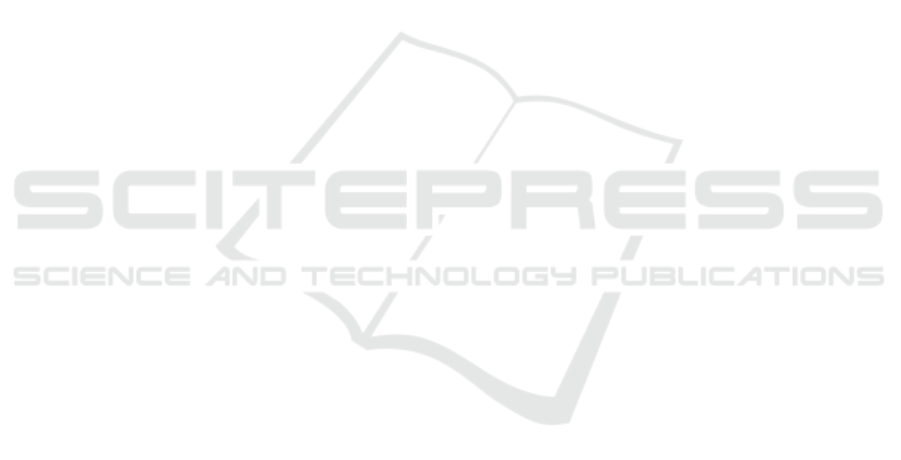
REFERENCES
Adel Bargal, S., Zunino, A., Kim, D., Zhang, J., Murino, V.,
and Sclaroff, S. (2018). Excitation backprop for rnns.
In Proceedings of the IEEE Conference on Computer
Vision and Pattern Recognition, pages 1440–1449.
Bak, C., Kocak, A., Erdem, E., and Erdem, A. (2018). Spatio-
temporal saliency networks for dynamic saliency pre-
diction. IEEE Transactions on Multimedia, 20(7):1688–
1698.
Borji, A. (2018). Saliency prediction in the deep learn-
ing era: An empirical investigation. arXiv preprint
arXiv:1810.03716.
Borji, A. and Itti, L. (2013). State-of-the-art in visual atten-
tion modeling. IEEE transactions on pattern analysis
and machine intelligence, 35(1):185–207.
Borji, A. and Itti, L. (2015). Cat2000: A large scale fixation
dataset for boosting saliency research. arXiv preprint
arXiv:1505.03581.
Bruce, N. and Tsotsos, J. (2006). Saliency based on informa-
tion maximization. In Advances in neural information
processing systems, pages 155–162.
Bylinskii, Z., Judd, T., Borji, A., Itti, L., Durand, F., Oliva,
A., and Torralba, A. Mit saliency benchmark.
Cornia, M., Baraldi, L., Serra, G., and Cucchiara, R. (2016).
A deep multi-level network for saliency prediction. In
2016 23rd International Conference on Pattern Recog-
nition (ICPR), pages 3488–3493. IEEE.
Deng, J., Dong, W., Socher, R., Li, L.-J., Li, K., and Fei-Fei,
L. (2009). Imagenet: A large-scale hierarchical image
database. In 2009 IEEE conference on computer vision
and pattern recognition, pages 248–255. Ieee.
Gao, D. and Vasconcelos, N. (2005). Discriminant saliency
for visual recognition from cluttered scenes. In Ad-
vances in neural information processing systems, pages
481–488.
Garcia-Diaz, A., Fdez-Vidal, X. R., Pardo, X. M., and Dosil,
R. (2009). Decorrelation and distinctiveness provide
with human-like saliency. In International Conference
on Advanced Concepts for Intelligent Vision Systems,
pages 343–354. Springer.
Garcia-Diaz, A., Fdez-Vidal, X. R., Pardo, X. M., and
Dosil, R. (2012). Saliency from hierarchical adapta-
tion through decorrelation and variance normalization.
Image and Vision Computing, 30(1):51–64.
Goferman, S., Zelnik-Manor, L., and Tal, A. (2012). Context-
aware saliency detection. IEEE transactions on pattern
analysis and machine intelligence, 34(10):1915–1926.
Goodfellow, I., Pouget-Abadie, J., Mirza, M., Xu, B., Warde-
Farley, D., Ozair, S., Courville, A., and Bengio, Y.
(2014). Generative adversarial nets. In Advances in
neural information processing systems, pages 2672–
2680.
Guo, C., Ma, Q., and Zhang, L. (2008). Spatio-temporal
saliency detection using phase spectrum of quaternion
fourier transform. In 2008 IEEE Conference on Com-
puter Vision and Pattern Recognition, pages 1–8. IEEE.
Hochreiter, S. and Schmidhuber, J. (1997). Long short-term
memory. Neural computation, 9(8):1735–1780.
Hossein Khatoonabadi, S., Vasconcelos, N., Bajic, I. V., and
Shan, Y. (2015). How many bits does it take for a
stimulus to be salient? In Proceedings of the IEEE
Conference on Computer Vision and Pattern Recogni-
tion, pages 5501–5510.
Huang, X., Shen, C., Boix, X., and Zhao, Q. (2015). Salicon:
Reducing the semantic gap in saliency prediction by
adapting deep neural networks. In Proceedings of the
IEEE International Conference on Computer Vision,
pages 262–270.
Itti, L., Koch, C., and Niebur, E. (1998). A model of saliency-
based visual attention for rapid scene analysis. IEEE
Transactions on Pattern Analysis & Machine Intelli-
gence, (11):1254–1259.
Ji, S., Xu, W., Yang, M., and Yu, K. (2013). 3d convolu-
tional neural networks for human action recognition.
IEEE transactions on pattern analysis and machine
intelligence, 35(1):221–231.
Jiang, L., Xu, M., Liu, T., Qiao, M., and Wang, Z. (2018a).
Deepvs: A deep learning based video saliency pre-
diction approach. In The European Conference on
Computer Vision (ECCV).
Jiang, L., Xu, M., Liu, T., Qiao, M., and Wang, Z. (2018b).
Deepvs: A deep learning based video saliency predic-
tion approach. In Proceedings of the European Confer-
ence on Computer Vision (ECCV), pages 602–617.
Jiang, L., Xu, M., and Wang, Z. (2017). Predicting video
saliency with object-to-motion cnn and two-layer con-
volutional lstm. arXiv preprint arXiv:1709.06316.
Jiang, M., Huang, S., Duan, J., and Zhao, Q. (2015). Sali-
con: Saliency in context. In The IEEE Conference on
Computer Vision and Pattern Recognition (CVPR).
Judd, T., Durand, F., and Torralba, A. (2012). A benchmark
of computational models of saliency to predict human
fixations.
Kingma, D. P. and Ba, J. (2014). Adam: A
method for stochastic optimization. arXiv preprint
arXiv:1412.6980.
Kruthiventi, S. S., Ayush, K., and Babu, R. V. (2017). Deep-
fix: A fully convolutional neural network for predicting
human eye fixations. IEEE Transactions on Image Pro-
cessing, 26(9):4446–4456.
K
¨
ummerer, M., Theis, L., and Bethge, M. (2014). Deep
gaze i: Boosting saliency prediction with feature maps
trained on imagenet. arXiv preprint arXiv:1411.1045.
Leboran, V., Garcia-Diaz, A., Fdez-Vidal, X. R., and Pardo,
X. M. (2017). Dynamic whitening saliency. IEEE
transactions on pattern analysis and machine intelli-
gence, 39(5):893–907.
Leifman, G., Rudoy, D., Swedish, T., Bayro-Corrochano,
E., and Raskar, R. (2017). Learning gaze transitions
from depth to improve video saliency estimation. In
Proceedings of the IEEE International Conference on
Computer Vision, pages 1698–1707.
Li, X., Zhao, L., Wei, L., Yang, M.-H., Wu, F., Zhuang,
Y., Ling, H., and Wang, J. (2016). Deepsaliency:
Multi-task deep neural network model for salient object
detection. IEEE Transactions on Image Processing,
25(8):3919–3930.
3DSAL: An Efficient 3D-CNN Architecture for Video Saliency Prediction
35