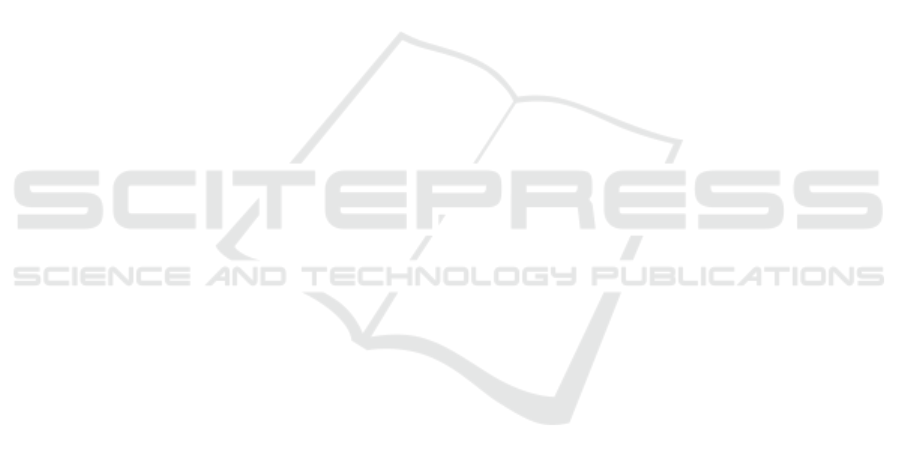
the position is detected, reassignment of the chan-
nels can be used to maintain the order of the fea-
tures without rotating the wristband.
• Arm movement: motion artifacts are the other ma-
jor problem in NIR systems. The motion of the
hand (arm rotation, elongation, elevation) causes
blood movement and muscle/tendon movement.
These movements cause an unwanted change in
the signal. It is difficult to solve this problem en-
tirely by including the arm movement information
in the study or machine learning training proce-
dures. However, it can be reduced by training
the different arm rotations and elevations for the
same gesture. An additional sensors output such
as IMU orientation data can also be used to com-
pensate for the arm rotation problem.
• Skin-sensor Coupling: Even though NIR wrist-
band does not suffer from any electrical sensor-
skin coupling unlike EMG and EIT techniques,
They still suffer from problems caused by me-
chanical coupling between the sensor and the
skin. This is mainly because light can be reflected
directly from the skin without entering the skin
tissue, thereby causing the saturation of photo-
diodes. This problem can be reduced by using an
appropriate mechanical wristband design which
can keep the coupling constant.
• IR interference: IR light from external IR sources,
e.g., The sun, IR illuminating cameras adds noise
on the main signal. In order to remove these
noises, enclosing the outer part of the wristband
with IR block film can be a potential solution. The
other solution can be a differential measurement
of the external IR and subtraction from the main
signal.
7 CONCLUSION
This paper presents a wrist-worn gesture sensing sys-
tem that consists of an array of an Infrared Emitter and
Photo-Receivers that are used to detect gestures by
measuring reflected and refracted light from tissues in
or under the skin. In this study, we have demonstrated
that Near-infrared wristbands can offer a low-cost and
high accuracy gesture sensing possibility. With the
advancement of ultra-miniature SMD IR emitters and
receivers, these techniques can be easily integrated
to wrist-worn devices such as smart-watches and Fit-
bit monitors. The system’s software consists of data
acquisition, preprocessing and classification stages.
Thirteen classes of gestures were analyzed to vali-
date the accuracy of the classification algorithm. Fu-
ture works include data collection and processing for
cross-session and cross-user performance. Integration
with orientation sensors and pressure sensors should
be investigated as a potential solution to enable recog-
nition of arm rotation and pressure distribution around
the wrist. Development of embedded data acquisition
and wireless data transfer methods can also be imple-
mented for entirely wearable and wireless wristband
gesture sensing system.
REFERENCES
Benalc
´
azar, M. E., Jaramillo, A. G., Zea, A., P
´
aez, A., An-
daluz, V. H., et al. (2017). Hand gesture recogni-
tion using machine learning and the myo armband. In
Signal Processing Conference (EUSIPCO), 2017 25th
European, pages 1040–1044. IEEE.
Boyali, A. and Hashimoto, N. (2016). Spectral collaborative
representation based classification for hand gestures
recognition on electromyography signals. Biomedical
Signal Processing and Control, 24:11–18.
Chaiken, J., Deng, B., Goodisman, J., Shaheen, G.,
and Bussjaeger, R. J. (2011). Analyzing near-
infrared scattering from human skin to monitor
changes in hematocrit. Journal of biomedical optics,
16(9):097005.
Colac¸o, A., Kirmani, A., Yang, H. S., Gong, N.-W.,
Schmandt, C., and Goyal, V. K. (2013). Mime: com-
pact, low power 3d gesture sensing for interaction
with head mounted displays. In Proceedings of the
26th annual ACM symposium on User interface soft-
ware and technology, pages 227–236. ACM.
Dementyev, A. and Paradiso, J. A. (2014). Wristflex: low-
power gesture input with wrist-worn pressure sensors.
In Proceedings of the 27th annual ACM symposium on
User interface software and technology, pages 161–
166. ACM.
Freeman, W. T. and Weissman, C. D. (1997). Hand gesture
machine control system. US Patent 5,594,469.
Fukui, R., Watanabe, M., Shimosaka, M., and Sato, T.
(2014). Hand-shape classification with a wrist contour
sensor: Analyses of feature types, resemblance be-
tween subjects, and data variation with pronation an-
gle. The International Journal of Robotics Research,
33(4):658–671.
Huang, Y., Guo, W., Liu, J., He, J., Xia, H., Sheng, X.,
Wang, H., Feng, X., and Shull, P. B. (2015). Prelim-
inary testing of a hand gesture recognition wristband
based on emg and inertial sensor fusion. In Interna-
tional Conference on Intelligent Robotics and Appli-
cations, pages 359–367. Springer.
Izzetoglu, M., Bunce, S. C., Izzetoglu, K., Onaral, B., and
Pourrezaei, K. (2007). Functional brain imaging us-
ing near-infrared technology. IEEE Engineering in
Medicine and Biology Magazine, 26(4):38.
Kerber, F., L
¨
ochtefeld, M., Kr
¨
uger, A., McIntosh, J., Mc-
Neill, C., and Fraser, M. (2016). Understanding same-
side interactions with wrist-worn devices. In Pro-
HUCAPP 2020 - 4th International Conference on Human Computer Interaction Theory and Applications
116