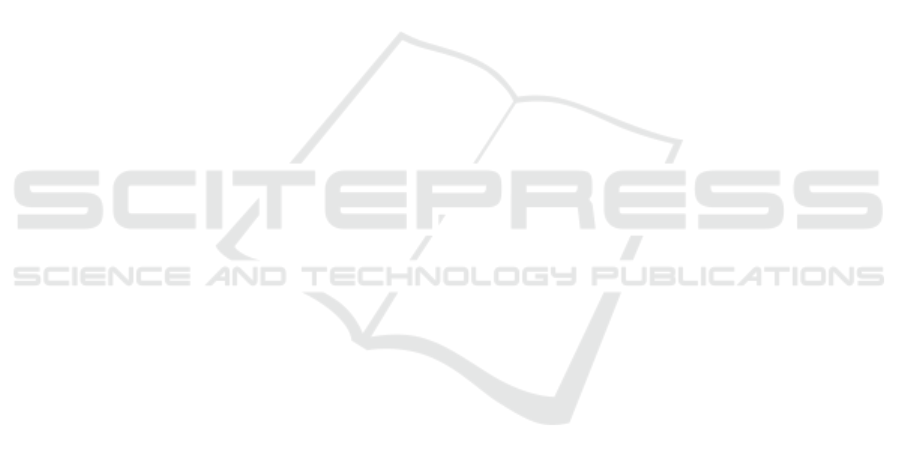
average error (less than 2 m) than the existing method
and baseline.
We investigated how much the error was dis-
tributed for each feature. Figure 9 shows the distri-
butions of the errors of the geo-localization using the
real 360-degree images. In the results of the existing
method (Fig. 9(a)) and baseline (Fig. 9(b)), the peaks
of the distributions have an error of 4 m. In contrast,
in our method (Fig. 9(c)), almost all cases had errors
less than 1 m, though there were a few cases with er-
rors of 5 m. The above results confirm that the pro-
posed PSD features extracted from the ridgeline sig-
nals are effective for geo-localization.
6 CONCLUSIONS
We proposed a method for estimating geo-location us-
ing ridgeline features extracted from 360-degree im-
ages. We evaluated the accuracy of the proposed geo-
localization method using synthesized images gener-
ated from a digital elevation model. We confirmed
that our method substantially outperformed the exist-
ing method. Furthermore, we conducted an experi-
ment to evaluate geo-location using real 360-degree
images collected in the sand dunes. We confirmed
that the average error of our method was less than 2
m.
In future work, we will further evaluate our
method on various datasets of with low texture us-
ing 360-degree cameras. We will also explore the use
of synthesized images to create a target database to
reduce the cost of database generation.
REFERENCES
(Reference 2019-2-12). Digital Map five meter Grid (Ele-
vation), Geospatial Information Authority of Japan.
Baatz, G., Saurer, O., K. K
¨
oser, K., and Pollefeys, M.
(2012). Large scale visual geo-localization of images
in mountainous terrain. In Proceedings of the Euro-
pean Conference on Computer Vision, pages 517–530.
Brejcha, J. and
ˇ
Cad
´
ık, M. (2017). State-of-the-art in visual
geo-localization. Pattern Analysis and Applications,
20(3):613–637.
Chen, X., Hu, W., Zhang, L., Shi, Z., and Li, M. (2018).
Integration of low-cost gnss and monocular cameras
for simultaneous localization and mapping. Sensors
18(7) 2193.
Dusha, D. and Mejias, L. (2012). Error analysis and atti-
tude observability of a monocular gps/visual odome-
try integrated navigation filter. International Journal
of Robotics Research, pages 1–41.
Groves, P. D. (2013). Principles of gnss, inertial, and mul-
tisensor integrated navigation systems. Artech House.
Hofmann-Wellenhof, B., Lichtenegger, H., and Collins, J.
(2001). Global positioning system theory and prac-
tice. Springer-Verlag Wien.
Kim, H. J., Dunn, E., and Frahm, J. (2017). Learned con-
textual feature reweighting for image geo-localization.
In Proceedings of the IEEE Conference on Computer
Vision and Pattern Recognition (CVPR), pages 3251–
3260.
Langley, R. B. (1998). Rtk gps. In GPS WORLD, pages
70–76.
Li, X., Zhang, X., Ren, X., Fritsche, M., Wickert, J.,
and Schuh, H. (2015). Precise positioning with cur-
rent multi-constellation global navigation satellite sys-
tems: Gps, glonass, galileo and beidou. Scientific Re-
ports, 5(8328):1–14.
Lowry, S., Sunderhauf, N., Newman, P., Leonard, J. J.,
Cox, D., Corke, P., and Milford, M. J. (2016). Vi-
sual place recognition: A survey. IEEE Transactions
on Robotics, 32(1):1–19.
Nicolle, L., Bonneton, J., Konik, H., Muselet, D., and
Tougne, L. (2017). Towards an electronic orientation
table: using features extracted from the image to reg-
ister digital elevation model. In Proceedings of the
International Conference on Computer Vision Theory
and Applications, pages 28–38.
Odijk, D., Nadarajah, N., Zaminpardaz, S., and Teunissen,
P. J. G. (2017). Gps, galileo, qzss and irnss differen-
tial isbs: estimation and application. GPS Solutions,
21(2):439–450.
Piasco, N., Sidibe, D., Demonceaux, C., and Gouet-Brunet,
V. (2018). A survey on visual-based localization: On
the benefit of heterogeneous data. Pattern Recogni-
tion, 74:90 – 109.
Porzi, L., Rota, B. S., and Ricci, E. (2016). A deeply-
supervised deconvolutional network for horizon line
detection. In Proceedings of the 24th ACM Interna-
tional Conference on Multimedia, pages 137–141.
Saurer, O., Baatz, G., K
¨
oser, K., Ladick
´
y, L., and Polle-
feys, M. (2016). Image based geo-localization in
the alps. International Journal of Computer Vision,
116(3):213–225.
Geo-localization using Ridgeline Features Extracted from 360-degree Images of Sand Dunes
627