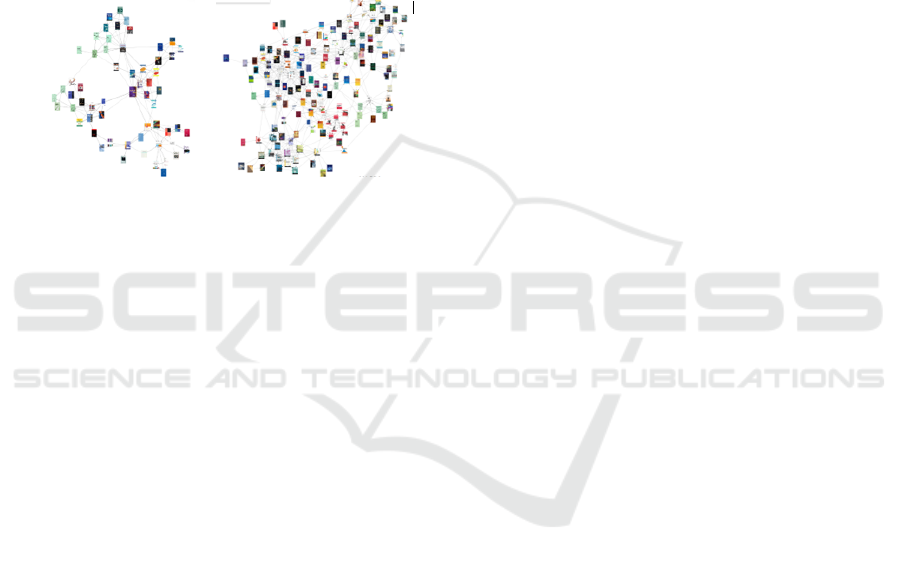
the left), where 5 subgraphs can be observed, there is
the third edition of the bestseller, Principles and Prac-
tice of Modeling by Structural Equations by Kline
(Kline, 2015). The companion site of this publication
provides methodological support and offers download
syntax, data and source files for all sample books for
execution in three environments EQS, LISREL and
Mplus, and a comparison of simulation results. A
similar experiment, conducted on March 28, 2019
(figure 1, on the right), shows interest growth in struc-
tural modeling; we have 157 items. Interestingly, the
fourth edition of the same bestseller has the biggest
rating there.
Figure 1: Books on “ structural equation modeling”, Ama-
zon.com, year 2013 versus 2019.
While solving the scientific problem of training
PhDs to use the SEM methodology, the following
main results were obtained in past author works: the
content of the simulation training by the structural
equations of specialists in the field of education is re-
vealed; the dynamics of software simulation by struc-
tural equations is analyzed; the necessity of including
these means in the courses for students and graduates
of higher educational institutions of Ukraine that spe-
cialize in the field of education and social sciences is
substantiated (Panchenko, 2013).
The syllabuses of PhD SEM courses of lead-
ing universities are analyzed (Higher School of Eco-
nomics, St. Petersburg; University of Amster-
dam; University of Vaasa, Finland; University of
Mannheim; Iowa State University; Brown University;
University of Leuven; School of Education University
of Pittsburgh; Oslo University etc).
An analysis of the best PhD programs in sociol-
ogy according to the Princeton Review’s “Gourman
Report of Graduate Programs” (Plous, 2021) showed
that they must have courses on structural equation
modelling. In table 1, we summarize the use of var-
ious SEM software in the training of doctors of phi-
losophy of the world’s leading universities.
Analysis of syllabusis (table 1) showed that in
general the courses are organized in the format of
seminars. The main activity is created at the intersec-
tion of lectures, group discussions, software applica-
tion and interpretation of results. Teaching materials
are available through the Blackboard or Moodle learn-
ing management system. The number of course cred-
its ranges from 1 to 6 with 1–3 credits for introductory
courses, and 4–6 credits for advanced. Courses are of-
fered as part of the training of doctors of philosophy
in the field of pedagogy, psychology, sociology, statis-
tics, information systems and business, public health,
sports, etc. Both proprietary and free software is used.
In most courses, one software tool is acting as the
main tool, and another one as an additional. But there
are courses in which several software tools are widely
used.
Here is an example of evaluating the work of
students in the course (www.soc.iastate.edu, 2021).
Homework with the use statistical software: 8%; crit-
ical review of articles on the topic (4 reviews of 4–5
pages): 30%; statistical analysis (4 reports of 12–15
pages): 62%. For a critical review, the parameters
of its evaluation are given: for example, to explain
the basic model that the author evaluates (20% of the
mark); discussion of critical mistakes made by the au-
thor (40%); an explanation of how the casual effect
(40%) can be correctly estimated. Some programs of-
fer an exam and a final project during the course as
their own mini-study on the use of SEM.
The objectives of the SEM courses are defined as
follows: using structural equation modeling method-
ology to study the problems of social and behavioral
science, understanding the strengths and flaws of the
method and its limitations, teaching methods of as-
sessment, identification models, testing their validity,
interpretation, critical evaluation of scientific publi-
cations on this subject, using statistical software to
perform structural equation modeling analysis, prepa-
ration of research reports in accordance with the stan-
dards of research (Panchenko and Razoronova, 2016).
In preparing specialists for using SEM an im-
portant point is to select or obtain the necessary
data sets that are representative and valid. We offer
our students the survey data from Ukrainian teachers
(Shchudlo et al., 2018; TALIS, 2017; Questionnaires,
2017).
On August 31, 2017, the Ukrainian Associa-
tion of Educational Researchers completed the All-
Ukrainian monitoring “Teaching and Learning Sur-
vey on Principals and Teachers of Secondary Edu-
cation Institutions” (based on the TALIS methodol-
ogy (OECD, 2018). The study was conducted within
the framework of the project “Teacher” and “Edu-
cation Reform: Quality Assessment in an Interna-
tional Context”, which is implemented by the All-
Ukrainian Foundation “Step by Step” with the support
of the Ministry of Education and Science of Ukraine
(Shchudlo et al., 2018). The study was attended by
Structural Equation Modeling in Educational Research: A Case-study for PhD Training
301