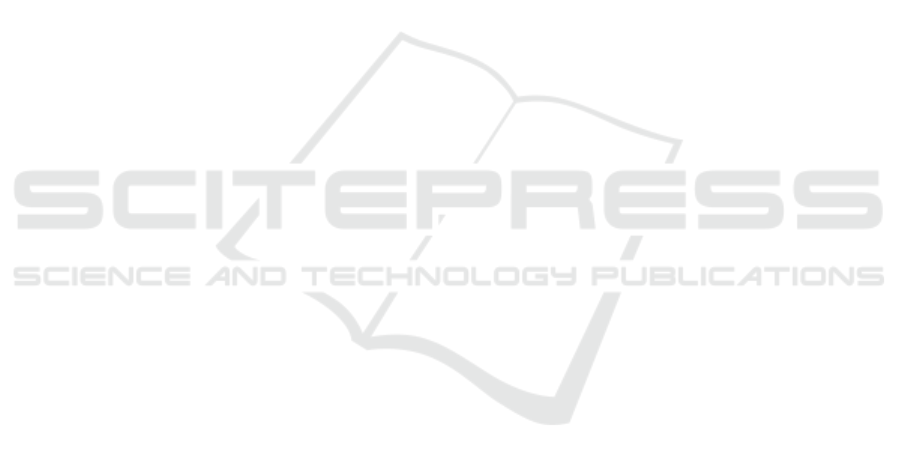
tual Information Based Feature Selection with
Generalized Minimum-Redundancy and Maximum-
Relevance Criteria. Entropy, 18(9):330. ZSCC:
0000009.
Huang, N., Lu, G., and Xu, D. (2016b). A Permuta-
tion Importance-Based Feature Selection Method for
Short-Term Electricity Load Forecasting Using Ran-
dom Forest. Energies, 9(10):767. ZSCC: 0000027.
Janicki, M. (2017). Methods of weather variables introduc-
tion into short-term electric load forecasting models
- a review. Przeglad Elektrotechniczny, 1(4):72–75.
ZSCC: 0000001.
Jiang, P., Liu, F., and Song, Y. (2017). A hybrid fore-
casting model based on date-framework strategy and
improved feature selection technology for short-term
load forecasting. Energy, 119:694–709. ZSCC:
0000065.
Kandil, N., Wamkeue, R., Saad, M., and Georges, S. (2006).
An Efficient Approach for Shorterm Load Forecasting
using Artificial Neural Networks. In 2006 IEEE In-
ternational Symposium on Industrial Electronics, vol-
ume 3, pages 1928–1932. ZSCC: 0000203 ISSN:
2163-5145.
Khatoon, S., Ibraheem, Singh, A. K., and Priti (2014). Ef-
fects of various factors on electric load forecasting:
An overview. In 2014 6th IEEE Power India Interna-
tional Conference (PIICON), pages 1–5. ZSCC: NoC-
itationData[s0] ISSN: null.
L
´
opez, M., Valero, S., Senabre, C., and Gabald
´
on, A.
(2017). Analysis of the influence of meteorological
variables on real-time Short-Term Load Forecasting
in Balearic Islands. In 2017 11th IEEE International
Conference on Compatibility, Power Electronics and
Power Engineering (CPE-POWERENG), pages 10–
15. ZSCC: 0000004 ISSN: 2166-9546.
L
´
opez Garc
´
ıa, M., Valero, S., Senabre, C., and Ga-
bald
´
on Mar
´
ın, A. (2013). Short-Term Predictability
of Load Series: Characterization of Load Data Bases.
IEEE Transactions on Power Systems, 28(3):2466–
2474. ZSCC: 0000011.
Ma, J. and Ma, X. (2017). State-of-the-art forecasting al-
gorithms for microgrids. In 2017 23rd International
Conference on Automation and Computing (ICAC),
pages 1–6. ZSCC: 0000006 ISSN: null.
Marinescu, A., Harris, C., Dusparic, I., Clarke, S., and
Cahill, V. (2013). Residential electrical demand fore-
casting in very small scale: An evaluation of forecast-
ing methods. In 2013 2nd International Workshop on
Software Engineering Challenges for the Smart Grid
(SE4SG), pages 25–32. ZSCC: 0000053 ISSN: null.
Matija
ˇ
s, M., Cerjan, M., and Krajcar, S. (2011). Features
affecting the load forecasting error on country level.
In Proceedings of the 2011 3rd International Youth
Conference on Energetics (IYCE), pages 1–7. ZSCC:
0000008 ISSN: null.
Mazzola, L., Denzler, A., and Christen, R. (2020). To-
wards a Peer-to-Peer Energy Market: an Overview.
arXiv:2003.07940 [physics]. ZSCC: NoCitation-
Data[s0] arXiv: 2003.07940.
Mirasgedis, S., Sarafidis, Y., Georgopoulou, E., Lalas, D. P.,
Moschovits, M., Karagiannis, F., and Papakonstanti-
nou, D. (2006). Models for mid-term electricity de-
mand forecasting incorporating weather influences.
Energy, 31(2):208–227. ZSCC: 0000258.
Mirowski, P., Chen, S., Ho, T. K., and Yu, C.-N. (2014). De-
mand forecasting in smart grids. Bell Labs Technical
Journal, 18(4):135–158. ZSCC: 0000085.
Mustapha, M., Mustafa, M. W., Khalid, S. N., Abubakar,
I., and Abdilahi, A. M. (2016). Correlation and
Wavelet-based Short-Term Load Forecasting using
Anfis. Indian Journal of Science and Technology,
9(46). ZSCC: 0000006.
Niu, D., Wang, Y., and Wu, D. D. (2010). Power load
forecasting using support vector machine and ant
colony optimization. Expert Systems with Applica-
tions, 37(3):2531–2539. ZSCC: 0000305.
Rahman, S. and Hazim, O. (1993). A generalized
knowledge-based short-term load-forecasting tech-
nique. IEEE Transactions on Power Systems,
8(2):508–514. ZSCC: 0000325.
Rana, M. and Koprinska, I. (2012a). Electricity load fore-
casting using non-decimated wavelet prediction meth-
ods with two-stage feature selection. In The 2012
International Joint Conference on Neural Networks
(IJCNN), pages 1–8. ZSCC: 0000005 ISSN: 2161-
4407.
Rana, M. and Koprinska, I. (2012b). Shift Invariance and
Border Distortion in Wavelet-Based Electricity Load
Forecasting. In 21st International Conference on
Pattern Recognition (ICPR 2012), page 4. ZSCC:
0000000.
Rana, M. and Koprinska, I. (2013). Wavelet Neural Net-
works for Electricity Load Forecasting – Dealing with
Border Distortion and Shift Invariance. In Mlade-
nov, V., Koprinkova-Hristova, P., Palm, G., Villa, A.
E. P., Appollini, B., and Kasabov, N., editors, Artifi-
cial Neural Networks and Machine Learning – ICANN
2013, Lecture Notes in Computer Science, pages 571–
578, Berlin, Heidelberg. Springer. ZSCC: NoCitation-
Data[s0].
Raza, M. Q. and Khosravi, A. (2015). A review on artifi-
cial intelligence based load demand forecasting tech-
niques for smart grid and buildings. Renewable and
Sustainable Energy Reviews, 50:1352–1372. ZSCC:
0000334.
Sahay, K. B. and Tripathi, M. (2014). Day ahead hourly
load forecast of PJM electricity market and iso new
england market by using artificial neural network. In
ISGT 2014, pages 1–5. ZSCC: 0000029 ISSN: null.
Saleh, A. I., Rabie, A. H., and Abo-Al-Ez, K. M. (2016). A
data mining based load forecasting strategy for smart
electrical grids. Advanced Engineering Informatics,
30(3):422–448. ZSCC: 0000034.
Silva, L. N., Abaide, A. R., Figueir
´
o, I. C., Martinuzzi,
D., and Rigodanzo, J. (2017). Development of an
ANN model to multi-region short-term load forecast-
ing based on power demand patterns recognition. In
2017 IEEE PES Innovative Smart Grid Technologies
Conference - Latin America (ISGT Latin America),
pages 1–6. ZSCC: 0000001.
Exogenous Data for Load Forecasting: A Review
499