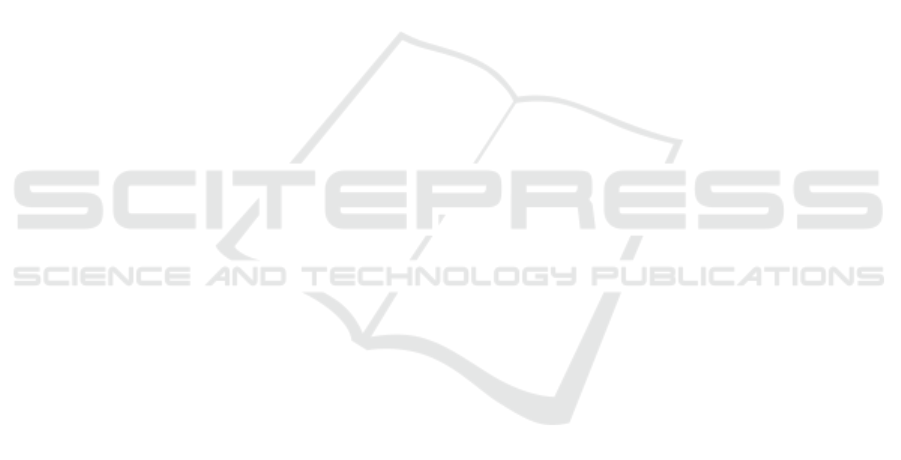
Huang, T. S. and Zeng, Z. (2007). Audio-visual affec-
tive expression recognition. In MIPPR 2007: Pattern
Recognition and Computer Vision, volume 6788, page
678802. International Society for Optics and Photon-
ics.
Jaiswal, M., Tabibu, S., and Bajpai, R. (2016). The truth and
nothing but the truth: Multimodal analysis for decep-
tion detection. In 2016 IEEE 16th International Con-
ference on Data Mining Workshops (ICDMW), pages
938–943. IEEE.
Jensen, M. L., Meservy, T. O., Burgoon, J. K., and Nuna-
maker, J. F. (2010). Automatic, multimodal evaluation
of human interaction. Group Decision and Negotia-
tion, 19(4):367–389.
Jimenez, L. O., Landgrebe, D. A., et al. (1998a). Supervised
classification in high-dimensional space: geometrical,
statistical, and asymptotical properties of multivariate
data. IEEE Transactions on Systems, Man, and Cyber-
netics, Part C: Applications and Reviews, 28(1):39–
54.
Jimenez, L. O., Landgrebe, D. A., et al. (1998b). Supervised
classification in high-dimensional space: geometrical,
statistical, and asymptotical properties of multivariate
data. IEEE Transactions on Systems, Man, and Cyber-
netics, Part C: Applications and Reviews, 28(1):39–
54.
Kawulok, M., Celebi, E., and Smolka, B. (2016). Advances
in face detection and facial image analysis. Springer.
Kirchh
¨
ubel, C. and Howard, D. M. (2013). Detecting sus-
picious behaviour using speech: Acoustic correlates
of deceptive speech–an exploratory investigation. Ap-
plied ergonomics, 44(5):694–702.
Kleinberg, B., Arntz, A., and Verschuere, B. (2019). Being
accurate about accuracy in verbal deception detection.
PloS one, 14(8):e0220228.
Krishnamurthy, G., Majumder, N., Poria, S., and Cam-
bria, E. (2018). A deep learning approach for
multimodal deception detection. arXiv preprint
arXiv:1803.00344.
Kulis, B. et al. (2013). Metric learning: A survey. Founda-
tions and Trends
R
in Machine Learning, 5(4):287–
364.
Levitan, S. I., An, G., Ma, M., Levitan, R., Rosenberg,
A., and Hirschberg, J. (2016). Combining acoustic-
prosodic, lexical, and phonotactic features for auto-
matic deception detection. In INTERSPEECH, pages
2006–2010.
Levitan, S. I., Xiang, J., and Hirschberg, J. (2018).
Acoustic-prosodic and lexical entrainment in decep-
tive dialogue. In Proc. 9th International Conference
on Speech Prosody, pages 532–536.
Lu, S., Tsechpenakis, G., Metaxas, D. N., Jensen, M. L.,
and Kruse, J. (2005). Blob analysis of the head and
hands: A method for deception detection. In Proceed-
ings of the 38th Annual Hawaii International Confer-
ence on System Sciences, pages 20c–20c. IEEE.
Maschke, G. W. and Scalabrini, G. J. (2005). The lie behind
the lie detector. Antipolygraph. org.
Meservy, T. O., Jensen, M. L., Kruse, J., Burgoon, J. K.,
Nunamaker, J. F., Twitchell, D. P., Tsechpenakis, G.,
and Metaxas, D. N. (2005). Deception detection
through automatic, unobtrusive analysis of nonverbal
behavior. IEEE Intelligent Systems, 20(5):36–43.
Mihalcea, R. and Strapparava, C. (2009). The lie detector:
Explorations in the automatic recognition of deceptive
language. In Proceedings of the ACL-IJCNLP 2009
Conference Short Papers, pages 309–312. Association
for Computational Linguistics.
Owayjan, M., Kashour, A., Al Haddad, N., Fadel, M., and
Al Souki, G. (2012). The design and development of
a lie detection system using facial micro-expressions.
In 2012 2nd international conference on advances
in computational tools for engineering applications
(ACTEA), pages 33–38. IEEE.
P
´
erez-Rosas, V., Abouelenien, M., Mihalcea, R., and
Burzo, M. (2015a). Deception detection using real-
life trial data. In Proceedings of the 2015 ACM on
International Conference on Multimodal Interaction,
pages 59–66. ACM.
P
´
erez-Rosas, V., Abouelenien, M., Mihalcea, R., Xiao, Y.,
Linton, C., and Burzo, M. (2015b). Verbal and nonver-
bal clues for real-life deception detection. In Proceed-
ings of the 2015 Conference on Empirical Methods in
Natural Language Processing, pages 2336–2346.
Sharma, R., Pavlovi
´
c, V. I., and Huang, T. S. (2002).
Toward multimodal human-computer interface. In
Advances In Image Processing And Understanding:
A Festschrift for Thomas S Huang, pages 349–365.
World Scientific.
Sondhi, S., Vijay, R., Khan, M., and Salhan, A. K. (2016).
Voice analysis for detection of deception. In 2016 11th
International Conference on Knowledge, Information
and Creativity Support Systems (KICSS), pages 1–6.
IEEE.
Talkin, D. (1995). A robust algorithm for pitch tracking
(rapt). Speech coding and synthesis, 495:518.
Toma, C. L. and Hancock, J. T. (2010). Reading between
the lines: linguistic cues to deception in online dating
profiles. In Proceedings of the 2010 ACM conference
on Computer supported cooperative work, pages 5–8.
ACM.
Ververidis, D., Kotropoulos, C., and Pitas, I. (2004). Auto-
matic emotional speech classification. In 2004 IEEE
International Conference on Acoustics, Speech, and
Signal Processing, volume 1, pages I–593. IEEE.
Vrij, A., Edward, K., Roberts, K. P., and Bull, R. (2000).
Detecting deceit via analysis of verbal and nonverbal
behavior. Journal of Nonverbal behavior, 24(4):239–
263.
Vrij, A. and Semin, G. R. (1996). Lie experts’ beliefs about
nonverbal indicators of deception. Journal of nonver-
bal behavior, 20(1):65–80.
YUZSEVER, S. (2015). MUTUAL INFORMATION
BASED FEATURE SELECTION FOR ACOUSTIC
AUTISM DIAGNOSIS. PhD thesis, Bogazic¸i Univer-
sity.
Zeng, Z., Pantic, M., and Huang, T. S. (2009). Emo-
tion recognition based on multimodal information.
In Affective Information Processing, pages 241–265.
Springer.
Zhou, L., Burgoon, J. K., Nunamaker, J. F., and Twitchell,
D. (2004). Automating linguistics-based cues
for detecting deception in text-based asynchronous
computer-mediated communications. Group decision
and negotiation, 13(1):81–106.
An Audio-Visual based Feature Level Fusion Approach Applied to Deception Detection
205