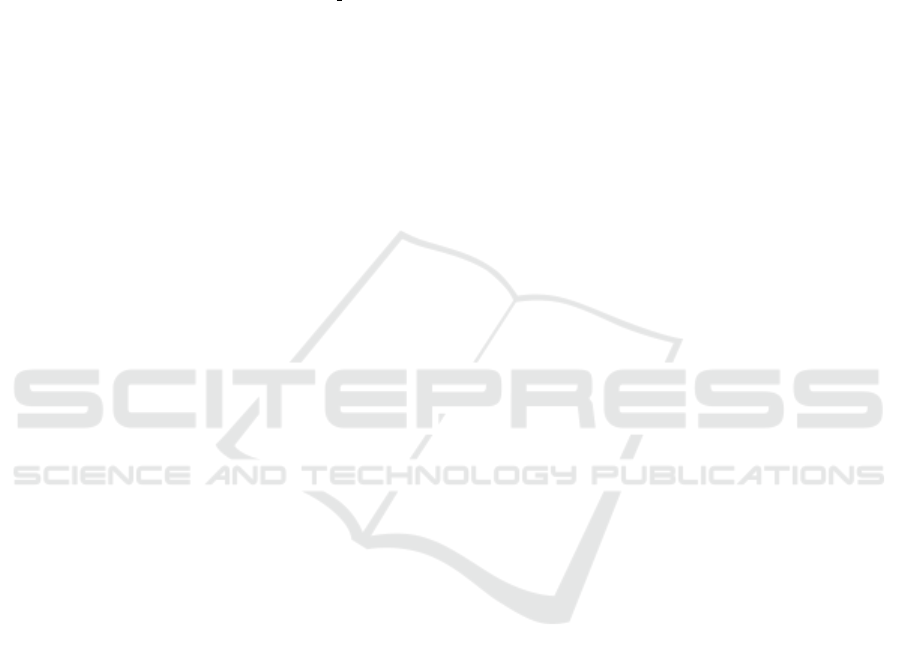
mation system. Upravlinnia rozvytkom skladnykh sys-
tem, 32:86–90.
Gorohovskiy, A. and Troyanovskaya, T. (2015). Informa-
tion technology for building the adaptive system of
distance learning. Scientific Works of Vinnytsia Na-
tional Technical University, (2). https://works.vntu.
edu.ua/index.php/works/article/view/140.
Huang, S.-L. and Shiu, J.-H. (2012). A User-Centric Adap-
tive Learning System for E-Learning 2.0. Journal
of Educational Technology & Society, 15(3):214–225.
https://www.ds.unipi.gr/et&s/journals/15 3/16.pdf.
Kristensen, T., Lamo, Y., Mughal, K., Tekle, K. M., and
Bottu, A. K. (2007). Towards a dynamic, content
based e-learning platform. In Proceedings of the 10th
IASTED International Conference on Computers and
Advanced Technology in Education, CATE ’07, page
107–114, USA. ACTA Press.
Kruglik, V. S. and Chorna, A. V. (2020). Using the Moodle
platform to individualize the training of future profes-
sionals in a blended learning environment. In Informa-
tion technologies in education, science and technol-
ogy: theses add. V International Scientific-practical
Conf. (Cherkasy, May 21-23, 2020), pages 159–160.
Cherkasy State Technological University, Cherkasy.
Kurgan, G. S. (2013). Cloud technologies of inter-
net education based on the knowledge repre-
sentation model. Bulletin of the Buryat State
University. Mathematics, computer science,
(9). https://cyberleninka.ru/article/n/oblachnye-
tehnologii-internet-obrazovaniya-na-osnove-kfs-
modeli-predstavleniya-znaniy/viewer.
Osadcha, K., Osadchyi, V., Semerikov, S., Chemerys, H.,
and Chorna, A. (2020). The review of the adaptive
learning systems for the formation of individual ed-
ucational trajectory. CEUR Workshop Proceedings,
2732:547–558.
Osadchyi, V. V. and Krasheninnik, I. V. (2020). LMS Moo-
dle as a tool for personalizing the training of future
professionals. In Information technologies in educa-
tion, science and technology: theses add. V Interna-
tional. scientific-practical Conf. (Cherkasy, May 21-
23, 2020), pages 169–170. Cherkasy State Technolog-
ical University, Cherkasy.
Oxman, S. and Wong, W. (2014). White paper: Adap-
tive learning systems. Integrated Education Solutions,
pages 6–7. https://pdfcoffee.com/qdownload/dvx-
adaptive-learning-white-paper-pdf-free.html.
Red, O. A. (2010). Intelligent adaptive distance learning
system RATOS-AI (part 2.) Conceptual model of the
system for training pharmacists. Zaporozhye Medical
Journal, (12, №4):28–36.
Ryabinova, E. N. (2009). Adaptive system of personalized
professional training of students of technical universi-
ties. Mashinostroenie, Moscow.
Shershneva, V. A., Vainshtein, Y. V., and Kochetkova, T. O.
(2018). Adaptive system of web-based teaching. Pro-
gram Systems: Theory and Applications, 9(4):179–
197.
Shute, V. J. and Zapata-Rivera, D. (2007). Adap-
tive Technologies, chapter 24. Routledge.
https://myweb.fsu.edu/vshute/pdf/shute
Siadaty, M. and Taghiyareh, F. (2007). PALS2: Pedagog-
ically Adaptive Learning System based on Learning
Styles. In Seventh IEEE International Conference
on Advanced Learning Technologies (ICALT 2007),
pages 616–618.
Silkina, N. S. and Sokolinsky, L. B. (2016). Survey of
adaptive e-learning models. Bulletin of the South Ural
State University. Series: Computational Mathematics
and Software Engineering, 5(4):61–76.
Skliarova, O. N. (2016). Adaptive automated learning sys-
tem for learning a foreign language “Aktur”: Method-
ology and requirements. In Regularities and Trends
in the Development of Science in Modern Society:
Proceedings of International Conference, volume 2,
pages 159–162, Ufa. AETERNA.
Solovov, A. V. (2010). Mathematical modeling of e-
learning content, navigation and processes in the con-
text of international standards and specifications. Re-
search Center for the Problems of the Quality of Pro-
fessional Training, Moscow.
Toktarova, V. I. and Fedorova, S. N. (2020). Theoretical
and methodological bases for designing the concept
of the adaptive system of mathematical training of stu-
dents in the conditions of electronic educational envi-
ronment of the higher educational institution. Siberian
pedagogical journal, (3):74–83.
Vlasenko, A. A. (2014). Development of an adaptive dis-
tance learning system in the field of information tech-
nology. PhD thesis.
Yasuda, K., Kawashima, H., Hata, Y., and Kimura, H.
(2015). Implementation of an adaptive learning
system using a bayesian network. In The 11th
International Conference Mobile Learning 2015,
pages 157–159. http://www.iadisportal.org/digital-
library/implementation-of-an-adaptive-learning-
system-using-a-bayesian-network.
Analysis and Summarization of the Experience of Developing Adaptive Learning Systems in Higher Education
215