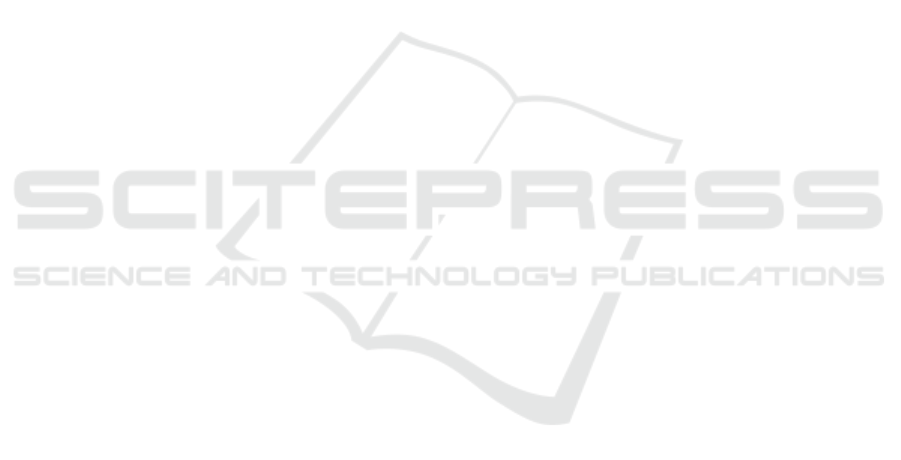
the developed world (Purcell et al., 2009; Khera et
al.,2019, 2018; Maas et al., 2016; Seibert et al.,
2018).
Because common PRS method assumes a sim-
plified genetic architecture consisting of indepen-
dent weights, understanding interactive relationships
among genes and SNPs that associate with disease
outcome remain a challenge. Existing standard multi-
variate categorical data analysis approaches fall short
in handling such enormous possible genetic inter-
action combinations with both linear and nonlinear
effects. In this context, more robust and efficient
methods towards a polygeneic risk calculation are
necessary in capturing the overlap between context-
dependent effects of both rare and common alleles on
human genetic disorder. Herein, we use the termi-
nology gene-gene (GxG) interactions to indicate any
genetic interaction including ones among SNPs that
may fall outside of coding regions.
With respect to better understanding the epista-
sis across an individual’s genome, various statistical
models have been designed with the intent of captur-
ing high dimensional GxG interactions. The Multi-
factor Dimensionality Reduction (MDR) method is
one such nonparametric framework that addresses
these challenges and has been extensively applied to
detect nonlinear complex GxG interactions associated
with individual disease (Ritchie et al., 2001; Moore
and Andrews, 2014). By isolating a specific pool
of genetic factors from all polymorphism and cross-
valiating prediction scores averaged across identified
high risk multi-locus genotypes, the original MDR
approach is able to categorize multilocus genotypes
into two groups of risk based on a threshold value.
While created with the primary intention towards
GxG interaction detection by reducing dimensional-
ity interactively in inferring genotype encodings, the
MDR model has additionally demonstrated applica-
bility as a risk score calculation model in constructing
PRS scores (Dai et al., 2013).
Modifications built on top of the MDR framework
have been proposed in order to better capture multiple
significant epistasis models and potential missed in-
teractions owning to limitations of the original model
in the higher dimensions. Model-Based Multifactor
Dimensionality Reduction (MB-MDR) was formu-
lated as a flexible GxG detection framework for both
dichotomous and continuous traits (Mahachie John et
al., 2011; Cattaert et al., 2010). Rather than a direct
comparison against a threshold level in the original
MDR method, MB-MDR merges multilocus geno-
types exhibiting significant High or Low risk levels
through association testing and adds an additional ‘No
evidence of risk’ categorization. In comparison to the
standard MDR framework which reveals at most one
optimal epistasis model, the MB-MDR method flexi-
bly weighs multiple models by producing a model list
ranked with respect to their statistical parameters.
In the present work, we aim to reformulate the
PRS leveraging the MB-MDR approach to better cap-
ture alternative encodings and epistatic interactions
of individual disease risk in a novel Multilocus Risk
Score (MRS). Through the following sections, we
briefly review the features of the MDR and MB-MDR
software, describe how our new MRS method evalu-
ates polygenic risk, and compare MRS profiling per-
formance to the standard PRS method on evidence-
based simulated dataset collections. In observing
prediction accuracy results, we demonstrate the im-
proved performance of our multi-model weighted
epistasis framework with inferred genotype encod-
ings over existing PRS methods, showing great po-
tential for more accurate identification of high risk in-
dividuals for a specific complex disease.
2 METHODS
2.1 Multifactor Dimensionality
Reduction (MDR) and Model-based
MDR (MB-MDR)
MDR is a nonparametric method that detects multi-
ple genetic loci associated with a clinical outcome
by reducing the dimension of a genotype dataset
through pooling multilocus genotypes into high-risk
and low-risk groups (Ritchie et al., 2001). MDR has
been applied to a number of real-world datasets and
sufficiently identified important variant interactions
that associated with various diseases (Motsinger and
Ritchie, 2006). Extended from the original MDR al-
gorithm, MB-MDR was first introduced in 2009 (Cat-
taert et al., 2010), and its current implementation ef-
ficiently and effectively detects multiple sets of sig-
nificant gene-gene interactions in relation to a trait of
interest while efficiently controlling type I error rates
via a cross-validation strategy. By merging multi-
locus genotypes exhibiting significant high or low risk
based on association testing rather than comparing to
an arbitrary threshold as in MDR, MB-MDR provides
a flexible framework to detect and measure epistasis.
Specifically, in addition to the test statistic and P
values associated with each genotype combination,
another important output of MB-MDR is the HLO
matrices. Briefly, in the case of a binary trait, for each
genotype combination, an HLO matrix is a 3 x 3 ma-
trix with each cell containing H (high), L (low) or O
BIOINFORMATICS 2020 - 11th International Conference on Bioinformatics Models, Methods and Algorithms
80