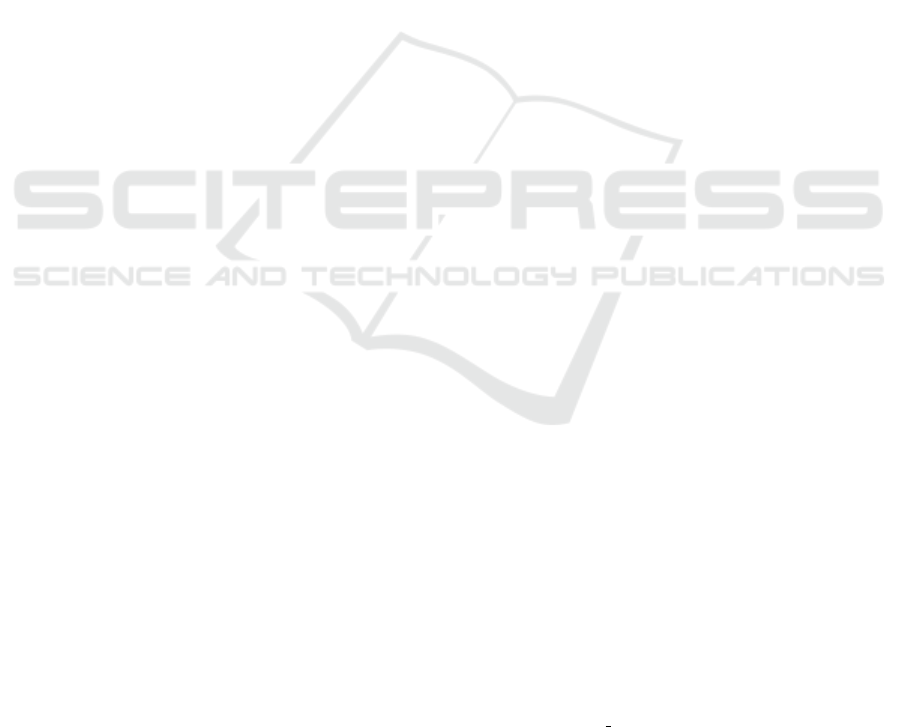
ization and problems of educational psychology. Stud-
ies in Soviet Thought, 36(3):169–202.
Elumalai, K. V., Sankar, J. P., Kalaichelvi, R., John, J. A.,
Menon, N., Alqahtani, M. S. M., and Abumelha,
M. A. (2019). Factors affecting the quality of e-
learning during the Covid-19 pandemic from the per-
spective of higher education students. Journal of In-
formation Technology Education: Research, 19:731–
753.
Erdniev, P. M. and Erdniev, B. P. (1976). The systematiza-
tion of knowledge and intensification of the learning
unit. Soviet Education, 18(5):83–97.
Ginns, P. and Ellis, R. A. (2009). Evaluating the quality of
e-learning at the degree level in the student experience
of blended learning. British Journal of Educational
Technology, 40(4):652–663.
Grushevskaya, V. Y. (2016). Methods of teaching the devel-
opment of infographics in a pedagogical university.
Pedagogical education in Russia, 7:673–685.
https://cyberleninka.ru/article/n/metodika-
obucheniya-razrabotke-infografiki-v-
pedagogicheskom-vuze.
Hay, D. B., Kehoe, C., Miquel, M. E., Hatzipana-
gos, S., Kinchin, I. M., Keevil, S. F., and Lygo-
Baker, S. (2008). Measuring the quality of e-
learning. British Journal of Educational Technology,
39(6):1037–1056.
Holub, O., Moiseienko, M., and Moiseienko, N. (2020).
Fluid flow modelling in Houdini. CEUR Workshop
Proceedings, 2732:909–917.
Iryanti, E. and Pandiya, R. (2017). Evaluating the quality of
e-learning using consistent fuzzy preference relations
method. In Proceedings of the 2016 6th International
Conference on System Engineering and Technology,
ICSET 2016, pages 61–66.
Ivanova, H. I., Lavrentieva, O. O., Eivas, L. F., Zenkovych,
I. O., and Uchitel, A. D. (2020). The students’ brain-
work intensification via the computer visualization
of study materials. CEUR Workshop Proceedings,
2643:185–209.
Kalmykova, Z. I. (1959). Dependence of knowledge as-
similation level on pupils’ activity in learning. Soviet
Education, 1(11):63–68.
Kazaine, I. (2017). Evaluating the quality of e-learning ma-
terial. Vide. Tehnologija. Resursi - Environment, Tech-
nology, Resources, 2:74–77.
Khalid, N. and Ziden, A. A. (2016). Investigating the qual-
ity of e-learning technology in relation to outcomes.
Social Sciences (Pakistan), 11(20):4882–4886.
Kravtsov, H. and Pulinets, A. (2020). Interactive aug-
mented reality technologies for model visualization in
the school textbook. CEUR Workshop Proceedings,
2732:918–933.
Leontiev, D. A., Osin, E. N., Fam, A. K., and Ovchin-
nikova, E. Y. (2020). How you choose is as
important as what you choose: Subjective quality
of choice predicts well-being and academic perfor-
mance. Current Psychology. https://doi.org/10.1007/
s12144-020-01124-1.
Little, B. (2003). “Six sigma” techniques improve the qual-
ity of e-learning. Industrial and Commercial Training,
35(3):104–108.
Lundqvist, K., Williams, S., and Baker, K. (2006). Eval-
uation of quality of e-learning environments. In
Proceedings of the European Conference on Games-
based Learning, volume 2006-January, pages 216–
223.
Lytvynova, S. G. (2013). To question of quality examina-
tion of electronic educational resources. Information
Technologies and Learning Tools, 34(2):21–27. https:
//journal.iitta.gov.ua/index.php/itlt/article/view/812.
Male, G. and Pattinson, C. (2011). Enhancing the qual-
ity of e-learning through mobile technology: A socio-
cultural and technology perspective towards quality e-
learning applications. Campus-Wide Information Sys-
tems, 28(5):331–344.
Manko, N. N. (2009). Cognitive visualization of didac-
tic objects in the activation of educational activi-
ties. Pedagogy and psychology. Proceedings of Altai
State University, 2. http://izvestia.asu.ru/2009/2/peda/
TheNewsOfASU-2009-2-peda-04.pdf.
Markovi
´
c, S. and Jovanovi
´
c, N. (2012). Learning style as a
factor which affects the quality of e-learning. Artificial
Intelligence Review, 38(4):303–312.
Marshall, S. (2012). Improving the quality of e-learning:
Lessons from the eMM. Journal of Computer Assisted
Learning, 28(1):65–78.
Mazorchuk, M. S., Vakulenko, T. S., Bychko, A. O.,
Kuzminska, O. H., and Prokhorov, O. V. (2020).
Cloud technologies and learning analytics: Web ap-
plication for PISA results analysis and visualization.
CEUR Workshop Proceedings, 2879:484–494.
Midak, L. Y., Kravets, I. V., Kuzyshyn, O. V., Baziuk,
L. V., and Buzhdyhan, K. V. (2021). Specifics of us-
ing image visualization within education of the up-
coming chemistry teachers with augmented reality
technology. Journal of Physics: Conference Series,
1840(1):012013.
Minsky, M. (2013). A framework for representing knowl-
edge. In Readings in Cognitive Science: A Perspec-
tive from Psychology and Artificial Intelligence, pages
156–289.
Movchan, V. I. (2017). Preparation of future primary
school teachers for the formation of students’ visual
thinking by means of infographics. Bulletin of the
Cherkasy Bohdan Khmelnytsky National University.
Series ‘Pedagogical Sciences’, 11.
Olefirenko, N. V. (2015). Theoretical and methodolog-
ical foundations for training primary school teach-
ers to design e-learning resources. The thesis for
doctor degree in pedagogic, speciality 13.00.04 –
the theory and methods of professional education.,
H.S. Skovoroda Kharkiv National Pedagogical Uni-
versity. https://kafinfo.org.ua/images/stories/2018-
2019/autoref onv.pdf.
Olefirenko, N. V., Kostikova, I. I., Ponomarova, N. O.,
Bilousova, L. I., and Pikilnyak, A. V. (2019). E-
learning resources for successful math teaching to
pupils of primary school. CEUR Workshop Proceed-
ings, 2433:443–458.
Training Teachers-to-Be to Create Infographics and Its Expert Evaluation
321