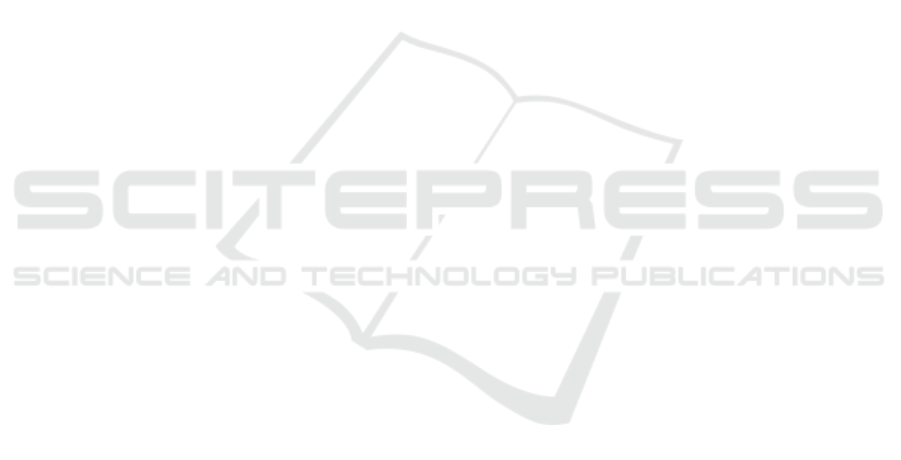
5 CONCLUSIONS
We proposed a method for improving the accuracy of
gender classification using the gaze distribution of hu-
man observers on training images in which the pri-
vacy of the subjects was protected. We used stimu-
lus images with masked head regions and measured
the gaze distributions of observers. We confirmed
that the participants mainly observed the torso re-
gions of the subjects in the stimulus images. Next,
we conducted gender classification experiments us-
ing privacy-protected training images with masking,
pixelization, and blur. The experimental results con-
firm that our method, which uses the gaze map with
masked head regions, improved the accuracy of gen-
der classification. In future work, we intend to con-
tinue developing the method to increase the accuracy
by combining gaze maps with and without masking.
We will expand this investigation into gaze maps with
privacy protection for various classification tasks re-
lated to attributes other than gender. This work was
partially supported by JSPS KAKENHI under grant
number JP17K00238 and MIC SCOPE under grant
number 172308003.
REFERENCES
Campisi, P. (2013). Security and privacy in biometrics: To-
wards a holistic approach. Security and Privacy in
Biometrics, Springer, pages 1–23.
Corinna, C. and Vladimir, V. (1995). Support-vector net-
works. Machine Learning, 20(3):273–297.
Cox, D. R. (1958). The regression analysis of binary se-
quences. Journal of the Royal Statistical Society. Se-
ries B (Methodological), 20(2):215–242.
Fathi, A., Li, Y., and Rehg, J. (2012). Learning to recognize
daily actions using gaze. In Proceedings of 12th Euro-
pean Conference on Computer Vision, pages 314–327.
Flammini, F., Setola, R., and Franceschetti, G. (2013). Ef-
fective surveillance for homeland security: Balancing
technology and social issues. CRC Press.
Grigory, A., Sid-Ahmed, B., Natacha, R., and Jean-Luc, D.
(2015). Learned vs. hand-crafted features for pedes-
trian gender recognition. In Proceedings of 23rd ACM
International Conference on Multimedia, pages 1263–
1266.
Joon, O. S., Rodrigo, B., Mario, F., and Bernt, S. (2016).
Faceless person recognition: Privacy implications in
social media. In Proceedings of European Conference
on Computer Vision, pages 19–35.
Karessli, N., Akata, Z., Schiele, B., and Bulling, A. (2017).
Gaze embeddings for zero-shot image classification.
In Proceedings of IEEE conference on computer vi-
sion and pattern recognition, pages 4525–4534.
Murrugarra-Llerena, N. and Kovashka, A. (2017). Learning
attributes from human gaze. In Proceedings of IEEE
Winter Conference on Applications of Computer Vi-
sion, pages 510–519.
Nishiyama, M., Matsumoto, R., Yoshimura, H., and Iwai,
Y. (2018). Extracting discriminative features using
task-oriented gaze maps measured from observers for
personal attribute classification. Pattern Recognition
Letters, 112:241 – 248.
Oh, S. J., Fritz, M., and Schiele, B. (2017). Adversarial
image perturbation for privacy protection a game the-
ory perspective. In Proceedings of IEEE International
Conference on Computer Vision, pages 1491–1500.
Ribaric, S., Ariyaeeinia, A., and Pavesic, N. (2016). De-
identification for privacy protection in multimedia
content. Image Communication, 47(C):131–151.
Ruchaud, N., Antipov, G., Korshunov, P., Dugelay, J. L.,
Ebrahimi, T., and Berrani, S. A. (2015). The impact
of privacy protection filters on gender recognition. Ap-
plications of Digital Image Processing XXXVIII, page
959906.
Sattar, H., Bulling, A., and Fritz, M. (2017). Predicting
the category and attributes of visual search targets us-
ing deep gaze pooling. In Proceedings of IEEE Inter-
national Conference on Computer Vision Workshops,
pages 2740–2748.
Schumann, A. and Stiefelhagen, R. (2017). Per-
son re-identification by deep learning attribute-
complementary information. In Proceedings of IEEE
Conference on Computer Vision and Pattern Recogni-
tion Workshops, pages 1435–1443.
Sudowe, P., Spitzer, H., and LeibeSudowe, B. (2015). Per-
son attribute recognition with a jointly-trained holis-
tic cnn model. In Proceedings of IEEE International
Conference on Computer Vision Workshop, pages
329–337.
Sugano, Y., Ozaki, Y., Kasai, H., Ogaki, K., and Sato,
Y. (2014). Image preference estimation with a
data-driven approach: A comparative study between
gaze and image features. Eye Movement Research,
7(3):862–875.
Xu, M., Ren, Y., and Wang, Z. (2015). Learning to pre-
dict saliency on face images. In Proceedings of IEEE
International Conference on Computer Vision, pages
3907–3915.
Yamada, T., Gohshi, S., and Echizen, I. (2013). Privacy vi-
sor: Method for preventing face image detection by
using differences in human and device sensitivity. In
Proceedings of International Conference on Commu-
nications and Multimedia Security, pages 152–161.
Yubin, D., Ping, L., Change, L. C., and Xiaoou, T. (2014).
Pedestrian attribute recognition at far distance. In Pro-
ceedings of 22nd ACM International Conference on
Multimedia, pages 789–792.
Zhang, Y., Lu, Y., Nagahara, H., and Taniguchi, R. (2014).
Anonymous camera for privacy protection. In Pro-
ceedings of 22nd International Conference on Pattern
Recognition, pages 4170–4175.
Zhao, Q., Chang, S., Harper, F. M., and J. A. Konstan, J.
(2016). Gaze prediction for recommender systems.
In Proceedings of 10th ACM Conference on Recom-
mender Systems, pages 131–138.
VISAPP 2020 - 15th International Conference on Computer Vision Theory and Applications
156