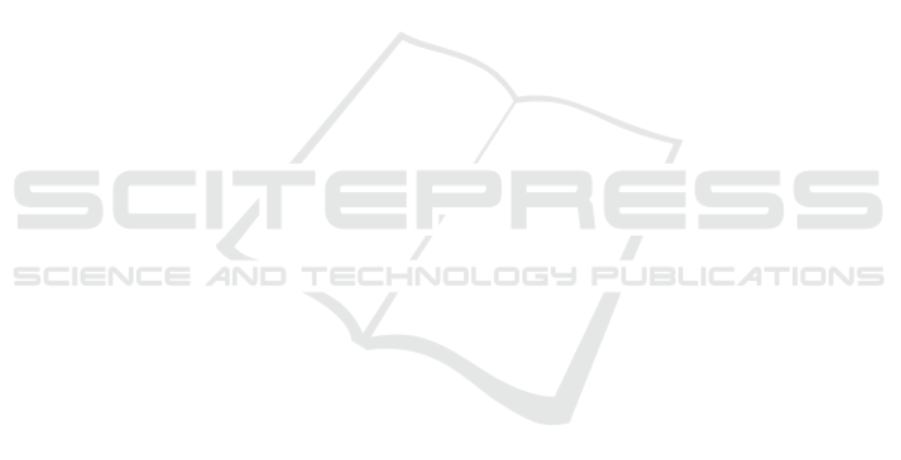
of state-of-art pre-trained natural language process-
ing embedding models based on BERT (Huang et al.,
2019; Alsentzer et al., 2019) for downstream tasks,
which can be used in this context. The explainability
of the model is a crucial issue here as this can be uti-
lized by different stakeholders (Lipton, 2017). In the
future, we will also explore this explainability issue.
5 CONCLUSIONS
We investigated missingness and different time win-
dow sizes in extremely sparse EHR data obtained
from a Swedish university hospital for the task of
early prediction of sepsis using a deep learning-based
LSTM model. It was shown that the size of the win-
dow has a significant impact on the predictive perfor-
mance of the models. We also observed that treating
missing data as missing not at random can in some
cases lead to better predictive performance compared
to assuming that it is missing at random.
REFERENCES
Alsentzer, E., Murphy, J. R., Boag, W., Weng, W.-H.,
Jin, D., Naumann, T., and McDermott, M. (2019).
Publicly available clinical bert embeddings. arXiv
preprint arXiv:1904.03323.
Cassini, A., Plachouras, D., Eckmanns, T., Sin, M. A.,
Blank, H.-P., Ducomble, T., Haller, S., Harder, T.,
Klingeberg, A., Sixtensson, M., et al. (2016). Bur-
den of six healthcare-associated infections on Euro-
pean population health: estimating incidence-based
disability-adjusted life years through a population
prevalence-based modelling study. PLoS medicine,
13(10):e1002150.
Dalianis, H., Henriksson, A., Kvist, M., Velupillai, S., and
Weegar, R. (2015). Health bank-a workbench for data
science applications in healthcare. In CAiSE Industry
Track, pages 1–18.
Delahanty, R. J., Alvarez, J., Flynn, L. M., Sherwin, R. L.,
and Jones, S. S. (2019). Development and Evaluation
of a Machine Learning Model for the Early Identifi-
cation of Patients at Risk for Sepsis. Annals of emer-
gency medicine.
Desautels, T., Calvert, J., Hoffman, J., Jay, M., Kerem, Y.,
Shieh, L., Shimabukuro, D., Chettipally, U., Feldman,
M. D., Barton, C., et al. (2016). Prediction of sepsis in
the intensive care unit with minimal electronic health
record data: a machine learning approach. JMIR med-
ical informatics, 4(3).
Despins, L. A. (2017). Automated detection of sepsis using
electronic medical record data: a systematic review.
Journal for Healthcare Quality, 39(6):322–333.
Ferrer, R., Martin-Loeches, I., Phillips, G., Osborn, T. M.,
Townsend, S., Dellinger, R. P., Artigas, A., Schorr,
C., and Levy, M. M. (2014). Empiric antibiotic treat-
ment reduces mortality in severe sepsis and septic
shock from the first hour: results from a guideline-
based performance improvement program. Critical
care medicine, 42(8):1749–1755.
Futoma, J., Hariharan, S., and Heller, K. (2017a). Learn-
ing to detect sepsis with a multitask Gaussian process
RNN classifier. In Proceedings of the 34th Interna-
tional Conference on Machine Learning-Volume 70,
pages 1174–1182. JMLR.org.
Futoma, J., Hariharan, S., Heller, K., Sendak, M., Bra-
jer, N., Clement, M., Bedoya, A., and O’Brien, C.
(2017b). An improved multi-output gaussian process
rnn with real-time validation for early sepsis detec-
tion. In Doshi-Velez, F., Fackler, J., Kale, D., Ran-
ganath, R., Wallace, B., and Wiens, J., editors, Pro-
ceedings of the 2nd Machine Learning for Health-
care Conference, volume 68 of Proceedings of Ma-
chine Learning Research, pages 243–254, Boston,
Massachusetts. PMLR.
Goodfellow, I., Bengio, Y., and Courville, A. (2016). Deep
learning. MIT press.
Henriksson, A., Zhao, J., Bostr
¨
om, H., and Dalianis, H.
(2015). Modeling heterogeneous clinical sequence
data in semantic space for adverse drug event detec-
tion. In 2015 IEEE International Conference on Data
Science and Advanced Analytics (DSAA), pages 1–8.
IEEE.
Hochreiter, S. and Schmidhuber, J. (1997). Long short-term
memory. Neural computation, 9(8):1735–1780.
Huang, K., Altosaar, J., and Ranganath, R. (2019). Clinical-
bert: Modeling clinical notes and predicting hospital
readmission. arXiv preprint arXiv:1904.05342.
Jones, A. E., Shapiro, N. I., Trzeciak, S., Arnold, R. C.,
Claremont, H. A., Kline, J. A., Investigators, E. M. S.
R. N. E., et al. (2010). Lactate clearance vs central ve-
nous oxygen saturation as goals of early sepsis ther-
apy: a randomized clinical trial. Jama, 303(8):739–
746.
Kumar, A., Roberts, D., Wood, K. E., Light, B., Parrillo,
J. E., Sharma, S., Suppes, R., Feinstein, D., Zanotti,
S., Taiberg, L., et al. (2006). Duration of hypotension
before initiation of effective antimicrobial therapy is
the critical determinant of survival in human septic
shock. Critical care medicine, 34(6):1589–1596.
Li, S. C.-X., Jiang, B., and Marlin, B. (2019). Misgan:
Learning from incomplete data with generative adver-
sarial networks. arXiv preprint arXiv:1902.09599.
Lipton, Z. C. (2017). The doctor just won’t accept that!
arXiv preprint arXiv:1711.08037.
Mani, S., Ozdas, A., Aliferis, C., Varol, H. A., Chen, Q.,
Carnevale, R., Chen, Y., Romano-Keeler, J., Nian, H.,
and Weitkamp, J.-H. (2014). Medical decision sup-
port using machine learning for early detection of late-
onset neonatal sepsis. Journal of the American Medi-
cal Informatics Association, 21(2):326–336.
Moor, M., Horn, M., Rieck, B., Roqueiro, D., and
Borgwardt, K. (2019). Early recognition of sep-
sis with gaussian process temporal convolutional net-
works and dynamic time warping. arXiv preprint
arXiv:1902.01659.
HEALTHINF 2020 - 13th International Conference on Health Informatics
54