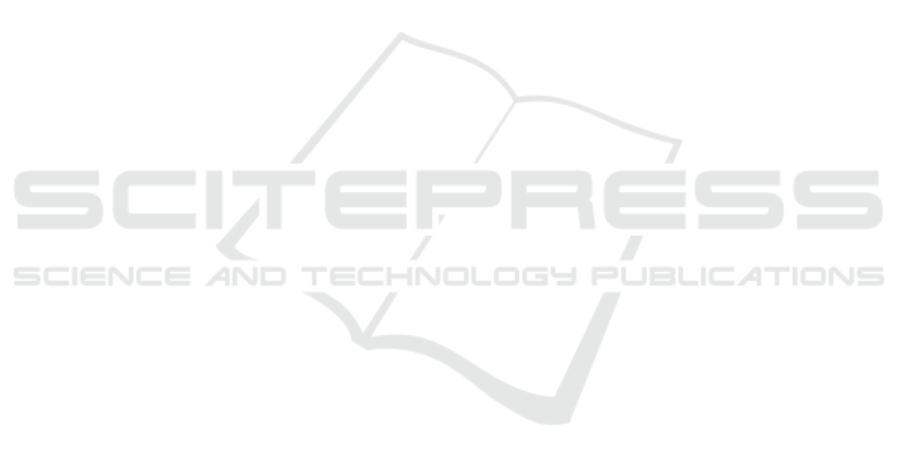
Firstly, contrarily to our hypotheses, dense trajecto-
ries are probably not the right way to describe dy-
namic textures for such kind of liquid movements.
Secondly, the extraction of such trajectories is pos-
sibly not good enough with state-of-the-art approach
and then it should be adapted. It could be for exam-
ple because of the fixed trajectory length. Indeed, we
may lose some trajectories that have length shorter or
longer than 15 frames and therefore a lot of interest-
ing spatio-temporal information may be lost during
the tracking process. This might be particularly true
in our case when the two phases of the mechanical
process are creating droplets and bubbles.
6 CONCLUSIONS
In this paper, a new dataset based on two-phased flow
visualization inside a simulation of a cooling gallery
is proposed to the community. This dataset opens a
field for research on dynamic texture analysis and es-
pecially for flow patterns extraction and analysis of
their trajectories. Here, flow patterns are used for
a classification task: two classical computer vision
techniques are studied in order to validate the fact that
there exists a correlation between the motor speed and
the movements of fluids in a two-phase flow engineer-
ing process. The first approach, based on the state-of-
the-art approach to extract and characterize trajecto-
ries, seems not to work well on fluids particles even
if there exists room for improvements. This repre-
sents a first challenging task since being able to ex-
tract and analyze these trajectories is really important
for the considered application domain: being able to
analyze and describe the behaviour of particules and
thus their trajectories could be useful for engineers.
On the other side, deep learning approaches based on
R(2+1)D convolutions give better results even if not
completely satisfying. Consequently, we propose in
this study to improve the approach adding a prepro-
cessing step that changes the original videos repre-
sentation to highlight specularities of fluids, thanks to
DoG. The counterpart of this deep method is the loss
of explanability of the decision and modeling process.
Then, another challenging task brought by this study
is how to use deep features to extract flow patterns and
their trajectories to make further analysis possible.
ACKNOWLEDGEMENTS
This work was supported by University of Zhejiang
and Haoyi Niu in particular. We gratefully acknowl-
edged the support of his work with the video data used
for this research.
REFERENCES
Andrearczyk, V. and Whelan, P. F. (2018). Convolu-
tional neural network on three orthogonal planes for
dynamic texture classification. Pattern Recognition,
76:36–49.
Crivelli, T., Cernuschi-Frias, B., Bouthemy, P., and Yao,
J.-F. (2013). Motion Textures: Modeling, Classifi-
cation, and Segmentation Using Mixed-State Markov
Random Fields. SIAM Journal on Imaging Sciences,
6(4):2484–2520.
Dalal, N. and Triggs, B. (2005). Histograms of Ori-
ented Gradients for Human Detection. In 2005 IEEE
Computer Society Conference on Computer Vision
and Pattern Recognition (CVPR’05) , volume 1, pages
886–893. IEEE.
Dalal, N., Triggs, B., Schmid, C., Dalal, N., Triggs, B.,
Schmid, C., Detection, H., and Oriented, U. (2006).
Human Detection Using Oriented Histograms of Flow
and Appearance. European Conference on Computer
Vision (ECCV), 1(1):428–441.
Farneb
¨
ack, G. and Farneb, G. (2003). Two-Frame Motion
Estimation Based on. Lecture Notes in Computer Sci-
ence, 2003(1):363–370.
Grabner, H., Grabner, M., and Bischof, H. (2006). Real-
Time Tracking via On-line Boosting. In Procedings
of the British Machine Vision Conference 2006, pages
6.1–6.10. British Machine Vision Association.
Jansson, Y. and Lindeberg, T. (2018). Dynamic Texture
Recognition Using Time-Causal and Time-Recursive
Spatio-Temporal Receptive Fields. Journal of Mathe-
matical Imaging and Vision, 60(9):1369–1398.
Jianbo Shi and Tomasi (1994). Good features to track.
In Proceedings of IEEE Conference on Computer Vi-
sion and Pattern Recognition CVPR-94, volume 169,
pages 593–600. IEEE Comput. Soc. Press.
Krizhevsky, A., Sutskever, I., and Hinton, G. E. (2017). Im-
ageNet classification with deep convolutional neural
networks. Communications of the ACM, 60(6):84–90.
Laptev, I., Marszalek, M., Schmid, C., and Rozenfeld,
B. (2008). Learning realistic human actions from
movies. In 2008 IEEE Conference on Computer Vi-
sion and Pattern Recognition, pages 1–8. IEEE.
Lowe, D. G. (2004). Distinctive image features from scale-
invariant keypoints. International Journal of Com-
puter Vision, 60(2):91–110.
Lu, Z., Xie, W., Pei, J., and Huang, J. J. (2007). Dynamic
texture recognition by spatio-temporal multiresolution
Histograms. Proceedings - IEEE Workshop on Motion
and Video Computing, MOTION 2005, (200338):241–
246.
Nelson, R. C. and Polana, R. (1992). Qualitative recogni-
tion of motion using temporal texture. CVGIP: Image
Understanding, 56(1):78–89.
Qi, X., Li, C. G., Zhao, G., Hong, X., and Pietik
¨
ainen, M.
(2016). Dynamic texture and scene classification by
transferring deep image features. Neurocomputing.
Trajectory Extraction and Deep Features for Classification of Liquid-gas Flow under the Context of Forced Oscillation
25