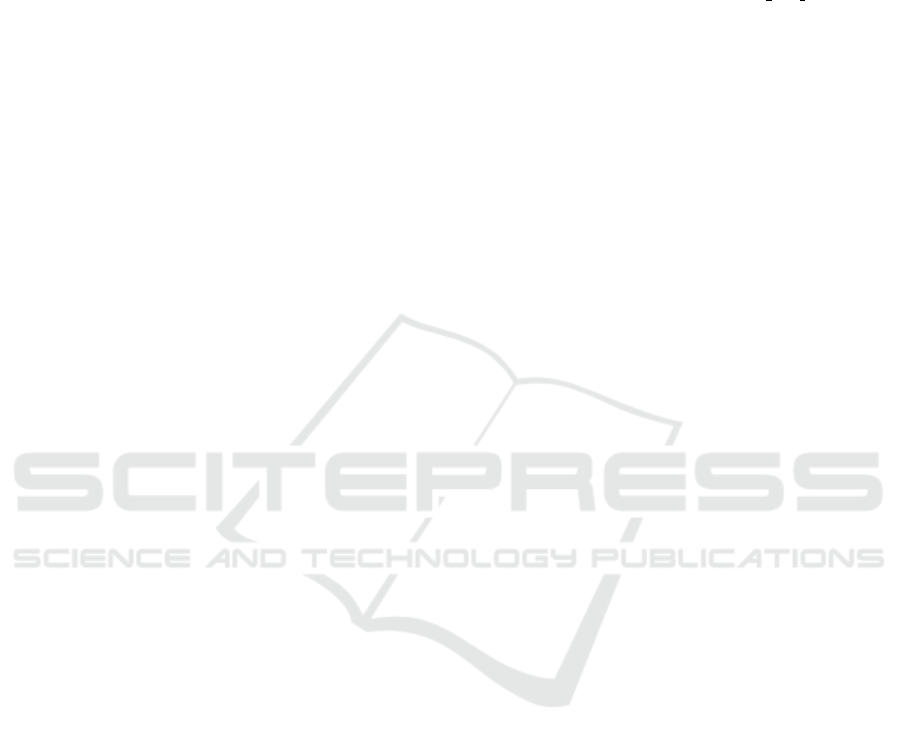
George, G., Osinga, E. C., Lavie, D., and Scott, B. A.
(2016). Big data and data science methods for man-
agement research. The Academy of Management Jour-
nal, 59(5):1493–1507.
Griffiths, T. L. and Steyvers, M. (2004). Finding scientific
topics. Proceedings of the National Academy of Sci-
ences, 101(suppl 1):5228–5235.
Guryanova, L., Bogachkova, L., Zyma, O., Novosel, M.,
Poluektova, N., and Gvozdytskyi, V. (2020a). Mod-
els of estimation and analysis of a systemic risk in
the banking sector. In 2020 IEEE 2nd International
Conference on System Analysis Intelligent Computing
(SAIC), pages 1–6.
Guryanova, L., Yatsenko, R., Dubrovina, N., and Babenko,
V. (2020b). Machine learning methods and models,
predictive analytics and applications. CEUR Work-
shop Proceedings, 2649:1–5.
Hrabovskyi, Y., Babenko, V., Al’Boschiy, O., and Gerasi-
menko, V. (2020). Development of a Technology for
Automation of Work with Sources of Information on
the Internet. WSEAS Transactions on Business and
Economics, 17(25):231–240.
Hu, N., Zhang, T., Gao, B., and Bose, I. (2019). What
do hotel customers complain about? text analysis
using structural topic model. Tourism Management,
72:417–426.
Ignatyuk, A., Liubkina, O., Murovana, T., and Magome-
dova, A. (2020). FinTech as an innovation challenge:
From big data to sustainable development. E3S Web
of Conferences, 166:13027.
Ilchuk, M., Davydenko, N., and Nehoda, Y. (2019). Sce-
nario modeling of financial resources at the enterprise.
Intellectual Economics, 13(2).
James, G., Witten, D., Hastie, T., and Tibshirani, R. (2013).
An introduction to statistical learning: with Applica-
tions in R, volume 112 of Springer Texts in Statistics.
Springer, New York.
James, T. L., Calderon, E. D. V., and Cook, D. F. (2017).
Exploring patient perceptions of healthcare service
quality through analysis of unstructured feedback. Ex-
pert Systems with Applications, 71:479–492.
Kaminskyi, A., Nehrey, M., and Rizun, N. (2020). The im-
pact of COVID-induced shock on the risk-return cor-
respondence of agricultural ETFs. CEUR Workshop
Proceedings, 2713:204–218.
Klymenko, N., Nosovets, O., Sokolenko, L., Hryshchenko,
O., and Pisochenko, T. (2019). Off-balance
accounting in the modern information sys-
tem of an enterprise. Academy of Account-
ing and Financial Studies Journal, 23(2).
https://www.abacademies.org/articles/offbalance-
accounting-in-the-modern-information-system-of-an-
enterprise-8403.html.
Kobayashi, V. B., Mol, S. T., Berkers, H. A., Kismihok, G.,
and Den Hartog, D. N. (2018a). Text classification for
organizational researchers: A tutorial. Organizational
research methods, 21(3):766–799.
Kobayashi, V. B., Mol, S. T., Berkers, H. A., Kismih
´
ok, G.,
and Den Hartog, D. N. (2018b). Text mining in orga-
nizational research. Organizational research methods,
21(3):733–765.
Kuzmenko, O. V., Lieonov, S. V., and Boiko, A. O.
(2020). Data mining and bifurcation analysis of the
risk of money laundering with the involvement of fi-
nancial institutions. Journal of International Studies,
13(3). https://www.jois.eu/files/22 871 Kuzmenko%
20et%20al.pdf.
L
´
opez, A., Detz, A., Ratanawongsa, N., and Sarkar, U.
(2012). What patients say about their doctors online:
a qualitative content analysis. Journal of general in-
ternal medicine, 27(6):685–692.
Matviychuk, A., Strelchenko, I., Vashchaiev, S., and Ve-
lykoivanenko, H. (2019). Simulation of the crisis con-
tagion process between countries with different lev-
els of socio-economic development. CEUR Workshop
Proceedings, 2393:485–496.
National Academies of Sciences, Engineering, and
Medicine, Division on Engineering and Physical Sci-
ences, Division of Behavioral and Social Sciences and
Education, Computer Science and Telecommunica-
tions Board, Board on Mathematical Sciences and An-
alytics, Committee on Applied and Theoretical Statis-
tics, Board on Science Education, and Committee on
Envisioning the Data Science Discipline: The Under-
graduate Perspective (2018). Data science for under-
graduates: Opportunities and options. The National
Academies Press, Washington, DC.
Nehrey, M. and Hnot, T. (2017). Using recommendation
approaches for ratings matrixes in online marketing.
Studia Ekonomiczne, (342):115–130.
Nehrey, M., Kaminskyi, A., and Komar, M. (2019). Agro-
economic models: a review and directions for re-
search. Periodicals of Engineering and Natural Sci-
ences, 7(2):702–711. http://pen.ius.edu.ba/index.php/
pen/article/view/579.
Nosratabadi, S., Mosavi, A., Duan, P., Ghamisi, P., Filip,
F., Band, S. S., Reuter, U., Gama, J., and Gandomi,
A. H. (2020). Data science in economics: comprehen-
sive review of advanced machine learning and deep
learning methods. Mathematics, 8(10):1799.
Ojo, A. and Rizun, N. (2020). Structural and temporal topic
models of feedbacks on service quality–a path to the-
ory development? In Americas Conference on In-
formation Systems (AMCIS 2020). Healthcare Infor-
matics & Health Information Tech (SIGHealth), vol-
ume 15.
Oliskevych, M., Beregova, G., and Tokarchuk, V.
(2018). Fuel consumption in Ukraine: Evi-
dence from vector error correction model. Inter-
national Journal of Energy Economics and Policy,
8(5). https://www.econjournals.com/index.php/ijeep/
article/view/6825/3925.
Oliskevych, M. and Lukianenko, I. (2019). Labor force
participation in Eastern European countries: nonlinear
modeling. Journal of Economic Studies, 46(6):1258–
1279.
Parish, E. J. and Duraisamy, K. (2016). A paradigm for
data-driven predictive modeling using field inversion
and machine learning. Journal of Computational
Physics, 305:758–774.
Data Science in Economics Education: Examples and Opportunities
563