciucci, D., edi-
tors, Rough Sets, pages 52–65, Cham. Springer Inter-
national Publishing.
Csajb
´
ok, Z. E. (2020). On possible approaches to dif-
ferentiation of rough real functions. In Fazekas, I.,
Kov
´
asznai, G., and T
´
om
´
acs, T., editors, 11th Inter-
national Conference on Applied Informatics (ICAI),
number 2650 in CEUR Workshop Proceedings, pages
65–75, Aachen.
Rough Continuity Represented by Intuitionistic Fuzzy Sets
273