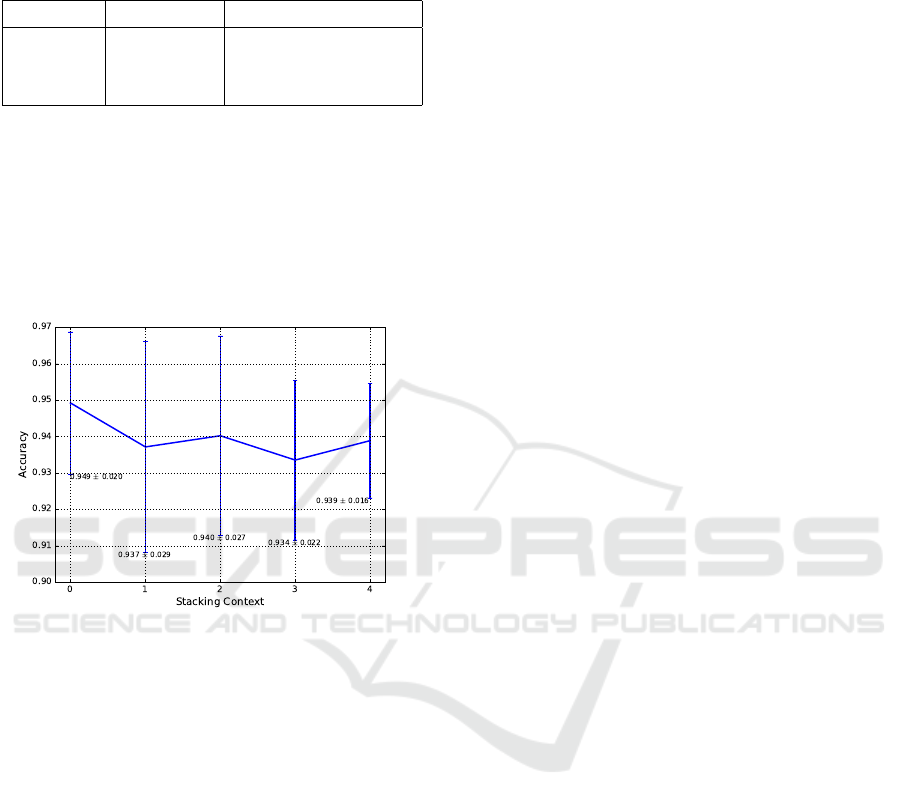
Table 3: Results of naive stacking. Window length: 10ms;
overlap: 2ms; 6 States per HMM; 7 Gaussians per state;
without feature space reduction.
Context Accuracy Vector Dimension
0 0.92 42
1 0.80 126
2 0.74 210
formance between different context sizes is not sig-
nificant as a statistical analysis via T-Test indicates.
These results are obtained on a local optimum
with a 13-dimensional feature space using solely sta-
tistical features. Therefore, we will investigate this
behavior for temporal features and further dimensions
in the future.
Figure 4: Evaluation: stacking. Setup: 10ms window
length; 2ms overlap; 6 States per HMM; 7 Gaussians per
state; 13-dimensional feature space.
7 CONCLUSION
In this paper, we successfully implemented, evaluated
and improved an offline, early fusion HAR system us-
ing a 21-dimensional biosignal comprised of different
sensors placed onto a knee bandage. The base system
performed very well with a 91% accuracy using only
simple features. We showed, that the performance
could be improved by four percentage points to 94.9%
using a LDA trained with HMM state aligned labeled
data and reducing the feature space dimension. Fur-
thermore, we found that in our case stacking feature
vectors to improve context did not increase perfor-
mance but instead slightly decreased it with respect
to not stacking at all, which is one topic for further
investigation.
In the future, we will evaluate additional more so-
phisticated features targeted to the specific sensors
and their influence on the overall performance as well
as the feature space reduction specific performance.
Furthermore, we will create and evaluate different
topologies for different activities and investigate the
performance of our system using a person indepen-
dent evaluation on a larger dataset. To the best of our
knowledge, this is the first work on feature space re-
duction in a HAR system using various biosensors in-
tegrated into a knee bandage recognizing a diverse set
of activities.
REFERENCES
Amma, C., Gehrig, D., and Schultz, T. (2010). Airwrit-
ing recognition using wearable motion sensors. In
First Augmented Human International Conference,
page 10. ACM.
Fleischer, C. and Reinicke, C. (2005). Predicting the in-
tended motion with emg signals for an exoskeleton
orthosis controller. In 2005 IEEE/RSJ International
Conference on Intelligent Robots and Systems (IROS
2005), pages 2029–2034.
Hellmers, S., Kromke, T., Dasenbrock, L., Heinks, A.,
Bauer, J. r. M., Hein, A., and Fudickar, S. (2017). Stair
Climb Power Measurements via Inertial Measurement
Units. pages 1–9.
Hu, H. and Zahorian, S. A. (2010). Dimensionality reduc-
tion methods for HMM phonetic recognition. In 2010
IEEE International Conference on Acoustics, Speech
and Signal Processing, pages 4854–4857. IEEE.
Liu, H. and Schultz, T. (2018). Ask: A framework for data
acquisition and activity recognition. In 11th Interna-
tional Conference on Bio-inspired Systems and Signal
Processing, Madeira, Portugal, pages 262–268.
Liu, H. and Schultz, T. (2019). A Wearable Real-time Hu-
man Activity Recognition System using Biosensors
Integrated into a Knee Bandage. In Proceedings of
the 12th International Joint Conference on Biomedi-
cal Engineering Systems and Technologies, pages 47–
55. SCITEPRESS - Science and Technology Publica-
tions.
Liu, X., Zhou, Z., Mai, J., and Wang, Q. (2017). Multi-
class SVM Based Real-Time Recognition of Sit-to-
Stand and Stand-to-Sit Transitions for a Bionic Knee
Exoskeleton in Transparent Mode. In The Semantic
Web - ISWC 2015, pages 262–272. Springer Interna-
tional Publishing, Cham.
Lukowicz, P., Ward, J. A., Junker, H., St
¨
ager, M., Tr
¨
oster,
G., Atrash, A., and Starner, T. (2004). Recognizing
Workshop Activity Using Body Worn Microphones
and Accelerometers. In Pervasive Computing, pages
18–32. Springer, Berlin, Heidelberg, Berlin, Heidel-
berg.
Mathie, M., Coster, A., Lovell, N., and Celler, B. (2003).
Detection of daily physical activities using a triaxial
accelerometer. In Medical and Biological Engineer-
ing and Computing. 41(3):296—301.
Mezghani, N., Fuentes, A., Gaudreault, N., Mitiche, A.,
Aissaoui, R., Hagmeister, N., and De Guise, J. A.
Feature Space Reduction for Multimodal Human Activity Recognition
139