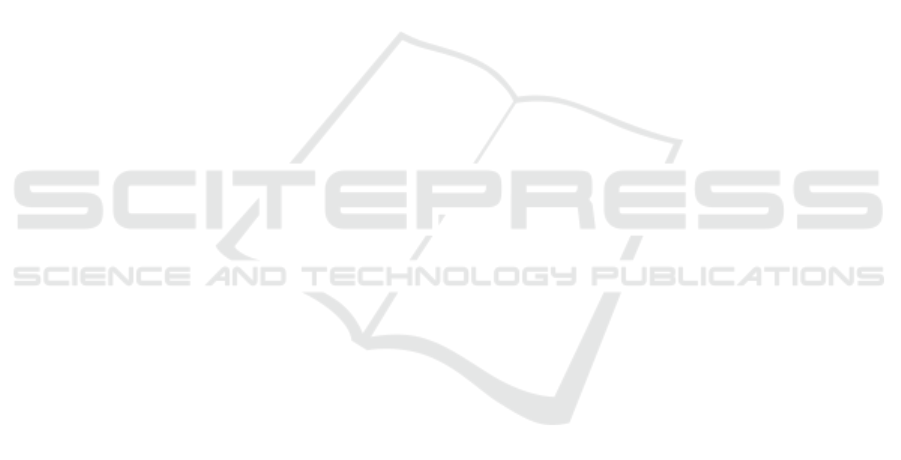
activity, the average accuracies were higher than 75%
for all the classifiers and features analyzed (even re-
moving the epochs’ onset). This suggests a clear dif-
ference between this kind of idle state and color ex-
posure too. Finally, the obtained outcomes confirm
those obtained in (Torres-Garc
´
ıa et al., 2019) using a
different dataset and analyzing a single type of idle
state.
As future work, we will assess the methods in
other related-neuro paradigms. Besides, the outcomes
could be improved for an additional stage for fea-
ture selection or feature reduction such as Principal
Component Analysis (PCA) and the optimization of
the classifiers’ hyperparameters. Also, an assessment
to identify the minimum size of the epochs to dis-
tinguishing between color exposure and idle state is
needed. This would be the second step towards an on-
line color-based BCI implementation. Last, the non-
stationary nature of EEG signals will make necessary
the application of incremental learning in that imple-
mentation, for tuning the method’s hyperparameters
along the time of use of a specific subject.
ACKNOWLEDGEMENTS
We thank the European Research Consortium for In-
formatics and Mathematics (ERCIM) for supporting
this research with a postdoctoral fellowship.
REFERENCES
AlSaleh, M., Moore, R., Christensen, H., and Arvaneh, M.
(2018). Discriminating between imagined speech and
non-speech tasks using eeg. In 2018 40th Annual In-
ternational Conference of the IEEE Engineering in
Medicine and Biology Society (EMBC), pages 1952–
1955. IEEE.
˚
Asly, S., Moctezuma, L. A., Gilde, M., and Molinas, M.
(2019). Towards EEG-based signals classification
of RGB color-based stimuli. In 8th Graz Brain-
Computer Interface Conference, page In press.
Didiot, E., Illina, I., Fohr, D., and Mella, O. (2010).
A wavelet-based parameterization for speech/music
discrimination. Computer Speech & Language,
24(2):341–357.
Dyson, M. (2010). Selecting optimal cognitive tasks for
control of a brain computer interface. PhD thesis, The
University of Essex.
Dyson, M., Sepulveda, F., and Gan, J. Q. (2010). Localisa-
tion of cognitive tasks used in EEG-based BCIs. Clin-
ical Neurophysiology, 121(9):1481–1493.
Liu, X. and Hong, K.-S. (2017). Detection of primary RGB
colors projected on a screen using fNIRS. Journal of
Innovative Optical Health Sciences, 10(03):1750006.
Lotte, F., Bougrain, L., Cichocki, A., Clerc, M., Con-
gedo, M., Rakotomamonjy, A., and Yger, F. (2018).
A review of classification algorithms for EEG-based
brain–computer interfaces: a 10 year update. Journal
of neural engineering, 15(3):031005.
Moctezuma, L. A., Carrillo, M., Villase
˜
nor-Pineda, L., and
Torres-Garc
´
ıa, A. A. (2017). Hacia la clasificaci
´
on de
actividad e inactividad ling
¨
u
´
ıstica a partir de se
˜
nales
de electroencefalogramas (EEG). Research in Com-
puting Science, 140:135–149.
Rasheed, S. (2011). Recognition of primary colours in elec-
troencephalograph signals using support vector ma-
chines. PhD thesis, Universit
`
a degli Studi di Milano.
Rasheed, S. and Marini, D. (2015). Classification of
EEG signals produced by RGB colour stimuli. Jour-
nal of Biomedical Engineering and Medical Imaging,
2(5):56.
˚
Asly, S. (2019). Supervised learning for classification of
EEG signals evoked by visual exposure to RGB col-
ors. Master’s thesis, Norwegian University of Science
and Technology.
Soler-Guevara, A., Giraldo, E., and Molinas, M. (2019).
Partial brain model for real-time classification of RGB
visual stimuli: a brain mapping approach to BCI. In
8th Graz Brain-Computer Interface Conference, page
In press.
Song, Y. and Sepulveda, F. (2014). Classifying speech re-
lated vs. idle state towards onset detection in brain-
computer interfaces overt, inhibited overt, and covert
speech sound production vs. idle state. In 2014 IEEE
Biomedical Circuits and Systems Conference (Bio-
CAS) Proceedings, pages 568–571. IEEE.
Torres-Garc
´
ıa, A. A., Moctezuma, L. A.,
˚
Asly, S., and Moli-
nas, M. (2019). Discriminating between color expo-
sure and idle state using EEG signals for BCI appli-
cation. In International Conference on e-Health and
Bioengineering (EHB) 2019, page In press. IEEE.
Torres-Garc
´
ıa, A. A. and Molinas, M. (2019). Analyz-
ing the Recognition of Color Exposure and Imagined
Color from EEG signals. In International Conference
on BioInformatics and BioEngineering (BIBE) 2019,
page In press. IEEE.
Witten, I. H., Frank, E., Hall, M. A., and Pal, C. J. (2016).
Data Mining: Practical machine learning tools and
techniques. Morgan Kaufmann.
Yu, J.-H. and Sim, K.-B. (2016). Classification of
color imagination using Emotiv EPOC and event-
related potential in electroencephalogram. Optik,
127(20):9711–9718.
BIOSIGNALS 2020 - 13th International Conference on Bio-inspired Systems and Signal Processing
194