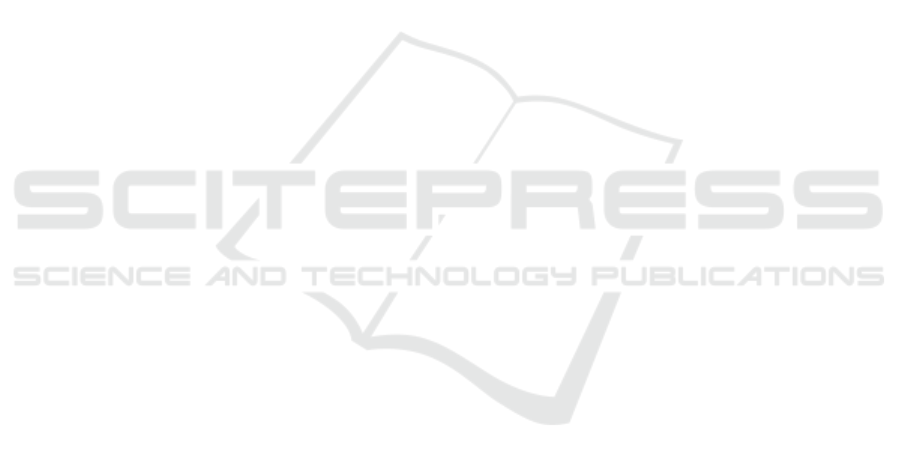
ory for warping. In Pajdla, T. and Matas, J., editors,
European Conference on Computer Vision (ECCV),
volume 3024 of LNCS, pages 25–36, Prague, Czech
Republic. Springer.
Buades, A., Lisani, J., and Miladinovi
´
c, M. (2016). Patch-
based video denoising with optical flow estimation.
IEEE Transactions on Image Processing, 25(6):2573–
2586.
Butler, D. J., Wulff, J., Stanley, G. B., and Black, M. J.
(2012). A naturalistic open source movie for optical
flow evaluation. In A. Fitzgibbon et al. (Eds.), editor,
European Conf. on Computer Vision (ECCV), Part IV,
LNCS 7577, pages 611–625. Springer-Verlag.
Ehret, T., Morel, J., and Arias, P. (2018). Non-local kalman:
A recursive video denoising algorithm. In 2018 25th
IEEE International Conference on Image Processing
(ICIP), pages 3204–3208.
Horn, B. and Schunck, B. (1981). Determining optical flow.
MIT Artificial Intelligence Laboratory, 17:185–203.
Larsson, M. and S
¨
oderstr
¨
om, L. (2015). Analysis of optical
flow algorithms for denoising. Student Paper.
Liu, C. and Freeman, W. T. (2010). A high-quality video
denoising algorithm based on reliable motion estima-
tion. In Daniilidis, K., Maragos, P., and Paragios, N.,
editors, Computer Vision – ECCV 2010, pages 706–
719, Berlin, Heidelberg. Springer Berlin Heidelberg.
Lucas, B. D. and Kanade, T. (1981). An iterative image
registration technique with an application to stereo vi-
sion. In Proceedings of the 7th international joint
conference on Artificial intelligence - Volume 2, pages
674–679, San Francisco, CA, USA. Morgan Kauf-
mann Publishers Inc.
Meinhardt-Llopis, E., S
´
anchez, J., and Kondermann, D.
(2013). Horn-Schunck Optical Flow with a Multi-
Scale Strategy. Image Processing On Line, 3:151–
172.
Monz
´
on, N., Salgado, A., and S
´
anchez, J. (2016). Regular-
ization strategies for discontinuity-preserving optical
flow methods. IEEE Transactions on Image Process-
ing, 25(4):1580–1591.
Monz
´
on, N., Salgado, A., and S
´
anchez, J. (2016). Robust
Discontinuity Preserving Optical Flow Methods. Im-
age Processing On Line, 6:165–182.
S
´
anchez, J., Meinhardt-Llopis, E., and Facciolo, G. (2013).
TV-L1 Optical Flow Estimation. Image Processing
On Line, 3:137–150.
S
´
anchez, J., Monz
´
on, N., and Salgado, A. (2013). Ro-
bust optical flow estimation. Image Processing
On Line, 2013:252–270. http://dx.doi.org/10.
5201/ipol.2013.21.
S
´
anchez, J., Salgado, A., and Monz
´
on, N. (2013). Op-
tical flow estimation with consistent spatio-temporal
coherence models. In International Conference on
Computer Vision Theory and Applications (VISAPP),
pages 366–370. Institute for Systems and Technolo-
gies of Information, Control and Communication.
S
´
anchez, J., Salgado, A., and Monz
´
on, N. (2015). Comput-
ing inverse optical flow. Pattern Recognition Letters,
52:32 – 39.
Scharr, H. and Spies, H. (2005). Accurate optical flow in
noisy image sequences using flow adapted anisotropic
diffusion. Sig. Proc.: Image Comm., 20:537–553.
Sellent, A., Kondermann, D., Simon, S. J., Baker, S.,
Dedeoglu, G., Erdler, O., Parsonage, P., Unger, C.,
and Niehsen, W. (2012). Optical flow estimation ver-
sus motion estimation.
Spies, H. and Scharr, H. (2001). Accurate optical flow in
noisy image sequences. In Proceedings Eighth IEEE
International Conference on Computer Vision. ICCV
2001, volume 1, pages 587–592 vol.1.
Verri, A. and Poggio, T. A. (1989). Motion field and opti-
cal flow: Qualitative properties. IEEE Trans. Pattern
Anal. Mach. Intell., 11:490–498.
Zach, C., Pock, T., and Bischof, H. (2007). A Duality
Based Approach for Realtime TV-L1 Optical Flow.
In Hamprecht, F. A., Schn
¨
orr, C., and J
¨
ahne, B., ed-
itors, Pattern Recognition, volume 4713 of Lecture
Notes in Computer Science, chapter 22, pages 214–
223. Springer Berlin Heidelberg, Berlin, Heidelberg.
VISAPP 2020 - 15th International Conference on Computer Vision Theory and Applications
724