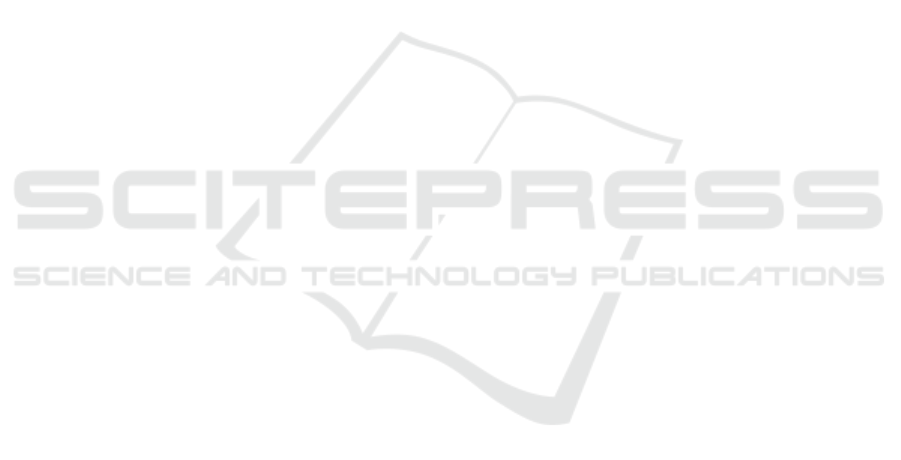
(Mokryn et al., 2019) investigated the feature of prod-
uct trendiness on purchase prediction performance.
They found a significant difference when the prod-
uct trendiness was included. In addition, works in
(Wang et al., 2015) analysed the correlation between
devices that are connected to the users when visit-
ing an e-commerce website and their purchase be-
haviours. They found that there is a positive corre-
lation in their buying behaviour and the usage of mo-
bile devices to visit e-commerce websites. However,
they did not investigate the effect of using mobile de-
vices on purchase prediction using machine learning
algorithms. In our research, we conducted an exper-
imental analysis in order to analyse the effect of op-
erating systems and platform types on the prediction
of users’ purchase intention using machine learning
algorithms. The results showed that similar to con-
sidering product trendiness as feature (Mokryn et al.,
2019); there is a positive correlation on purchase pre-
diction performance in the sessions when the machine
learning algorithms are aware of users’ operating sys-
tems and platform types. The limitation of this work
is that we compared the difference of the machine
learning algorithms’ performance on purchase predic-
tion before and after adding both features however
one of the features(os or ff) could lead better corre-
lation result.
5 CONCLUSION AND FUTURE
WORKS
In this work, we investigated the effect of the op-
erating system and platform types that users use to
browse the e-commerce platforms on their purchase
behaviour. In order to identify their impact, we
run computational experiments with and without in-
cluding operating system and platform types as fea-
tures. The results showed that the users’ purchase be-
haviours are correlated with these features.
As future research, we will integrate the purchase
prediction results with session-based recommender
systems in order to improve personalisation when
users browse the products in a session.
REFERENCES
Bader-El-Den, M., Teitei, E., and Perry, T. (2018). Biased
random forest for dealing with the class imbalance
problem. IEEE transactions on neural networks and
learning systems, 30(7):2163–2172.
Bang, Y., Han, K., Animesh, A., and Hwang, M. (2013).
From online to mobile: Linking consumers’ online
purchase behaviors with mobile commerce adoption.
In PACIS, page 128.
Brzezinski, D., Stefanowski, J., Susmaga, R., and Szczech,
I. (2018). Visual-based analysis of classification mea-
sures and their properties for class imbalanced prob-
lems. Information Sciences, 462:242–261.
Carre
´
on, E. C. A., Nonaka, H., Hentona, A., and Yamashiro,
H. (2019). Measuring the influence of mere exposure
effect of tv commercial adverts on purchase behavior
based on machine learning prediction models. Infor-
mation Processing & Management, 56(4):1339–1355.
Choudhury, A. M. and Nur, K. (2019). A machine learn-
ing approach to identify potential customer based on
purchase behavior. In 2019 International Conference
on Robotics, Electrical and Signal Processing Tech-
niques (ICREST), pages 242–247. IEEE.
Christy, A. J., Umamakeswari, A., Priyatharsini, L., and
Neyaa, A. (2018). Rfm ranking–an effective approach
to customer segmentation. Journal of King Saud
University-Computer and Information Sciences.
Esmeli, R., Bader-El-Den, M., and Abdullahi, H. (2020).
Using word2vec recommendation for improved pur-
chase prediction. In IEEE World Congress on Compu-
tational Intelligence (WCCI) 2020. Institute of Elec-
trical and Electronics Engineers.
Esmeli, R., Bader-El-Den, M., and Mohasseb, A. (2019).
Context and short term user intention aware hybrid
session based recommendation system. In 2019 IEEE
International Symposium on INnovations in Intelli-
gent SysTems and Applications (INISTA), pages 1–6.
IEEE.
Gupta, R. and Pathak, C. (2014). A machine learning frame-
work for predicting purchase by online customers
based on dynamic pricing. Procedia Computer Sci-
ence, 36:599–605.
Kaatz, C., Brock, C., and Figura, L. (2019). Are you
still online or are you already mobile?–predicting the
path to successful conversions across different de-
vices. Journal of Retailing and Consumer Services,
50:10–21.
Kim, E., Kim, W., and Lee, Y. (2003). Combination of mul-
tiple classifiers for the customer’s purchase behavior
prediction. Decision Support Systems, 34(2):167–175.
Korpusik, M., Sakaki, S., Chen, F., and Chen, Y.-Y. (2016).
Recurrent neural networks for customer purchase pre-
diction on twitter. In CBRecSys@ RecSys, pages 47–
50.
Liu, H., Lobschat, L., Verhoef, P. C., and Zhao, H. (2019).
App adoption: The effect on purchasing of customers
who have used a mobile website previously. Journal
of Interactive Marketing, 47:16–34.
Mart
´
ınez, A., Schmuck, C., Pereverzyev Jr, S., Pirker, C.,
and Haltmeier, M. (2020). A machine learning frame-
work for customer purchase prediction in the non-
contractual setting. European Journal of Operational
Research, 281(3):588–596.
Mohasseb, A., Bader-El-Den, M., and Cocea, M. (2019). A
customised grammar framework for query classifica-
tion. Expert Systems with Applications, 135:164–180.
Analysing the Effect of Platform and Operating System Features on Predicting Consumers’ Purchase Intent using Machine Learning
Algorithms
339