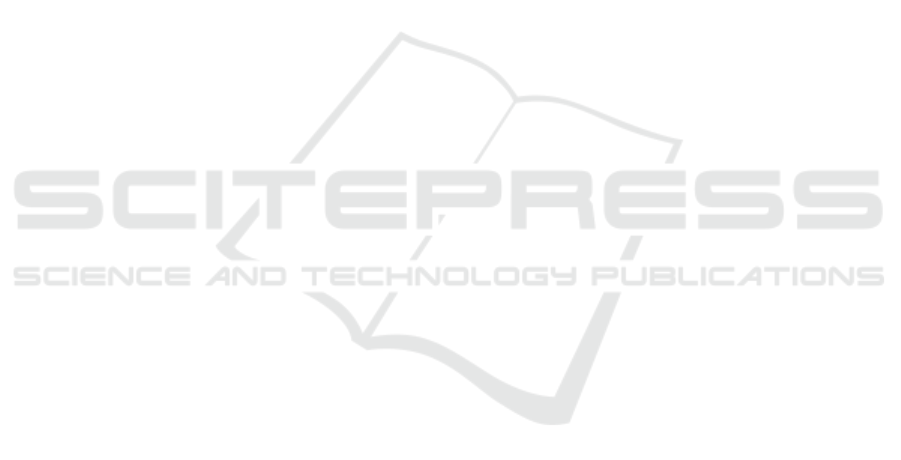
Freund, Y. and Schapire, R. E. (1996). Experiments with
a new boosting algorithm. In Proceedings of the
Thirteenth International Conference on International
Conference on Machine Learning, ICML’96, page
148–156, San Francisco, CA, USA. Morgan Kauf-
mann Publishers Inc.
Hsieh, W.-H., Shih, D.-H., Shih, P.-Y., and Lin, S.-B.
(2019). An ensemble classifier with case-based rea-
soning system for identifying internet addiction. Inter-
national journal of environmental research and public
health, 16(7):1233.
Huizinga, J. (2016). Homo Ludens: A Study of the Play-
Element in Culture. Angelico Press.
Hussain, R. G., Ghazanfar, M. A., Azam, M. A., Naeem, U.,
and Ur Rehman, S. (2019). A performance compari-
son of machine learning classification approaches for
robust activity of daily living recognition. Artificial
Intelligence Review, 52(1):357–379.
ICD-11 for Mortality and Morbidity Statistics (Ver-
sion: 09/2020) (2020). 6C51 Gaming disor-
der. https://icd.who.int/browse11/l-m/en#/http://id.
who.int/icd/entity/1448597234.
Ji, H.-M., Chen, L.-Y., and Hsiao, T.-C. (2019). Real-
time detection of internet addiction using reinforce-
ment learning system. In Proceedings of the Genetic
and Evolutionary Computation Conference Compan-
ion, pages 1280–1288.
Keng, B. (2016). The expectation-maximization algo-
rithm. http://bjlkeng.github.io/posts/the-expectation-
maximization-algorithm.
Kittler, J., Hatef, M., Duin, R. P., and Matas, J. (1998). On
combining classifiers. IEEE transactions on pattern
analysis and machine intelligence, 20(3):226–239.
Klochko, O. and Fedorets, V. (2019). An empirical com-
parison of machine learning clustering methods in the
study of Internet addiction among students majoring
in Computer Sciences. CEUR Workshop Proceedings,
2546:58–75.
Klochko, O., Fedorets, V., Tkachenko, S., and Maliar, O.
(2020). The use of digital technologies for flipped
learning implementation. CEUR Workshop Proceed-
ings, 2732:1233–1248.
Klochko, O. V. (2019). Matematychne modeliuvannia
system i protsesiv v osviti/pedahohitsi (Mathemat-
ical modeling of systems and processes in educa-
tion/pedagogy). Druk.
Kr
¨
amer, J., Schrey
¨
ogg, J., and Busse, R. (2019). Classifica-
tion of hospital admissions into emergency and elec-
tive care: a machine learning approach. Health Care
Management Science, 22(1):85–105.
Kuncheva, L. I. (2014). Combining pattern classifiers:
methods and algorithms. John Wiley & Sons.
Lee, T.-H., Ullah, A., and Wang, R. (2020). Bootstrap ag-
gregating and random forest. In Fuleky, P., editor,
Macroeconomic Forecasting in the Era of Big Data:
Theory and Practice, pages 389–429. Springer Inter-
national Publishing, Cham.
Leontyev, D. A. (2017). Psikhologiya smysla: priroda.
stroyeniye i dinamika smyslovoy realnosti (The psy-
chology of meaning: nature, structure and dynamics
of meaningful reality). Litres.
Linoff, G. S. and Berry, M. J. A. (2011). Data mining tech-
niques: for marketing, sales, and customer relation-
ship management. John Wiley & Sons.
Moshtaghi, M., Bezdek, J. C., Erfani, S. M., Leckie, C.,
and Bailey, J. (2019). Online cluster validity indices
for performance monitoring of streaming data clus-
tering. International Journal of Intelligent Systems,
34(4):541–563.
Pacol, C. A. and Palaoag, T. D. (2020). En-
hancing sentiment analysis of textual feedback in
the student-faculty evaluation using machine learn-
ing techniques. In 3rd International Confer-
ence On Academic Research in Science, Technology
and Engineering. https://www.dpublication.com/wp-
content/uploads/2020/11/3-3003.pdf.
Santos, S. G. T. d. C. and de Barros, R. S. M. (2020).
Online AdaBoost-based methods for multiclass prob-
lems. Artificial Intelligence Review, 53(2):1293–
1322.
Souri, A., Ghafour, M. Y., Ahmed, A. M., Safara, F.,
Yamini, A., and Hoseyninezhad, M. (2020). A new
machine learning-based healthcare monitoring model
for student’s condition diagnosis in Internet of Things
environment. Soft Computing, 24(22):17111–17121.
Subasi, A., Kevric, J., and Abdullah Canbaz, M. (2019).
Epileptic seizure detection using hybrid machine
learning methods. Neural Computing and Applica-
tions, 31(1):317–325.
Sudakov, K. V. (2011). Funktsionalnyye sistemy (Func-
tional systems). Izdatelstvo RAMN.
Suma, S. N., Nataraja, P., and Sharma, M. K. (2021). Inter-
net addiction predictor: Applying machine learning in
psychology. In Advances in Artificial Intelligence and
Data Engineering, pages 471–481. Springer.
Tarasenko, A., Yakimov, Y., and Soloviev, V. (2019). Con-
volutional neural networks for image classification.
CEUR Workshop Proceedings, 2546:101–114.
Tuysuzoglu, G. and Birant, D. (2020). Enhanced bagging
(ebagging): A novel approach for ensemble learning.
The International Arab Journal of Information Tech-
nology, 17(4):515–528.
Wallis, D. (1997). Just click no: Talk story about Dr. Ivan K.
Goldberg and the internet addiction disorder. The New
Yorker. http://www.newyorker.com/magazine/1997/
01/13/just-click-no.
Weka (2021). Weka 3: Machine Learning Software in Java.
http://old-www.cms.waikato.ac.nz/ ml/weka/.
Young, K. S. (1998a). Caught in the net: How to recognize
the signs of internet addiction–and a winning strategy
for recovery. John Wiley & Sons.
Young, K. S. (1998b). Internet addiction: The emergence of
a new clinical disorder. CyberPsychology & Behav-
ior, 1(3):237–244. https://www.liebertpub.com/doi/
pdf/10.1089/cpb.1998.1.237.
Yuryeva, L. N. and Bolbot, T. Y. (2006). Kompyuter-
naya zavisimost: formirovaniye, diagnos-
tika, korrektsiya i profilaktika (Computer ad-
diction: formation, diagnostics, correction
The Use of Ensemble Classification and Clustering Methods of Machine Learning in the Study of Internet Addiction of Students
259