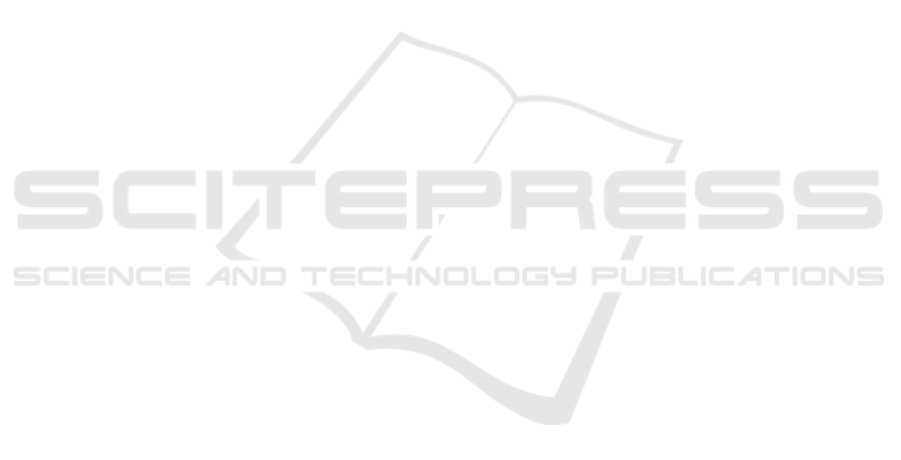
classification as we achieved 75.2 % mean accuracy
corresponding to the Kappa value of 0.67 on testing
dataset for 64 features. 72.5 % Accuracy with Kappa
value of 0.63 is achieved using 32 features only. Our
results are the best compared to all methods listed in
Table 3. Performance of ANN and Deep learning ap-
proaches can be further improved but these methods
require large amount of data and high computational
cost. Secondly, the results comparison with different
techniques which have been proposed in recent times,
has shown that the proposed method comes up with
best results for Kappa value as well as for classifica-
tion accuracy. Additionally, proposed technique gain
the dominance over other listed techniques is compu-
tational cost. We have achieved better results for less
number of features as compared to other methods.
4 CONCLUSION
A method for classifying MI signals of EEG for BCI
systems has been proposed in this paper and results
have been compared with different state of the art al-
gorithms. Proposed methodology presented in this
paper encapsulates 4 band-pass filters for preprocess-
ing. FBCSP algorithm is developed as feature ex-
traction technique to classify EEG signals for 4-class
motor imagery. Many classification algorithms have
been tested on our methodology with feature set of
different lengths where SVM has produced best clas-
sification results among all the listed approaches. Re-
sults are compared with some latest techniques of the
literature witnessing that the proposed methodology
addressed best results among all the listed techniques.
We have came with the conclusion that using a very
less number of CSP features extracted from only mu
and beta rhythms can provide a reliable feature ex-
traction source for multi class EEG data classifica-
tion. Since we have utilized the CSP features of a
limited number for classification task by a computa-
tionally very inexpensive classification technique, the
proposed algorithm can be applied for on-line classi-
fication of MI tasks in real-time BCI applications.
REFERENCES
H. Meisheri, N. Ramrao, S. K. Mitra, “Multiclass Common
Spatial Pattern for EEG based Brain Computer Inter-
face with Adaptive Learning Classifier”, ELSEVIER
Pattern Recognition Letters 2018
X. Xie, Z. L. Yu, Z. Gu, J. Zhang, L. Cen and Y. Li, ”Bi-
linear Regularized Locality Preserving Learning on
Riemannian Graph for Motor Imagery BCI,” in IEEE
Transactions on Neural Systems and Rehabilitation
Engineering, vol. 26, no. 3, pp. 698-708, March 2018.
M. Cheng, W. Jia, X. Gao, S. Gao and F. Yang, ”Mu
rhythm-based cursor control: an offine analysis,” Clin-
ical Neurophysiology, 2004.
B. Osalusi, A. Abraham, D. Aborisade, “EEG Classification
in Brain Computer Interface (BCI): A Pragmatic Ap-
praisal”, American Journal of Biomedical Engineer-
ing 2018, 8(1): 1-11
F. Lotte, L. Bougrain, A. Cichocki, M. Clerc, M. Congedo,
A. Rakotomamonjy, and F. Yger, “A review of clas-
sification algorithms for EEG-based brain–computer
interfaces: a 10 year update”, Journal of Neural Engi-
neering, 15 (2018) 031005 (28pp)
T. Nguyen, I. Hettiarachchi, A. Khatami, L. Gordon-Brown,
C. P. Lim and S. Nahavandi, ”Classification of Multi-
Class BCI Data by Common Spatial Pattern and Fuzzy
System,” in IEEE Access, vol. 6, pp. 27873-27884,
2018.
Amjed S. Al-Fahoum and Ausilah A. Al-Fraihat, ”Meth-
ods of EEG Signal Features Extraction Using Lin-
ear Analysis in Frequency and Time-Frequency Do-
mains,” ISRN Neuroscience, vol. 2014, Article ID
730218, 7 pages, 2014.
S. Kumar and F. Sahin, “A Framework for a Real Time In-
telligent and Interactive Brain Computer Interface”,
Computers and Electrical Engineering Volume 43,
April 2015.
H. Dose, J. S. Møller, S. Puthusserypady, H. K. Iversen
“A Deep Learning MI-EEG Classification Model for
BCIs”, 26th European Signal Processing Conference
(EUSIPCO) 2018
W. J. Bosl, H. Tager-Flusberg and C. A. Nelson, “EEG An-
alytics for Early Detection of Autism Spectrum Disor-
der: A data-driven approach”, Scientific Reports vol-
ume 8, Article number: 6828 (2018)
T. Nguyen, A. Khosravi, D. Creighton, S. Nahavandi
“EEG signal classification for BCI applications by
wavelets and interval type-2 fuzzy logic systems”, EL-
SEVIER, Expert Systems with Applications 42 (2015)
4370–4380
Qi Xu, H. Zhou, Y. Wang, J. Huang, ” Fuzzy support vec-
tor machine for classification of EEG signals using
wavelet-based features”, ELSEVIER Medical Engi-
neering and Physics 31 (2009) 858–865
F. Z. Baghli, Y. Lakhal, L. El Bakkali and O. Hamdoun,
”Design and simulation of Adaptive Neuro Fuzzy In-
ference System (ANFIS) controller for a robot manip-
ulator,” 2014 Second World Conference on Complex
Systems (WCCS), Agadir, 2014, pp. 298-303.
A. Mahmood, R. Zainab, R. B. Ahmad, M. Saeed and A. M.
Kamboh, ”Classification of multi-class motor imagery
EEG using four band common spatial pattern,” 2017
39th Annual International Conference of the IEEE En-
gineering in Medicine and Biology Society (EMBC),
Seogwipo, 2017, pp. 1034-1037.
M. Tangermann, K. M
¨
uller, Ad Aertsen, N. Birbaumer,
C.Braun, C. Brunner, R. Leeb, C. Mehring, K. J.
Miller, G. R. M
¨
uller-Putz, G. Nolte, G. Pfurtscheller,
H. Preissl, G. Schalk, A. Schl
¨
ogl, C. Vidaurre, S.
BIOSIGNALS 2020 - 13th International Conference on Bio-inspired Systems and Signal Processing
154