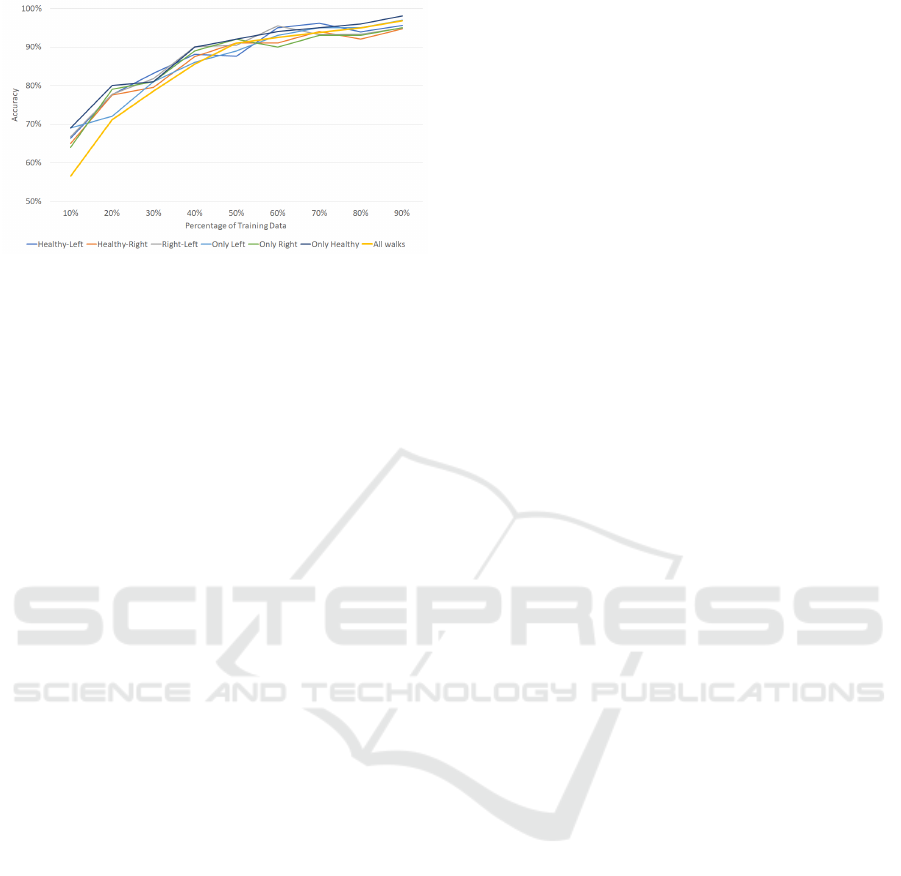
Figure 11: Comparison of classification the walks sepa-
rately.
6 CONCLUSIONS AND FUTURE
WORK
The aim of this paper is to present a system capable of
classifying pathology walks using RNN. The signals
of the lower train are recorded using a commercial
device, that records signals from accelerometer, gyro-
scope, magnetometer and orientation data. The sig-
nals are filtered and processed in order to extract the
information required to feed the neural network. With
the aim to obtain the optimal configuration of the NN
hand-tunning of the hyperparameters has been done,
among them the ratio of training/testing data, number
of neurons per layer and number of layers have been
studied.
To the light of the outcomes, we can see that, in
general cases, the accuracy of the system rises with
the training ratio. There are two cases where it is not
precise. This happens when the complexity of the NN
is too elevate, and the results do not converge at any
point. Adding an extra layer to the NN improves less
than 3% the accuracy of the system, however, it does
not increase significatively the effort.
The experiment of classifying the real cases of
pathology walks give proper results. However, the ex-
periment does not have a significant impact due to the
testing does not hold abundant data. Additionally, it
is able to classify equally the walks from the right and
left and the health walks.
Even though this system works and classifies
properly the walks, there are some improvements that
would be interesting to perform. The first one is to
study the influence of the origin (e.g. the beginning
or the middle of the walk) and the amount of data of
the fragments. In this way, if the amount of data can
be reduced without remarkable changes in the accu-
racy the algorithm can be optimized.
Once a system capable of distinguishing walk
limp and the laterality of them, the next step is to try
the system with real pathologies. So, the next step is
the acquisition of a new database of users with dif-
ferent problems in the lower train. In this case, the
algorithm should be refined to get as a result, not only
the laterality of the limp but also the joint where it
occurs.
ACKNOWLEDGEMENTS
This work was partially supported by the Spanish
National Cybersecurity Institute (INCIBE) under the
Grants Program “Excellence of Advanced Cybersecu-
rity Research Teams.”
The work of this paper has been partly funded by
PREVIEW project, granted by the Spanish Min-
istry of Economy and Competence, with the code
TEC2015-68784-R (MINECO/FEDER)
REFERENCES
Banala SK, Agrawal SK, S. S. (2007). Banala SK, Agrawal
SK, Scholz SP. Active leg exoskeleton (ALEX) for
gait rehabilitation of motor-impaired patients. IEEE
Int Conf Rehabil Robot 2007, 401–7. Rehabilitation
Robotics, . . . , 00(c).
Chollet, F. et al. (2015). Keras. https://keras.io.
Del Din, S., Godfrey, A., Galna, B., Lord, S., and Rochester,
L. (2016). Free-living gait characteristics in ageing
and Parkinson’s disease: Impact of environment and
ambulatory bout length. Journal of NeuroEngineering
and Rehabilitation, 13(1):1–12.
Dickstein, R. (2008). Review article: Rehabilitation of gait
speed after stroke: A critical review of intervention
approaches. Neurorehabilitation and Neural Repair,
22(6):649–660.
Fear, E. C., Li, X., Hagness, S. C., and Stuchly, M. A.
(2002). Confocal microwave imaging for breast can-
cer detection: Localization of tumors in three dimen-
sions. IEEE Transactions on Biomedical Engineering,
49(8):812–822.
Fernandez-Lopez, P., Sanchez-Casanova, J., Liu-Jimenez,
J., and Morcillo-Marin, C. (2017). Influence of walk-
ing in groups in gait recognition. Proceedings - Inter-
national Carnahan Conference on Security Technol-
ogy, 2017-Octob:1–6.
Fernandez-Lopez, P., Sanchez-Casanova, J., Tirado-Martin,
P., and Liu-Jimenez, J. (2018). Optimizing resources
on smartphone gait recognition. IEEE International
Joint Conference on Biometrics, IJCB 2017, 2018-
Janua:1–6.
Go, M., Iacovelli, C., Russo, E., Pournajaf, S., Blasi, C. D.,
and Franceschini, M. (2019). Stroke Gait Rehabilita-
tion : A Comparison of End-E ff ector , Overground
Exoskeleton , and. Applied Sciences.
BIOSIGNALS 2020 - 13th International Conference on Bio-inspired Systems and Signal Processing
66