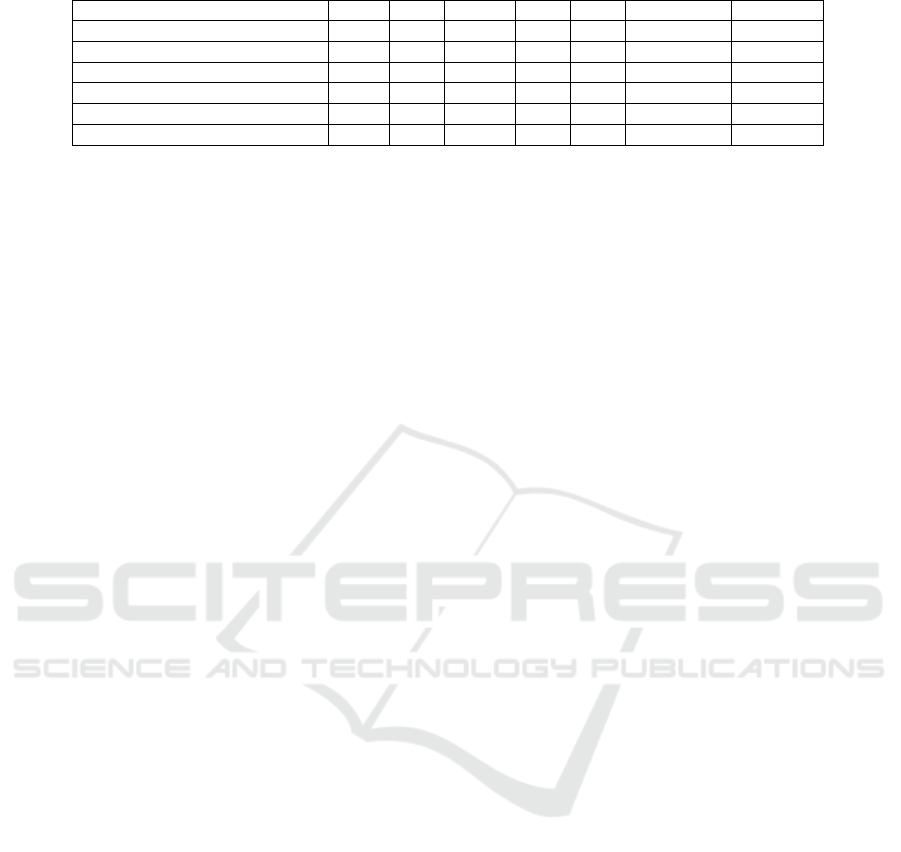
Table 5: Mean Average Precision and Average precision per object category, tested on our dataset.
Trainset mAp Car Truck Bus Van S.U. Truck A. Truck
DETRAC 27.1 76.4 15.6 53.3 14.9 1.1 1.5
MIO 47.4 78.3 60.7 70.1 4.0 16.4 54.9
DETRAC + MIO 46.1 81.9 51.1 68.5 26.6 12.0 36.3
DETRAC + MIO + Background 49.7 82.5 62.6 69.6 19.4 13.5 50.4
Ours 77.2 95.5 97.7 85.6 2.9 84.8 96.7
DETRAC + MIO + Ours 78.6 94.5 93.4 84.4 62.2 48.3 88.7
that our dataset and the larger UA-DETRAC dataset
result in similar detection performance, implying that
both sets contain sufficient information to train a de-
tector with similar high performance for localization.
In a second experiment, we have investigated the
effect of incrementally adding more datasets and have
shown that the best performance is obtained when
combining all datasets for training. Although the
MIO-TCD dataset has very different viewpoints, im-
age quality and lens distortion, it offers a large varia-
tion in the data with a high number of labels, so that it
still contributes visually to the detection of the other
viewpoints. The final system obtains a detection per-
formance of 96.4% average precision, improving with
5.9% over the original SSD implementation mainly
caused by our hard-negative mining.
Finally, we have measured the classification per-
formance of our hierarchical system. The effect of
incrementally adding more datasets reveals that the
best performance is obtained when training with all
datasets combined. By adding hierarchical classifica-
tion, the average classification performance increases
with 1.4% to 78.6% mAP. This positive result is based
on combining all datasets, although label inconsisten-
cies occur in the additional training data. Note that the
overall detection performance drops 0.8% in this case.
Since vans are not labeled as such in our dataset, we
have additionally trained our classifier for vans with
labels from the UA-DETRAC and MIO-TCD dataset.
The resulting detector obtained a decent classification
performance of 62.2% for vans, on our separate test
set. We have shown that non-labeled object classes
in actually existing datasets can be learned using ex-
ternal datasets providing the labels for at least those
classes, while simultaneously also improving the lo-
calization performance.
REFERENCES
Everingham, M., Van Gool, L., Williams, C. K. I., Winn,
J., and Zisserman, A. (2012). The PASCAL Visual
Object Classes Challenge 2012 (VOC2012) Results.
Fan, Q., Brown, L., and Smith, J. (2016). A closer look
at faster r-cnn for vehicle detection. In 2016 IEEE
Intelligent Vehicles Symposium (IV), pages 124–129.
Girshick, R. (2015). Fast R-CNN. In ICCV.
Girshick, R., Donahue, J., Darrell, T., and Malik, J. (2014).
Rich feature hierarchies for accurate object detection
and semantic segmentation. In IEEE CVPR.
He, K., Gkioxari, G., Doll
´
ar, P., and Girshick, R. (2017).
Mask R-CNN. In ICCV.
Lin, T.-Y. et al. (2014). Microsoft COCO: Common objects
in context. In ECCV, pages 740–755. Springer.
Liu, W., Anguelov, D., Erhan, D., Szegedy, C., Reed, S.,
Fu, C.-Y., and Berg, A. C. (2016). Ssd: Single shot
multibox detector. In ECCV, pages 21–37. Springer.
Luo, Z. et al. (2018). MIO-TCD: A new benchmark dataset
for vehicle classification and localization. IEEE Trans.
Image Processing, 27(10):5129–5141.
Lyu., S. et al. (2018). UA-DETRAC 2018: Report
of AVSS2018 IWT4S challenge on advanced traffic
monitoring. In 2018 15th IEEE International Con-
ference on Advanced Video and Signal Based Surveil-
lance (AVSS), pages 1–6.
Redmon, J., Divvala, S., Girshick, R., and Farhadi, A.
(2016). You only look once: Unified, real-time ob-
ject detection. In CVPR, pages 779–788.
Redmon, J. and Farhadi, A. (2017). Yolo9000: Better,
faster, stronger. In CVPR, pages 6517–6525.
Ren, S., He, K., Girshick, R., and Sun, J. (2015). Faster R-
CNN: Towards real-time object detection with region
proposal networks. In NIPS.
Russakovsky, O. et al. (2015). ImageNet Large Scale Vi-
sual Recognition Challenge. International Journal of
Computer Vision (IJCV), 115(3):211–252.
Simonyan, K. and Zisserman, A. (2014). Very deep con-
volutional networks for large-scale image recognition.
arXiv preprint arXiv:1409.1556.
Sivaraman, S. and Trivedi, M. M. (2013). Looking at vehi-
cles on the road: A survey of vision-based vehicle de-
tection, tracking, and behavior analysis. IEEE Trans.
Intelligent Transportation Systems, 14(4):1773–1795.
Tian, Z., Shen, C., Chen, H., and He, T. (2019). FCOS:
Fully convolutional one-stage object detection. In
ICCV.
Wen, L., Du, D., Cai, Z., Lei, Z., Chang, M., Qi, H., Lim,
J., Yang, M., and Lyu, S. (2015). UA-DETRAC: A
new benchmark and protocol for multi-object detec-
tion and tracking. arXiv CoRR, abs/1511.04136.
SSD-ML: Hierarchical Object Classification for Traffic Surveillance
259