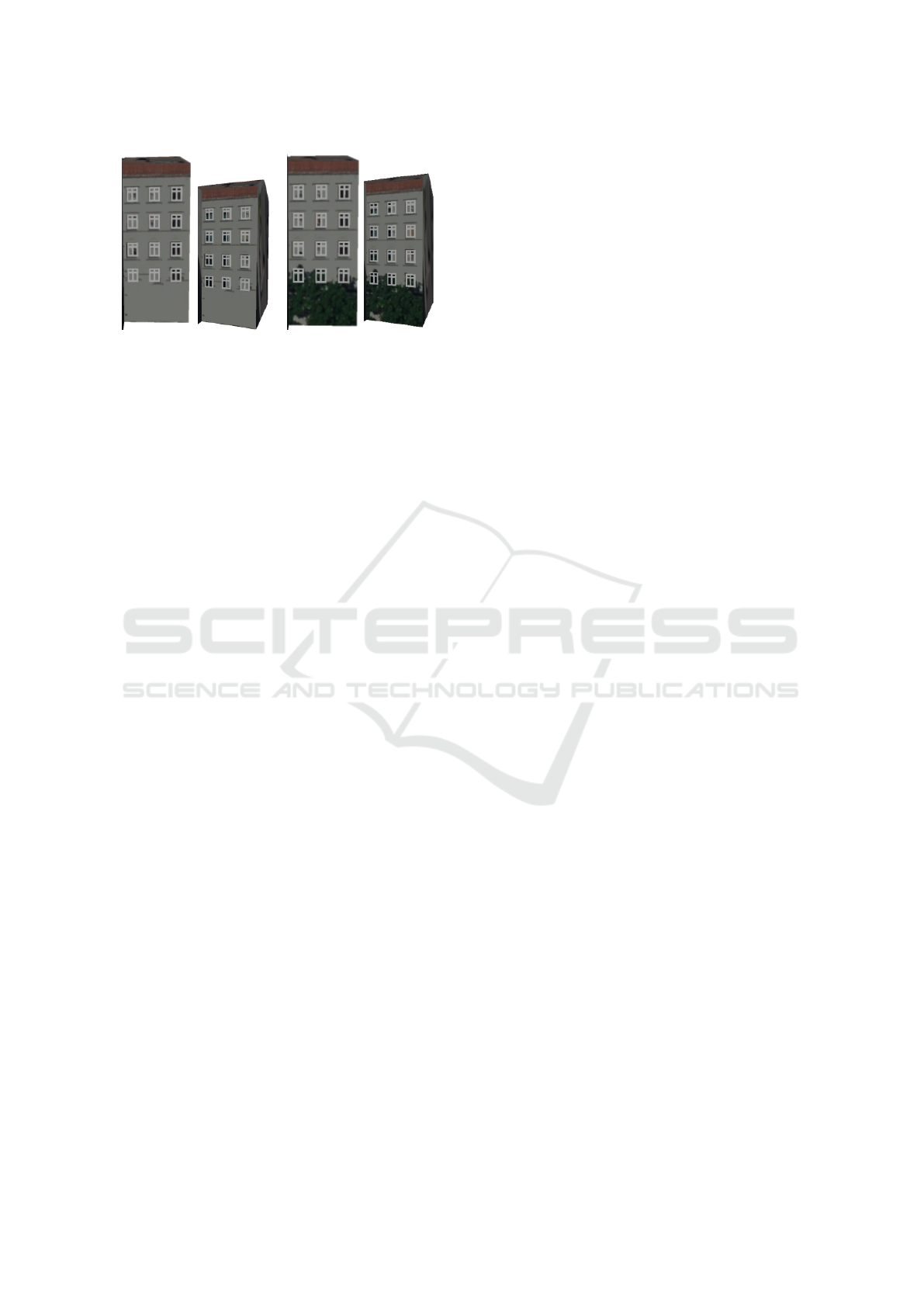
(a)
(b)
Figure 6: Example of an inpainted LoD3 model: (a) in-
painted model, (b) original model (down).
6 CONCLUSIONS
In quick response and virtual reality applications, it is
often important for the user to be able to orient him or
herself in the unknown terrain. In many applications,
not only a visually appealing model is important, but
also the trustworthiness of observed information. We
have shown that considering classification results im-
proves the inpainting result. In addition to the orig-
inal procedure of (Criminisi et al., 2004), we use an
approximated inpainting result that includes classes
straightforward; in the second step, we control the re-
sult by weighting them. Cleaned textures are partic-
ularly useful to adjust the scenario for different sea-
sons. An example is shown in Figure 1, a model was
adapted for a winter scenario, where the uncleaned
textures are particularly disturbing. Another ques-
tion is whether inpainted texture images help to im-
prove the detection results and clustering results in
turn. We compared the detection results of two pairs
of original and inpainted texture images. The compar-
ison shows that inpainting does not necessarily im-
prove the detection or clustering results. The paper
shows that patch-based methods are in no way infe-
rior to a CNN-based method and thereby, we believe
to have proved the methodological principle. Addi-
tionally, the method still have potential to exploit. For
example, we have concentrated on RGB-color space
and have performed inpainting channel-by-channel.
However, there are sources in the literature, such as
(Cao et al., 2011) that pointed out that there are color
spaces better suitable for inpainting tasks. It is also
clear that if the instances of the same class have dif-
ferent colors (for example, green, blue, and red win-
dow), then color averaging would produce colors not
present so far. Thus color averaging can be replaced
by identification of accumulation points. With respect
to CNN-based generation, it would make sense to use
the results obtained by our method as training data
in order to improve the results of Sec 5.3. Other
CNN-based workflows, including generative adver-
sarial networks, seem to be a promising tool as well.
Finally, the mask for occluding object was created
manually. This is clearly not affordable in applica-
tions and therefore, a unified concept incorporating
this step into context-aware segmentation is highly
desirable.
ACKNOWLEDGMENTS
Many thanks to the student assistant Ludwig List from
Fraunhofer IOSB for providing the NVIDIA neural
network inpainting result.
REFERENCES
Bertalmio, M., Bertozzi, A. L., and Sapiro, G. (2001).
Navier-stokes, fluid dynamics, and image and video
inpainting. In IEEE Computer Society Conference on
Computer Vision and Pattern Recognition, 2001, vol-
ume 1, pages I–355.
Bulatov, D., H
¨
aufel, G., Meidow, J., Pohl, M., Solbrig, P.,
and Wernerus, P. (2014a). Context-based automatic
reconstruction and texturing of 3D urban terrain for
quick-response tasks. ISPRS Journal of Photogram-
metry and Remote Sensing, 93:157–170.
Bulatov, D., H
¨
aufel, G., Solbrig, P., and Wernerus, P.
(2014b). Automatic representation of urban terrain
models for simulations on the example of VBS2. In
SPIE Security+ Defence, pages 925012–925012. In-
ternational Society for Optics and Photonics.
Buyssens, P., Daisy, M., Tschumperl
´
e, D., and L
´
ezoray,
O. (2015). Exemplar-based inpainting: Technical re-
view and new heuristics for better geometric recon-
structions. IEEE Transactions on image processing,
24(6):1809–1824.
Cao, F., Gousseau, Y., Masnou, S., and P
´
erez, P. (2011). Ge-
ometrically guided exemplar-based inpainting. SIAM
Journal on Imaging Sciences, 4(4):1143–1179.
Chen, L.-C., Zhu, Y., Papandreou, G., Schroff, F., and
Adam, H. (2018). Encoder-decoder with atrous sep-
arable convolution for semantic image segmentation.
In Proceedings of the European Conference on Com-
puter Vision (ECCV), pages 801–818.
Criminisi, A., P
´
erez, P., and Toyama, K. (2004). Region
filling and object removal by exemplar-based image
inpainting. IEEE Transactions on Image Processing,
13(9):1200–1212.
Efros, A. A. and Leung, T. K. (1999). Texture synthesis by
non-parametric sampling. In Proceedings of the 7th
IEEE International Conference on Computer Vision,
volume 2, pages 1033–1038.
Guo, S., Xiong, X., Liu, Z., Bai, X., and Zhou, F. (2018).
Infrared simulation of large-scale urban scene through
LOD. Optics express, 26(18):23980–24002.
Context-aware Patch-based Method for Façade Inpainting
217