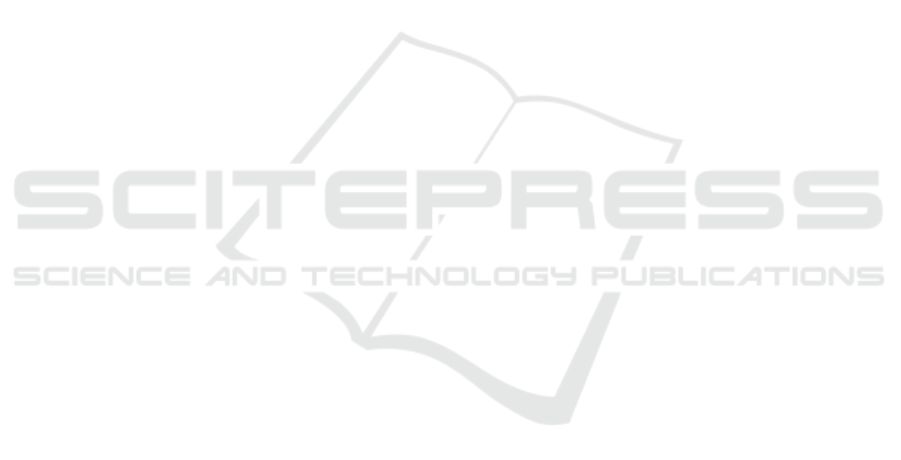
which are usually spelled out in the relevant regula-
tions. In particular, in NULES, these criteria are di-
vided into structural-functional, scientific-substantive
and methodological. But the use of certified courses
is not uniform throughout the semester. At the same
time, students actively use part of the resources in the
courses, and some resources are not used at all. In or-
der to identify the reasons for this and to select tools
for quick analysis of course performance data and its
resources, it is necessary to analyze the relevant tools,
which are built-in or complementary.
Let us focus on the statistical and analytical tools
of CLMS Moodle. Course resource efficiency indi-
cators can be obtained through the use of the embed-
ded modules: “Course Comparison” and “Statistics”
modules, as well as the optional “Analytics” module.
2.1 Features of the Module
“Comparison of Courses”
The analysis modules in the base Moodle distribution
are not very powerful, but they are present too. One
of the first modules that is appropriate to use when
analyzing courses is Course Comparison. This can be
found under Manage – Site Management – Reports.
This module allows you to view four reports:
1. The most active courses (ranks courses by the
number of actions taken by their participants as
a whole).
2. Most active courses (weighted) (calculates the av-
erage performance per user in the course).
3. Participation rate (shows courses in descending
order of user participation rate).
4. Activity ratio (determined by the ratio of involve-
ment and participation of users in the course).
Some of these concepts need clarification.
The first analytical report gives us the opportunity
to see on which course there is active activity. Activ-
ity on a course is the total number of all views and
publications on the course during the period under
study. However, this is of little use as a large num-
ber of enrolled users will visually create more activity
compared to courses with a relatively small number
of participants.
Consequently, a weighted average of each user’s
activity on the course is already more informative and
will show everyone’s participation. Here you can see
how truly active courses with a small number of users
come to the fore. However, if there are few active
users on the course, the mass of enrolled but inactive
users will drag the course down in the rankings.
The following report can clarify this nuance. It
shows how many real active students are on the
course. The participation rate of active users is calcu-
lated as the share of active users to the total enrolment
in the course. Active users are defined as those who
had had activity during the period under study. But
here again the question arises, what are these users
doing on the course? Are they just reading (receiving
information) or are they active?
The fourth report gives us the answer to this ques-
tion. The publication and views activity ratio is calcu-
lated as the share of publications in relation to views.
Where views refer to any user going to another page
and ’reading’ it or downloading a file resource from
the course to their computer. Publications are defined
as any activity the user performs on a course, for ex-
ample, completing a quiz, completing a task (down-
loading a file or writing a text response), replying to a
forum post, and so on. That is publishing is not just a
forum post.
2.2 Features of the “Statistics” Module
Another auxiliary module for analyzing course per-
formance is the Statistics module, which operates at
the site level, providing some statistics on the activity
on courses as a whole, as well as in each individual
course. At the site level, the results of this module are
available to administrators and site managers. At the
level of each course, teachers can use it to generate
statistics within that course.
About the Course Comparison module analyzing
activities without dividing into teachers and students,
it is important to say exactly who generates such ac-
tivities – the actual training of students or the active
creation of a teaching course by teachers. The Statis-
tics module brings clarification. Using this module,
we can see the activities of each individual role. It is
also possible to look separately at views, only publi-
cations, or only introductions. At a site-wide level,
these metrics plot all roles, while at a course level we
can get a separate graph for each role.
2.3 Possibilities of the “Analytics”
Module
Additional analytical reports can be obtained using
third-party modules. One such powerful addition is
the Analytics module. With its help it is possible to
analyze activity of each student both as a whole on a
course, and in each concrete resource of a course. We
are provided with such reports:
• Valuation chart (shows the distribution of valua-
tions using a stock chart)
• Work with content (shows the activities of stu-
AET 2020 - Symposium on Advances in Educational Technology
446