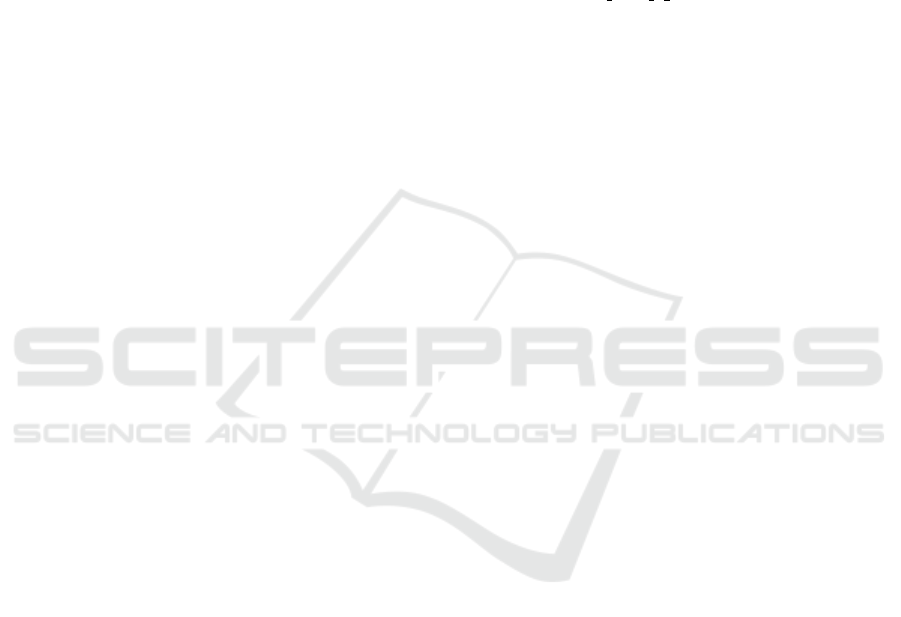
target audience; ability to work in a team.
• Partial search. The study material is presented
by the teacher in part (a certain part of the topic),
and the rest of the students work independently.
However, the teacher directs the work of appli-
cants with questions or pre-selected tasks to pre-
vent errors in their activities or found the wrong
solution.
• Research. The method is quite difficult to use
because it requires additional training from the
teacher and is quite time-consuming. Provides in-
dependence of students in the study of a particular
topic or theoretical aspect, its practical implemen-
tation in cloud-based learning technologies Co-
Calc, Wolfram Alpha, or the study of additional
topics related to the topic of the course, but not
considered due to time constraints on learning dis-
cipline. Researching the problem develops the
ability to conduct research, the ability to use hard-
ware and specialized cloud services, obtain addi-
tional data and interpret them, the ability to work
independently, all together are components of pro-
fessional competencies formed at the appropriate
level of a successful future statistician.
The means of forming the professional competen-
cies of future bachelors of statistics, which are speci-
fied in the presented methodology using cloud-based
learning technologies, include CoCalc and Wolfram
Alpha, textbooks or teaching materials, as well as
computers (laptops, tablets, smartphones) with an ac-
tive connection to the Internet.
The result of the proposed methodology is the
formed professional competencies of future bachelors
of statistics at a high level, as well as the success-
ful application of skills to use CoCalc and Wolfram
Alpha to perform practical work in the professional
field.
4 CONCLUSIONS
Therefore, according to the research, the most appro-
priate, convenient, and effective cloud-oriented learn-
ing technologies for the formation of professional
competencies of future bachelors of statistics by the
manifestation of all criteria are cloud-oriented learn-
ing technologies CoCalc and Wolfram Alpha. The
general structure of the methodology of using cloud
learning technologies for the formation of profes-
sional competencies of future bachelors of statistics
is described. In the future, it is planned to describe in
more detail the individual components of the method-
ology of using cloud learning technologies for the for-
mation of professional competencies of future bache-
lors of statistics, in particular the forms of use and
forms of organization of the educational process.
REFERENCES
Bagrii, V. N. (2012). Criteria and levels of future social
educators’ professional skills. Collection of scientific
works of Khmelnytskyi Institute of Social Technolo-
gies, University of Ukraine, (6). http://nbuv.gov.ua/j-
pdf/Znpkhist
2012 6 4.pdf.
Busel, V. T., editor (2005). The Great Interpretative Dictio-
nary of Modern Ukrainian Language. Perun, Kyiv.
Bykov, V. Y., Spirin, O. M., and Luparenko, L. A. (2014).
Open web-oriented systems for monitoring the imple-
mentation of scientific and pedagogical research re-
sults. The theory and practice of social systems man-
agement, (1):3–25.
Demyanenko, V. M., Lavrentieva, G. P., and Shyshkina,
M. P. (2013). Guidelines for the selection and use
of electronic tools and resources for educational pur-
poses. The computer at school and family, (1):44–48.
https://lib.iitta.gov.ua/748/.
Dychkivska, I. M. (2004). Innovative pedagogical tech-
nologies. Akademvydav, Kyiv.
Gavryliuk, O., Vakaliuk, T., and Kontsedailo, V. (2020). Se-
lection criteria for cloud-oriented learning technolo-
gies for the formation of professional competencies of
bachelors majoring in statistics. SHS Web of Confer-
ences, 75:04012.
Glazunova, O. G., Kuzminska, O. G., Voloshyna, T. V.,
Sayapina, T. P., and Korolchuk, V. I. (2017). Mi-
crosoft and Google cloud services: organizing group
project work for university students. Open educa-
tional e-environment of Modern University, (3):199–
211. http://openedu.kubg.edu.ua/journal/index.php/
openedu/article/view/84/135.
Golovnia, O. S. (2015). Criteria for selecting virtualization
software to train UNIX-like operating systems. Infor-
mation technology in education, (24):119–133.
Honcharenko, S. U., editor (2000). Vocational education:
vocabulary. Vyshcha shkola, Kyiv.
Kolos, K. R. (2013). Process model and component selec-
tion criteria for a computer-based educational environ-
ment for a postgraduate teacher education institution.
Information technology in education, (17):109–117.
Korotun, O. V. (2018). The use of the cloud-oriented en-
vironment in training databases of future computer
science teachers. PhD thesis, Institute of Informa-
tion Technologies and Learning Tools of the NAES
of Ukraine, Kyiv. https://lib.iitta.gov.ua/711959/.
Kremen, V., editor (2016). National report on
the state and prospects of education develop-
ment in Ukraine. Pedahohichna dumka, Kyiv.
https://mon.gov.ua/storage/app/media/nrk/Analitychni-
materialy/7-natsionalna-dopovid-pro-stan-i-rozvitok-
osviti-v-ukraini.pdf.
AET 2020 - Symposium on Advances in Educational Technology
140